Exploring Peptide Structure Generators in Biochemistry

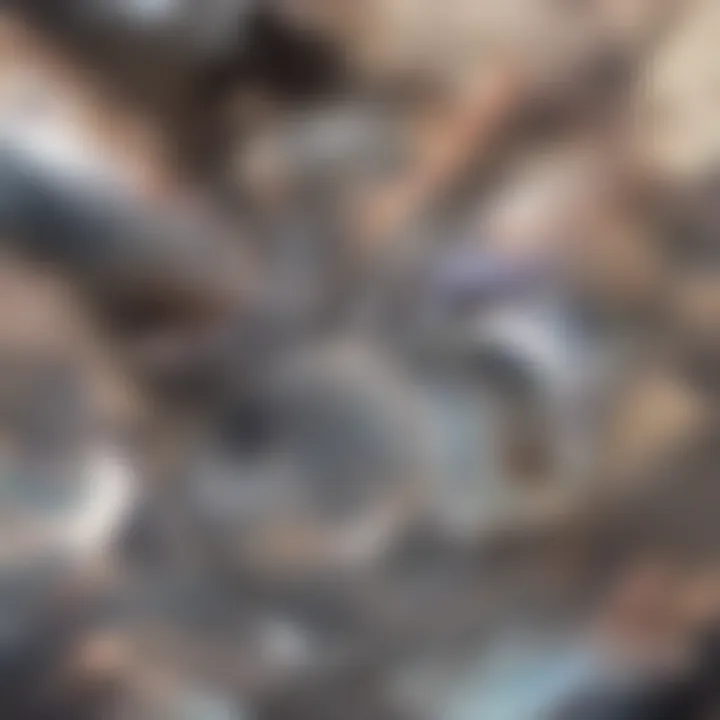
Intro
Peptide structure generators play a crucial role in modern biochemistry. Their ability to synthesize and analyze peptides is essential for various applications, including drug design and therapeutic strategies. This article aims to provide a thorough understanding of these generators by discussing their mechanisms, applications, and the challenges they face. This overview will set the stage for a deeper exploration of the subject.
Research Context
Background and Rationale
Peptide synthesis has been a cornerstone in biochemistry, with implications ranging from basic research to therapeutic development. As interest in biopharmaceuticals grows, the design of peptides becomes increasingly important. Peptide structure generators facilitate this process, allowing scientists to create peptide sequences rapidly and efficiently. Traditional methods of peptide synthesis can be time-consuming and resource-intensive. In contrast, structure generators leverage computational power, enhancing accuracy and enabling the exploration of novel sequences.
Literature Review
Research on peptide structure generation has evolved significantly over the past few decades. Early studies focused primarily on developing automated synthesis techniques. Lately, there is a shift towards integrating computational methods to complement experimental approaches. For instance, the work by Kuhlman and coworkers (2003) illustrates the advancements in computational peptide design. Their findings emphasize the potential of algorithms to accurately predict structural conformations, aiding in the synthesis of novel peptides with desired properties.
Current literature continues to explore improvements in structural predictions and align these tools with experimental validation. A review published by Maiti et al. (2021) highlights recent software tools and their applications in peptide generation. Furthermore, the challenges of structural alignment and accuracy remain a key focus, as errors in peptide design can have significant consequences in therapeutic applications.
Methodology
Research Design
A mixed-method approach is beneficial in studying peptide structure generators. This method combines both qualitative and quantitative analysis to propose solutions to existing challenges. By examining software capabilities alongside experimental outcomes, researchers can identify best practices in peptide synthesis.
Data Collection Methods
- Software performance metrics: Evaluating accuracy and time efficiency of various structure generators.
- Experimental results: Comparing predicted vs. actual peptide structures.
- Surveys and expert interviews: Gathering insight from leading biochemists about the advantages and limitations of current tools.
Such comprehensive data collection can provide nuanced perspectives about the efficacy and practical applications of peptide structure generators.
Preamble to Peptide Structure Generators
Peptide structure generators play a critical role in biochemistry. They are essential tools in understanding how peptides fold and function. Peptides are short chains of amino acids, and their specific structures dictate their biological activities. By using these generators, researchers can predict and analyze the three-dimensional configurations of peptides, which is vital for drug design and other applications. The benefits of understanding peptide structures include enhanced drug efficacy and improved therapeutic outcomes. This introduction sets the stage for a deep dive into the mechanisms, significance, and challenges associated with peptide structure generators.
Definition and Purpose
A peptide structure generator refers to the methodologies and tools used to create models of peptide structures. They simulate how peptides fold into their functional forms. The main purpose of these generators is to facilitate the understanding of peptide behavior and interactions at the molecular level. This understanding is crucial for various scientific fields, including pharmaceuticals, where accurate modeling can lead to the development of new drugs. Furthermore, these generators can assist in predicting how modifications to peptide sequences may alter their structure and function.
Historical Context
The journey of peptide structure generation has evolved significantly over the decades. In the early stages, methods such as manual modeling and simple physical simulations dominated the scene. As technology advanced, computational tools gained prominence, revolutionizing this field. The advent of techniques like molecular dynamics simulations allowed for a more comprehensive understanding of peptide structures. Historical milestones, such as the development of the first protein models in the 1950s, laid the groundwork for today’s sophisticated generators. This evolution reflects broader trends in biochemistry, where increasing computational power has enhanced research capabilities.
Significance in Modern Biochemistry
In contemporary biochemistry, peptide structure generators are of monumental importance. They serve as a bridge between sequence information and structural understanding. With the rise of personalized medicine, these tools enable researchers to tailor therapies to individual genetic profiles. Additionally, peptide generators aid in vaccine design by predicting how peptides will present antigens to immune cells. Their significance extends to biomarker discovery, where accurate structural predictions can enhance the identification of disease-related peptides. Thus, the role of peptide structure generators is integral to advancing biochemistry and improving human health.
"Understanding structure is essential for discovering how peptides can be used in various therapeutic applications."
The integration of these tools into research methodologies has transformed how scientists approach problems in biochemistry, solidifying their place as indispensable resources.
Mechanisms of Peptide Structure Generation
The mechanisms of peptide structure generation are crucial for understanding how peptides are created and analyzed. This foundation supports several applications, such as drug development and vaccine design. By exploring molecular composition, synthesis techniques, and methods for structural analysis, researchers can leverage peptide generators to improve outcomes in biochemistry.
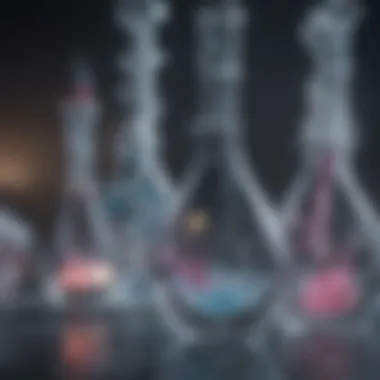
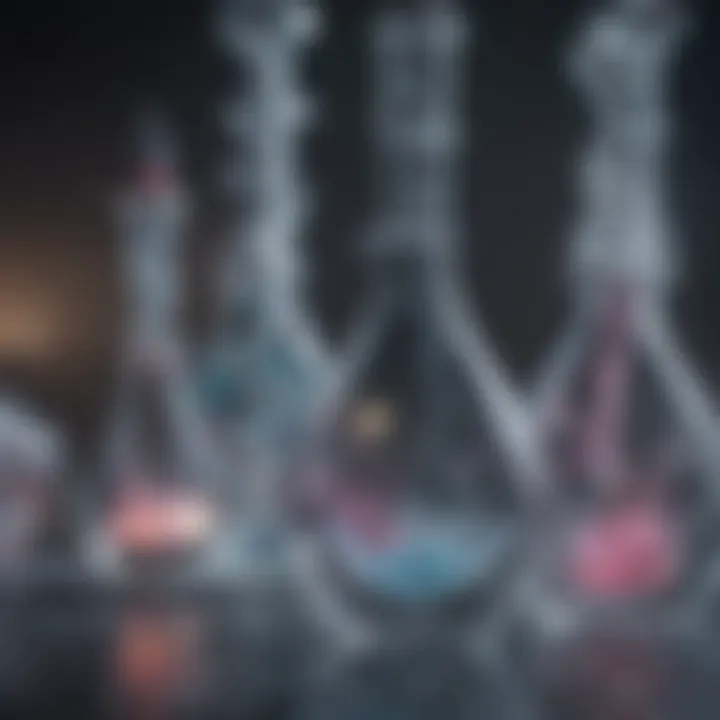
Chemical Composition of Peptides
Peptides are short chains of amino acids linked by peptide bonds. Their structure is determined by the sequence of these amino acids. Each amino acid has a unique side chain that influences the peptide's properties. Factors like polarity and charge play important roles in folding behaviors and stability. Understanding this composition is essential for effective peptide design, enabling scientists to optimize for desired functions.
Synthesis Techniques
Synthesis techniques for peptides have evolved significantly, allowing for rapid production and greater precision. Two primary methods are solid-phase synthesis and liquid-phase synthesis. Each method has its own merits and is chosen based on specific needs of the project.
Solid-Phase Synthesis
Solid-phase synthesis involves attaching the first amino acid to a solid support, thus allowing a stepwise addition of further amino acids. A key characteristic of this method is its efficiency in purifying the product. This purification process occurs after each addition, reducing impurities and yielding high-purity peptides. As a beneficial choice for synthesizing complex peptides, solid-phase synthesis is widely accepted. One of its unique features is the ability to easily automate the synthesis process, leading to reproducible results. However, a disadvantage can be its complexity with longer sequences, as this might lead to cumulative errors during synthesis.
Liquid-Phase Synthesis
Liquid-phase synthesis is another method that can be used for generating peptides. In this technique, the amino acids are dissolved in a solution, allowing for a more flexible reaction environment. A significant aspect of liquid-phase synthesis is that it can accommodate larger peptides than solid-phase methods. This makes it a popular choice in certain applications. One unique feature is that reaction conditions can be easily varied by adjusting solvent types and concentrations. Though advantageous, liquid-phase synthesis often results in lower purity, which may complicate downstream applications.
Techniques in Structural Analysis
Analyzing the structure of synthesized peptides is as important as their synthesis. Two commonly used techniques in structural analysis are NMR spectroscopy and X-ray crystallography. These techniques offer insights into the three-dimensional structures of peptides, providing vital information for further applications.
NMR Spectroscopy
Nuclear Magnetic Resonance (NMR) spectroscopy is an effective technique used to determine the structure of peptides in solution. A key characteristic of NMR is its ability to provide information about the dynamics of peptides in their natural environment. This quality makes NMR an advantageous choice among structural analysis techniques. Unlike other methods, NMR can give insight into conformational changes and interactions. The challenging aspect of NMR is that it requires high concentrations of peptide, which may not always be feasible.
X-ray Crystallography
X-ray crystallography remains a cornerstone method in elucidating peptide structures. This technique involves crystallizing peptides and then analyzing the diffraction pattern produced when X-rays interact with the crystal. A defining feature of X-ray crystallography is its high-resolution output, which allows researchers to visualize precise atomic arrangements. This makes it a favored choice for structural studies. However, the main limitation involves the difficulty of obtaining suitable crystals, as not all peptides can be crystallized easily.
"Understanding mechanisms involved in peptide synthesis and analysis reveals the intricacies of designing functional peptides for therapeutic use."
Computational Tools for Peptide Structure Generation
Computational tools play a crucial role in peptide structure generation. These tools streamline the design and analysis of peptides. They enhance our understanding of peptide behavior in a three-dimensional space. The incorporation of computational methods allows researchers to predict and visualize structures before laboratory synthesis. This efficiency saves time and resources, crucial in research and medical applications.
Overview of Computational Methods
Computational methods vary widely but share a common goal: generating accurate models of peptide structures. Among the most significant methods, molecular modeling and simulation techniques feature prominently. They provide insights into molecular interactions and stability. Some notable computational techniques include:
- Force Field Calculations: These provide energy approximations for stability and can predict conformational changes.
- Quantum Mechanical Methods: Useful for precise electronic structure information, helping to understand molecular properties at a fundamental level.
- Ab Initio and Density Functional Theory (DFT): These methods offer highly accurate details but require intensive computational resources.
"The power of computational tools lies in their ability to transform theoretical predictions into actionable insights."
Each of these methods has specific applications depending on the required accuracy and the nature of the peptide being studied. Their combined use can result in a comprehensive understanding of peptide behavior.
Molecular Dynamics Simulations
Molecular dynamics (MD) simulations offer a detailed approach to studying peptide structures. They allow researchers to observe the physical movements of atoms over time under specific conditions. This method provides valuable insights into:
- Conformational Changes: Observing how a peptide might fold or change shape in real time.
- Interaction with Other Molecules: Understanding how peptides interact with ligands, proteins, or membranes, which is vital in drug design.
- Thermodynamics: Providing information on stability and behavior under various environmental conditions.
MD simulations are particularly powerful because they simulate realistic biological environments. The application of these simulations is extensive, impacting fields such as biochemistry, pharmacology, and molecular biology.
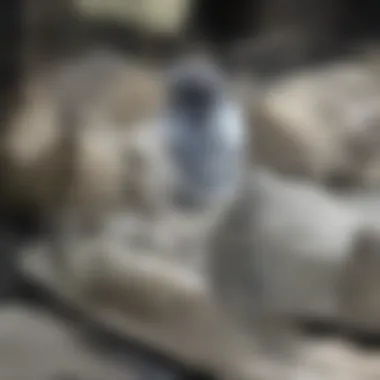
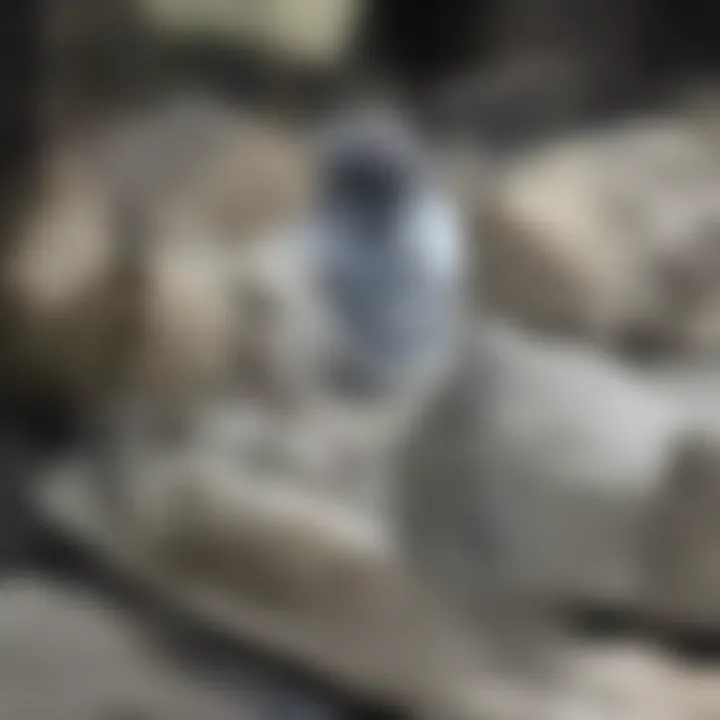
Machine Learning in Peptide Design
Machine learning (ML) has emerged as a revolutionary method in peptide structure generation. This approach leverages algorithms to predict outcomes based on large data sets. Its applications in peptide design provide:
- Pattern Recognition: Identifying patterns from existing peptide data, enabling predictions about new designs.
- Optimization Tools: Assisting in refining peptide sequences to enhance their stability and efficacy.
- Automating Design Processes: Reducing human error and increasing efficiency in generating peptide libraries.
Incorporating ML into peptide design not only accelerates the process but also opens new pathways for innovative therapeutic solutions. ML algorithms can analyze vast amounts of data, uncovering insights that might be missed using traditional methods.
As computational tools continue to evolve, they are shaping the future of peptide structure generation. Their integration into research underscores the importance of innovation and adaptability in biochemistry.
Applications of Peptide Structure Generators
Peptide structure generators play a crucial role in biochemistry by offering innovative methods for the development and design of peptides. Understanding their applications is vital, as they encompass various domains within medical science, particularly in drug development, vaccine design, and biomarker discovery. Peptides can be designed and synthesized to achieve specific functions, making them invaluable tools in therapeutic intervention and disease management.
Drug Development
In the field of drug development, peptide structure generators are instrumental. They help in designing peptide-based drugs that can target specific diseases. Peptide drugs tend to have high specificity for their targets, often leading to fewer side effects compared to traditional small-molecule drugs. This selectivity arises from the ability of peptides to interact with biological targets in a highly optimized manner. Moreover, the role of structure generators allows researchers to model peptide interactions at a molecular level, significantly speeding up the drug discovery process.
Some key benefits of utilizing peptide structure generators in drug development include:
- Enhanced efficacy: Tailored peptides can effectively target and modulate biological pathways associated with diseases.
- Reduced toxicity: Peptide-based therapies often exhibit lower toxicity profiles, improving patient safety.
- Faster development cycle: By employing computational methods, researchers can rapidly iterate and experiment with different peptide configurations.
Vaccine Design
Peptide structure generators are also prominent in vaccine design. They enable the creation of peptide antigens that can stimulate an immune response. This is particularly relevant in the development of vaccines for infectious diseases and cancers. Utilizing specific peptides, it is possible to induce the production of specific antibodies, enhancing the body's ability to fight pathogens or tumor cells.
Some important considerations in peptide vaccine design include:
- Immunogenicity: Ensuring that the peptides chosen can elicit a robust immune response.
- Stability: Designing peptides that can remain stable during storage and after administration.
- Delivery mechanisms: Developing effective ways to deliver these peptides, such as through nanoparticles or liposomes, to enhance the immune response.
Biomarker Discovery
Another significant application of peptide structure generators is in biomarker discovery. Biomarkers are crucial for the early detection and monitoring of diseases. Peptides can serve as sensitive indicators of disease progression or response to treatment. By utilizing structure generators, researchers can identify and validate new peptides that may function as biomarkers.
The importance of peptide-based biomarkers lies in:
- Specificity: Peptides can be selected based on their specific interactions with disease states.
- Quantitative analysis: Peptide biomarkers can be quantitatively measured, providing accurate data for clinical use.
- Potential for personalization: Peptide biomarkers may allow for tailored treatment strategies based on individual patient profiles.
Challenges in Peptide Structure Generation
Peptide structure generation is a complex field that plays a crucial role in modern biochemistry. However, understanding the challenges within this discipline is just as important. These challenges inform ongoing research and development, influencing the design of future technologies and methodologies. The difficulties encountered in peptide structure generation can impact drug discovery, vaccine development, and various therapeutic applications. Therefore, analyzing these issues is essential for researchers and professionals to navigate this intricate landscape effectively.
Limitations of Current Technologies
Current technologies for peptide synthesis and analysis are valuable but carry several limitations. These limitations can restrict the efficiency and accuracy of peptide structure generation. For instance, traditional methods often struggle with steric hindrance when synthesizing larger peptides. This can complicate folding and primary structure fidelity, ultimately affecting the secondary and tertiary structures that are critical for functionality.
Additionally, the efficiency of solid-phase synthesis and difficulties in purification can lead to incomplete reactions, thereby reducing yield. Other issues stem from the challenges in exhibiting the dynamic nature of peptides in real biological environments. To enhance the performance of peptide syntheis, researchers must innovate and explore alternative strategies, like improving existing methods or developing new ones.
Scaling Synthesis Processes
Scaling peptide synthesis processes remains a daunting challenge in the field. While small-scale synthesis can be manageable, transitioning to larger scales often reveals inefficiencies and inconsistencies. When synthesizing peptides for therapeutic use, maintaining quality and reproducibility becomes essential.
Scaling requires consideration of factors like solvent utilization, reaction conditions, and separation techniques. For example, substances like tris(2-carboxyethyl)phosphine (TCEP) are often used in peptide synthesis yet require careful handling in larger scales. These specifications complicate the scaling process, leading to elevated costs and increased timeframes for research and production. Addressing these challenges is critical for meeting the increasing demand for high-quality peptides in various applications.
Accuracy of Computational Predictions
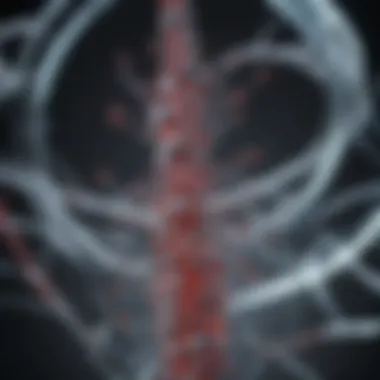
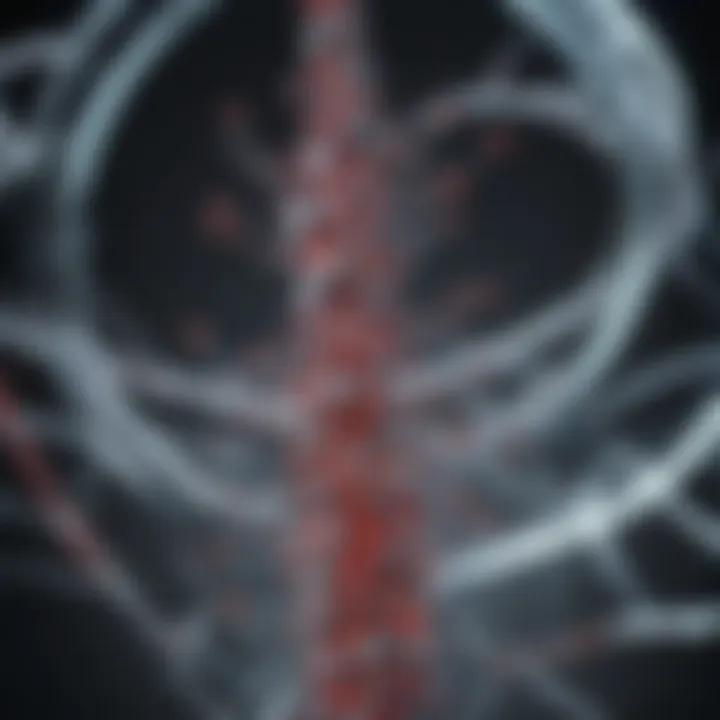
Finally, the accuracy of computational predictions is a significant challenge that researchers face. Computational models are essential for simulating peptide structure generation and dynamics. Nonetheless, these models rely on algorithms and assumptions that can introduce errors. Many models may not fully account for the complexity of the biological environment, which could lead to inaccurate predictions in folding and interactions.
Furthermore, machine learning applications in peptide design, while promising, are still developing. They require large amounts of high-quality data to train algorithms effectively. Differences in peptide sequences and the variable nature of interactions can yield unpredictable results. For researchers, enhancing computational accuracy is vital for developing reliable predictions that can guide synthesis and applications in health sciences.
In summary, identifying and understanding these challenges enhances the discourse around peptide structure generation. Addressing the limitations of current technologies, finding ways to scale synthesis processes effectively, and improving the accuracy of computational predictions will significantly advance this field.
Future Directions in Peptide Structure Generation
The field of peptide structure generation continues to evolve, shaped by new technologies and scientific discoveries. Understanding the future directions of this field is crucial for researchers and practitioners alike. It holds the potential for significant advancements in several domains, especially in drug development and therapeutic applications. This section delves into the specific elements that will define the future of peptide structure generation, noting the benefits and considerations that come with these advancements.
Advancements in Synthesizing Technologies
In recent years, synthesizing technologies have advanced considerably. These innovations enable the production of more complex peptides with higher efficiency and accuracy. Techniques such as automated peptide synthesizers have become more sophisticated, allowing for more streamlined processes. This shift towards automation reduces human error and increases reproducibility in peptide synthesis.
The continuing refinement of solid-phase peptide synthesis allows chemists to generate amino acid sequences with good yields and purity. Reactions can now occur under milder conditions which preserves sensitive functional groups. Moreover, research is investigating new coupling reagents that can enhance the efficiency of the synthesis process.
Key advancements include:
- Enhanced automation: Reduces labor costs and time.
- New coupling methods: Lead to fewer side reactions, improving purity.
- Microfluidics: Is paving the way for miniaturized synthesis, making processes quicker and more efficient.
These advancements are critical for scaling production, making high-quality peptides more accessible for research and therapeutic uses.
Integration of AI in Peptide Design
The integration of artificial intelligence (AI) is poised to revolutionize peptide design. Algorithms can predict the structure and function of peptides based on vast datasets. This could hasten the discovery of novel peptides with specific biological activity. By employing machine learning methods, researchers can analyze patterns that are not readily apparent through traditional approaches.
The potential applications of AI in peptide design include:
- Predicting peptide folding: AI can assist in anticipating how a peptide will fold, which strongly influences its function.
- Enhanced drug design: AI tools can identify peptide candidates for binding to specific biological targets, thus speeding up the drug discovery process.
- Optimization of peptide sequences: Machine learning can suggest modifications to peptide sequences to enhance stability or efficacy.
These innovations not only expedite the design process but can also reduce costs associated with research and development.
Personalized Medicine Approaches
Personalized medicine represents an emerging frontier in healthcare, utilizing individual patient information to tailor treatments. In peptide structure generation, this concept suggests the creation of custom peptides that fit the unique health profile of each patient. Such approaches could lead to the development of targeted therapies that are more effective and pose fewer side effects.
Several considerations arise with personalized medicine in peptide design:
- Patient-specific data: Genetic and proteomic data can guide the design of peptides that specifically interact with patient unique biomarkers.
- Dynamic adaptability: Peptides can be designed to change or adapt in response to a patient's evolving condition.
- Cost and scalability: While promising, personalized approaches may involve challenges in terms of production costs and scalability.
In summary, future directions in peptide structure generation are poised for notable transformations with ongoing advancements in synthesizing technologies, the incorporation of AI, and the rise of personalized medicine strategies. These elements will not only refine existing methodologies but also expand the possibilities of peptide applications in healthcare and biotechnology.
The End
Summary of Insights
The study of peptide structure generators sheds light on many facets of modern science. Some key points to consider include:
- Synthesis Techniques: Both solid-phase and liquid-phase methods have their unique advantages and applications in peptide synthesis.
- Computational Advances: Technologies like molecular dynamics simulations and machine learning have transformed how we approach peptide design, making it faster and more accurate.
- Applications: From drug development to biomarker discovery, the implications of peptide structure generation are broad and significant. These peptides can serve as pivotal components for innovative therapies and preventive medicine.
Overall, the synthesis and analysis of peptides become more integrated into research, establishing new pathways for scientific inquiry and therapeutic interventions.
Implications for Future Research
Future research in the field of peptide structure generation faces both opportunities and challenges. Significant considerations include:
- Technological Integration: Continued advancement in synthesizing technologies and computational methods can lead to improved peptide design.
- Personalization in Medicine: As the understanding of genomics and proteomics deepens, personalized medicine will become more prevalent, necessitating custom peptide designs.
- AI Utilization: The application of AI in peptide design promises to enhance predictive capabilities and streamline the development process.
By addressing these elements, researchers can foster a deeper understanding of peptide behavior and functionality, propelling the field into innovative territories. The ongoing exploration of peptide structure generators will likely reveal novel therapeutic options and refine existing approaches in biochemistry.