Navigating Ethics in Machine Learning
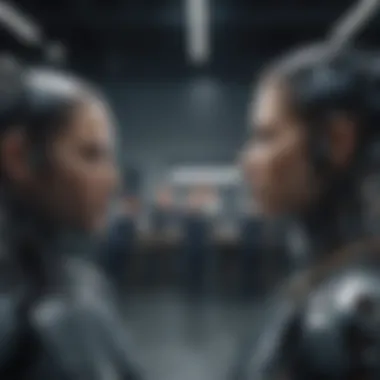
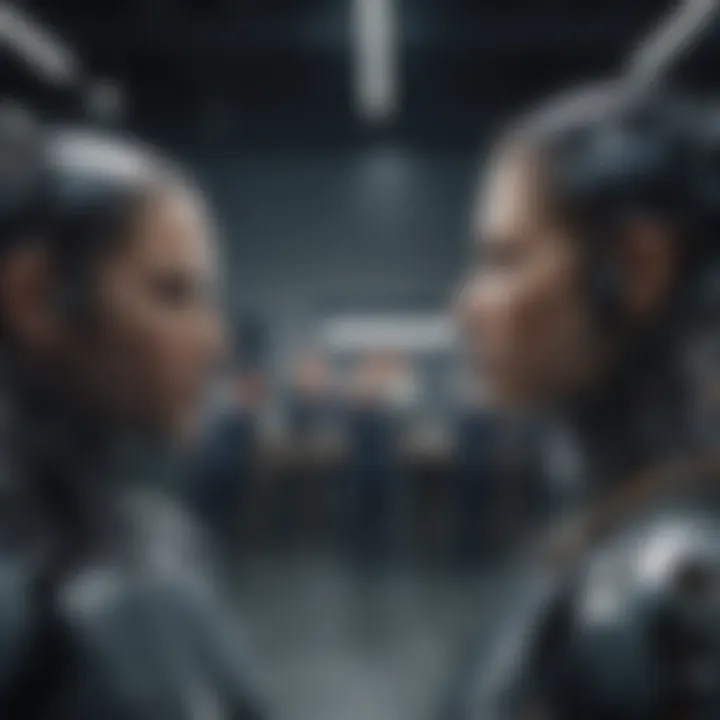
Intro
The rise of machine learning technologies has brought with it a myriad of ethical challenges. Understanding the implications of these challenges is crucial for various stakeholders, including students, researchers, educators, and professionals. Ethics in this context refers not only to the fairness of algorithms but also to the broader societal impacts they can have.
Machine learning systems can influence decision-making in healthcare, finance, hiring, and many other domains. As such, it is relevant to examine their ethical considerations thoroughly.
This article will navigate through important topics such as algorithmic decision-making, data privacy, bias, accountability, and the role of transparency. Each of these aspects plays a significant role in determining how responsibly machine learning can be integrated into society.
By delving into these themes, readers can better appreciate the ethical landscape of machine learning, recognizing the necessity for a conscientious approach to technology development and deployment.
Definition of Ethics in Technology
Ethics in technology refers to the principles that govern the conduct and decision-making processes within technology development and deployment. It aims to ensure that technological advancements contribute positively to society while minimizing harm. In the context of machine learning, ethical considerations include fairness, transparency, accountability, and privacy. These elements shape how algorithms are designed, trained and implemented. They are crucial in addressing potential negative impacts on individuals and communities.
A clear definition of ethics in technology allows stakeholders to align their values with their practices. It encourages critical reflection on the societal implications of machine learning technologies and can serve as a framework for responsible innovation.
The integration of ethics within machine learning holds immense importance for several reasons. Firstly, it protects against biased outcomes that can lead to discrimination. Machine learning models often learn from historical data, which may reflect human prejudices. If these biases remain unchecked, they can propagate unequal treatment across various groups, exacerbating social inequalities. Ensuring ethical practices helps mitigate these risks.
Secondly, ethical considerations promote transparency in algorithmic decision-making. Users are increasingly concerned about how decisions affecting their lives are made. By making machine learning processes more transparent, organizations can foster trust with their stakeholders. Clear communication regarding how algorithms function can reduce fears related to randomness and lack of oversight.
Lastly, a robust ethical framework is necessary for building a sustainable future in technology. As machine learning continues to evolve, its relationship with societal norms and values will also change. By embedding ethical considerations into its core, the technology can adapt to meet emerging societal expectations.
"Navigating the ethical landscape of machine learning is not only a moral obligation, but also a strategic necessity for sustaining trust and integrity in technological advancements."
Historical Context of Machine Learning Ethics
Understanding the historical context of machine learning ethics is essential for grasping the complexities and challenges faced today. The evolution of ethical considerations parallels advancements in machine learning technologies. This section will illuminate how previous developments have paved the way for current ethical dilemmas. It sets the groundwork for dissecting ongoing challenges within the field, emphasizing the need for ethical frameworks in guiding future innovations.
Evolution of Machine Learning Technologies
Machine learning has undergone significant transformations since its inception. Early algorithms primarily utilized simple statistical methods. These foundational systems relied on large datasets, but they often lacked the capability to generalize from limited information. As computational power increased, so did the complexity of the algorithms. With innovations such as neural networks and deep learning, machine learning models became capable of performing more intricate tasks, like image recognition and natural language processing.
Emergence of Ethical Concerns
As machine learning systems became more prevalent, ethical concerns about their implications intensified. The realization that algorithms could reflect and amplify social biases led to a critical examination of their outputs. For instance, facial recognition technology has been criticized for its effectiveness based on racial and gender disparities. Algorithms employed in hiring processes were found to favor certain demographics over others, creating a cycle of discrimination.
In light of these issues, several academic institutions and think tanks began to address the ethical dilemmas posed by machine learning. Discussions around transparency, accountability, and fairness shifted from theoretical considerations to urgent practical needs.
"The use of machine learning in decision-making processes must be scrutinized. Ethical considerations are no longer an afterthought; they are integral to the development of responsible technology."
There is now an active discourse among researchers aimed at developing frameworks that inherently incorporate ethical principles into machine learning practices. The establishment of industry standards and guidelines aims to mitigate detrimental impacts while promoting benefits arising from these technologies.
In summary, the historical context of machine learning ethics provides vital insights into the challenges we face today. It highlights the evolution of technology and the corresponding rise of ethical concerns. Recognizing the dynamics of this relationship is crucial for navigating future developments in machine learning.
Core Ethical Principles
Understanding and implementing core ethical principles is vital in the realm of machine learning. These principles help uphold standards that ensure technology serves society positively. The discussion of fairness, transparency, accountability, and privacy reflects on how machine learning systems interact with users and society as a whole. These concepts guide developers and stakeholders in creating systems that not only function efficiently but also align with ethical norms.
Fairness
Definition and Importance
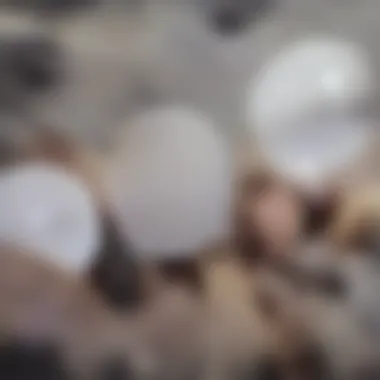
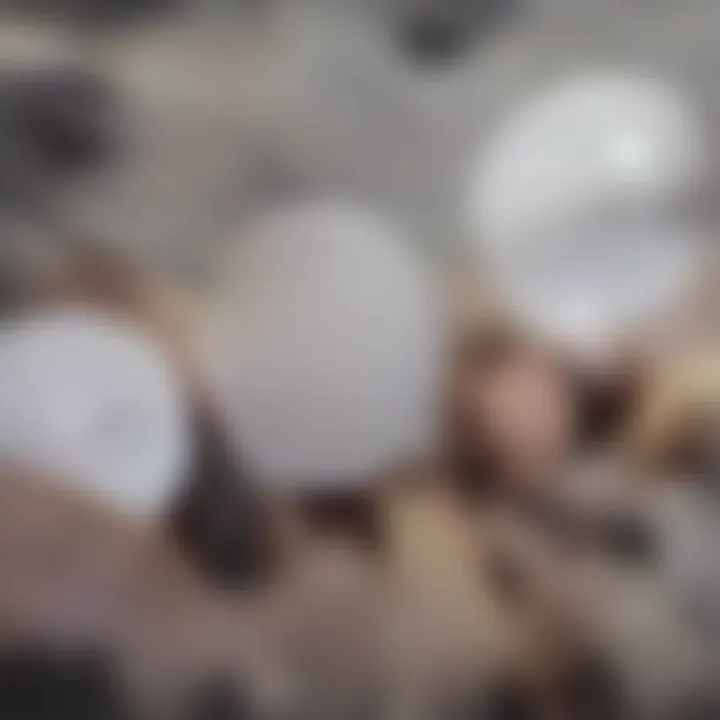
Fairness in machine learning refers to the idea of impartiality in algorithmic decisions. It aims to ensure that all individuals receive equal treatment, regardless of race, gender, or background. Incorporating fairness into machine learning is crucial because it helps prevent discrimination. For instance, an AI model used in hiring should not favor one demographic over another. This approach promotes workplace diversity and inclusion. Fairness is also a popular choice in discussions about ethics because it resonates with a wide audience concerned about social justice.
Addressing Algorithmic Bias
Addressing algorithmic bias is about recognizing and correcting biases within machine learning models. This carries substantial implications for ethical machine learning. If left unchecked, biases may lead to unfair outcomes, harming individuals and communities. Techniques to mitigate bias include better data representation and algorithm adjustments. Notably, addressing bias improves the credibility of AI systems. However, it requires ongoing efforts as biases may shift over time or differ across contexts, presenting both challenges and responsibilities for developers.
Transparency
Understanding Algorithmic Decision-Making
Understanding algorithmic decision-making is essential for users and stakeholders to trust machine learning systems. It involves making the decision-making process clear and accessible. Offering insight into how algorithms work demystifies AI technologies. This transparency cultivates a relationship of trust between users and technology providers. A transparent approach can significantly enhance user confidence in AI applications, which is a core objective in ethical discussions.
Communicating AI Operations
Communicating AI operations includes articulating how machine learning models function and how they are used. It involves providing clear documentation and explanations to users. Effective communication reduces misunderstandings about AI capabilities and limitations. It is beneficial for fostering informed user engagement with AI systems. However, the challenge lies in simplifying complex processes without losing essential details, which is crucial for user comprehension.
Accountability
Assigning Responsibility
Assigning responsibility in the context of machine learning is about determining who is liable for the outcomes produced by algorithms. This principle is essential for establishing trust and responsibility in AI deployments. Often, it complicates relationships between developers, users, and organizations. Clarifying accountability helps in addressing ethical breaches or operational mishaps. A clear responsibility framework can protect companies from legal issues while also ensuring users feel secure.
Impact on Users and Society
The impact of machine learning on users and society is profound. Every decision made by an algorithm influences lives, particularly when deployed at scale. Accountability ensures that there is a mechanism to address adverse effects on individuals and groups. The continuous monitoring of AI impacts allows for swift corrections if harm occurs. However, assessing impacts can be challenging due to the complexity and unpredictability of AI behavior in real-world settings.
Privacy
Data Governance and Protection
Data governance and protection is about managing and safeguarding user data effectively. As machine learning systems rely heavily on data, ethical handling becomes critical. Data governance frameworks are beneficial in establishing guidelines for data usage and ensuring compliance with laws like GDPR. By prioritizing data protection, companies build user trust and avoid potential legal consequences. However, managing privacy can often conflict with the data needs of model performance.
Implications of Data Misuse
Implications of data misuse can be severe, impacting individuals and broader society. Misuse may lead to breaches of personal information, identity theft, and loss of trust in technology. Understanding these implications is crucial for developers and organizations. It emphasizes the need for robust data protections and ethical practices. However, addressing data misuse requires a balance between innovation and privacy, which often poses difficulties for stakeholders.
Bias in Machine Learning
Understanding bias in machine learning is crucial. Bias affects the fairness and accuracy of algorithms, influencing their decision-making processes. This section will cover the types of bias, how to identify them, and strategies for mitigation. The effects of bias are significant as they may lead to systemic issues, reinforcing inequalities or stereotypes built into the data. By acknowledging and addressing these biases, professionals can advance the ethical landscape of machine learning.
Types of Bias
Sample Bias
Sample bias arises when the data collected does not represent the intended population. This can happen if certain groups are overrepresented or underrepresented in the data set. A key characteristic of sample bias is its potential to skew algorithm outcomes, making them less accurate for certain demographics. It's relevant for this article as it illustrates how poorly assembled data can mislead predictions. A unique feature of sample bias is its contribution to reinforcing existing societal biases. The disadvantages of sample bias include a lack of generalizability and potential harm to marginalized groups.
Prejudice Bias
Prejudice bias stems from existing societal stereotypes, often found in historical data. This bias reflects biases that are culturally or socially constructed. A significant characteristic of prejudice bias is that it can perpetuate discrimination in algorithmic decisions. The inclusion of this topic in our article is important because it reveals how biases in human judgment can influence AI systems. The uniqueness of prejudice bias lies in its ability to embed cultural biases into algorithms. The advantages include raising awareness about these issues, but the major disadvantages include the risk of reinforcing stereotypes and creating adverse outcomes for affected groups.
Measurement Bias
Measurement bias occurs when the tools used to collect data are flawed or miscalibrated. A key trait of measurement bias is its influence on the accuracy of data inputs for machine learning algorithms. This section contributes to the overall topic by highlighting the pitfalls of relying on inaccurate measurements. Its unique feature is the focus on the processes of data collection and how they impact outcomes. While measurement bias can lead to insights into flaws in the data pipeline, its disadvantages include producing unreliable results that impact decision-making negatively.
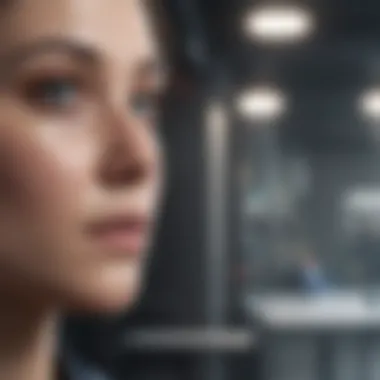
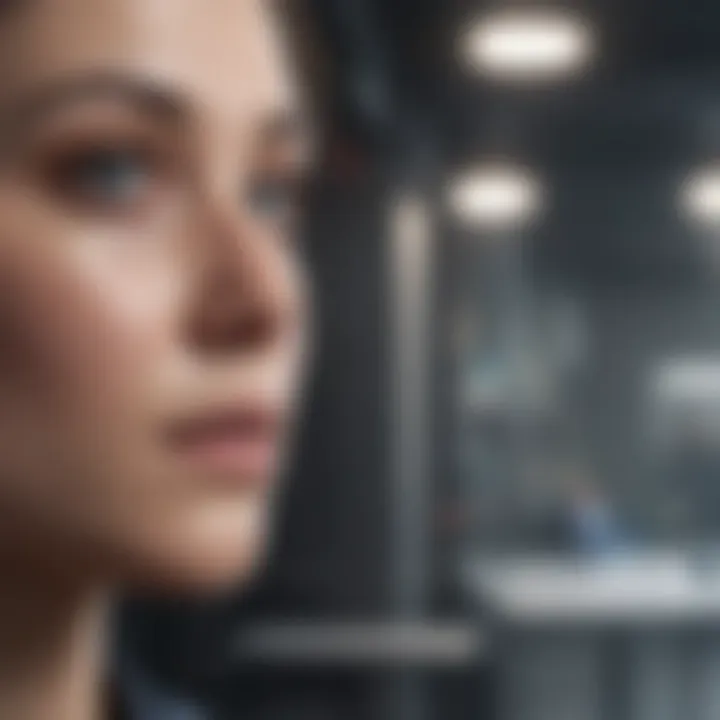
Identifying and Mitigating Bias
Techniques for Detection
Detection techniques help identify biases present in datasets and algorithms. These approaches often involve statistical analysis and visualization techniques. A primary characteristic of these techniques is their role in fostering a clearer understanding of how biases manifest. Utilizing various detection techniques is essential for our discussion as they provide a roadmap for recognizing bias. A notable unique feature of these techniques is their ability to offer actionable insights into the dataset’s composition. Their advantage lies in early identification of issues, while a disadvantage might include the complexity of implementation.
Strategies for Mitigation
Mitigation strategies are essential for addressing identified biases effectively. This includes practices such as diversifying training data, adjusting algorithms, or implementing fairness constraints. A core characteristic of these strategies is their proactive nature. Discussing these strategies is beneficial for providing a pathway toward ethical machine learning. The unique feature of mitigation strategies is in their ability to change the decision-making landscape of algorithms. While these strategies have advantages like promoting fairness, they can also pose challenges in balancing performance with ethical considerations.
Ethics Frameworks and Guidelines
Ethics frameworks and guidelines play a crucial role in shaping the landscape of machine learning technologies. They provide foundational principles that help organizations navigate the complex ethical dilemmas that arise in this field. These frameworks not only foster trust among users but also promote fairness, accountability, and transparency in AI systems. By aligning technological advancements with ethical standards, these frameworks aim to minimize harm and maximize benefits to society.
International Guidelines
OECD Principles
The OECD Principles are significant to the discussion of machine learning ethics because they establish a cohesive international standard for AI. They center on key values such as transparency, integrity, and accountability in the development and application of AI technologies. One important characteristic of these principles is their global applicability; they are designed to influence policymakers, industry leaders, and tech developers alike, making them a beneficial choice for this article.
A unique feature of the OECD Principles is their focus on promoting responsible AI. They urge stakeholders to consider the societal impacts of AI systems, thus emphasizing ethical engagement at every stage of machine learning development. However, a potential disadvantage is that these principles may lack specific enforcement mechanisms, which raises questions about their overall effectiveness in achieving compliance.
EU High-Level Expert Group
The EU High-Level Expert Group on Artificial Intelligence contributes significantly to the ethical framework discourse by providing guidelines that stress human-centric AI. A key characteristic of this group's work is the commitment to ensuring that AI systems respect human rights and democratic values. This aspect makes it a relevant choice for this article, as it addresses the pressing need for ethical orientation in AI deployments.
One unique feature of the EU Expert Group's recommendations is their emphasis on stakeholder involvement, which encourages diverse perspectives in the ethical discussion of AI. This inclusivity can be seen as an advantage, as it allows for a broad range of insights that enhance the robustness of ethical guidelines. However, the challenge lies in the need for compatible implementation across member states, which can introduce variances in compliance levels.
Corporate Governance and Responsibility
Establishing Ethical Standards
Establishing ethical standards is critical for organizations working in machine learning. It serves as a framework for decision-making processes that are aligned with ethical considerations. One defining characteristic of this aspect is that it sets a clear code of conduct for organizations, making it a beneficial inclusion in this article.
The unique feature of establishing ethical standards is the potential for organizations to create accountability structures. These can ensure that AI deployments consider societal impacts, aiding in responsible innovation. However, one disadvantage is the challenge of ensuring these standards are followed consistently across diverse teams and business units.
Case Studies of Implementation
The unique feature of these case studies is their situational specificity; they capture the context in which ethical standards were applied or neglected. This specificity can reveal advantages, such as improved stakeholder trust in companies that prioritize ethics, but can also highlight disadvantages, such as the risk of negative publicity for organizations that fail to adhere to established guidelines.
Consequences of Ethical Lapses
Ethical lapses in machine learning often result in significant real-world consequences. Understanding these consequences is not only essential for developers but also for policymakers, businesses, and society as a whole. The fallout from neglecting ethical standards can manifest in various forms, ranging from legal ramifications to the loss of public trust. Addressing these aspects is essential to maintain the integrity of machine learning applications and ensure they serve society positively.
Real-World Implications
Discrimination in Applications
Discrimination in applications can lead to biased results against certain groups. For instance, algorithms used in hiring processes may unintentionally favor one demographic over others based on historical data. This reinforces existing societal biases and can result in unjust treatment of individuals. Discrimination in applications is particularly concerning because it disrupts fairness in critical settings like employment, lending, and law enforcement. Its identification and mitigation are vital for creating equitable AI systems.
Public Trust and Reputation Damage
Public trust is imperative for the acceptance of machine learning technologies. When ethical lapses occur, trust diminishes significantly. For example, technology companies that misuse user data or deploy biased algorithms face reputational harm. This represents a critical aspect of ethical responsibility. If the public cannot trust a system, its potential benefits are severely undermined. Trust is not easily rebuilt, and damage to reputation can lead organizations to lose customers and market share.
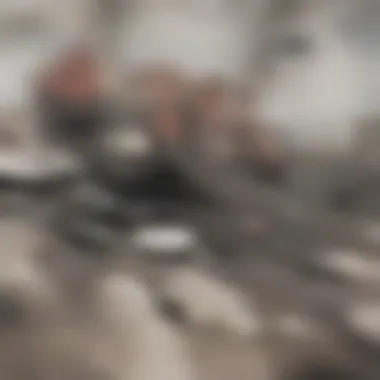
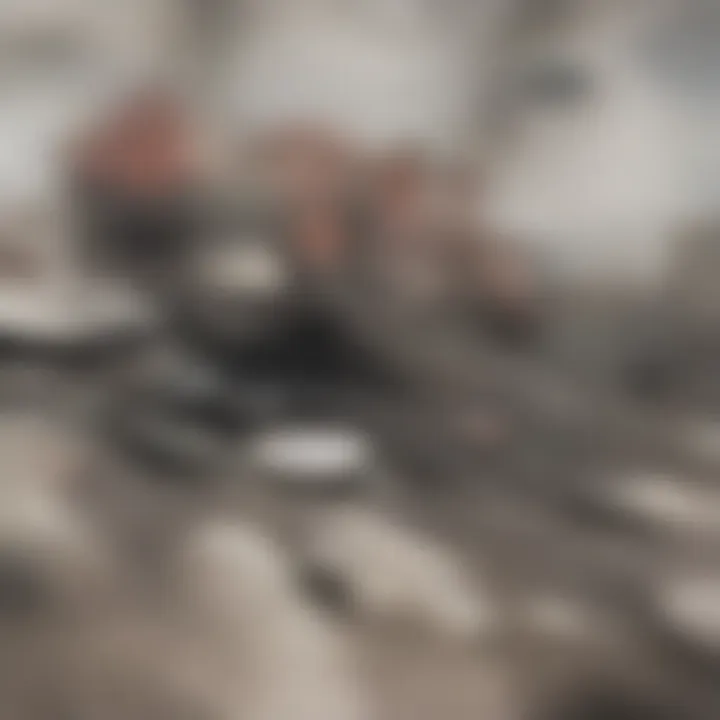
Legal and Regulatory Ramifications
Emerging Regulations
Emerging regulations can shape the future of ethical machine learning practices. Governments worldwide are beginning to create frameworks to govern AI technologies to protect consumers. Regulations such as the General Data Protection Regulation in Europe have set high standards for data protection. This affects how companies design and implement their machine learning models. Such regulations encourage firms to prioritize ethics, thus advancing the responsible deployment of technology.
Litigation Cases
Litigation cases stemming from ethical violations can have far-reaching effects on the companies involved. Recent examples, such as cases related to algorithmic discrimination, spotlight the legal risks organizations face. These cases emphasize the need for careful compliance with ethical guidelines in technology development. Companies may incur significant financial penalties or be forced to alter their practices drastically, impacting innovation in the tech sector.
"Ethical lapses in machine learning not only damage reputations but also pose serious legal challenges that can lead to regulatory changes."
Future Directions in Ethical Machine Learning
The field of machine learning is rapidly advancing, bringing new opportunities and challenges. Future directions in ethical machine learning are critical for ensuring responsible development and deployment of these technologies. Innovations in technology necessitate a closer look at ethical frameworks to guarantee protection for users and society. There is a clear need to align ethical standards with evolving practices. This alignment will facilitate trust in machine learning systems, allowing for widespread acceptance and integration into various fields.
Technological Innovations and Ethics
The Role of Explainability
Explainability is an essential aspect of machine learning. It refers to how well the internal workings of an algorithm can be understood by humans. The transparency of machine learning models grants stakeholders insights into decision-making processes. This characteristic is vital to trust and accountability in AI systems. The explainability is beneficial, especially in high-stakes scenarios like healthcare and criminal justice. A clear understanding of algorithmic decision-making reduces skepticism and promotes acceptance among users.
However, some complex models, such as deep neural networks, pose challenges regarding explainability. These models often operate as "black boxes," making them difficult to interpret. This lack of clarity can create concerns about accountability. Thus, researchers are increasingly focusing on ways to enhance explainability. Achieving a balance between performance and interpretability is crucial.
Explainable AI (XAI)
Explainable AI, or XAI, aims to produce models that are more transparent and understandable. This initiative contributes to mitigating ethical concerns associated with machine learning. The key feature of XAI is its ability to clarify the reasoning behind AI decisions. This characteristic is vital for regulatory compliance and ethical standards. XAI allows stakeholders to challenge or question AI decisions, fostering a sense of agency among users.
One unique feature of XAI is its various techniques, like feature importance and decision trees. These methods translate complex information into simpler concepts. By simplifying the output of AI, stakeholders can better evaluate the fairness and accuracy of decisions. However, developing XAI systems can come with trade-offs, such as reduced overall model accuracy. Therefore, it is a continuous balancing act between interpretability and model performance.
Changing Regulatory Landscapes
Global Perspectives
Global perspectives on machine learning ethics encompass a variety of approaches. Countries and organizations worldwide are recognizing the significance of establishing ethical guidelines. By assessing diverse frameworks, stakeholders can comprehend the implications of machine learning on a larger scale. The cooperation between nations is vital for setting universal standards for responsible AI use.
One important characteristic of global perspectives is the acknowledgment that ethical challenges are not confined within national borders. This understanding encourages collaboration across jurisdictions. However, the challenge lies in reconciling differing cultural values. Each country's ethical framework reflects its unique societal norms. Consequently, this variability can create inconsistencies in the application of machine learning ethics.
National Initiatives
National initiatives play a critical role in shaping machine learning ethics. Many governments are developing strategies to address ethical considerations explicitly. These initiatives contribute to the overall regulation of AI applications, enhancing safety and accountability.
One key feature of national initiatives is their alignment with local laws and cultural contexts. They cater to specific societal needs, making them more relatable and effective. Such initiatives emphasize the importance of stakeholder engagement in formulating policies. However, the disadvantage of national approaches could lead to fragmentation in regulation. Each nation may adopt different standards, complicating compliance for international companies.
Closure
Summary of Key Points
This article provided a thorough examination of several key themes surrounding machine learning ethics. We discussed the definition and significance of ethics in technology, revealing how it shapes algorithmic decision-making processes. We identified various forms of bias in machine learning and the necessity for accountability and transparency in AI systems. The frameworks and guidelines highlighted also stress the importance of establishing ethical standards within corporate governance.
Effective ethical practices not only enhance trust but also safeguard against potential legalities arising from algorithmic discrimination.
Call for Ongoing Ethical Vigilance
As we advance into the future of machine learning, a call for ongoing ethical vigilance is essential. Stakeholders must ensure that ethical considerations remain at the forefront of technological advancements. Continuous education on bias and algorithm ethics is necessary for users, developers, and researchers alike. Regularly contrasting new developments against ethical benchmarks fosters an environment of responsibility.
Maintaining an ethical mindset will not only improve the credibility of AI systems but will also contribute to shaping policies that govern their use, ultimately benefiting society as a whole.
In summary, as machine learning technology evolves, so too must our approaches to ethics. Awareness and proactive engagement with ethical issues can lead to more robust, fair, and transparent systems.