Exploring Trends in Face Emotion Recognition Online
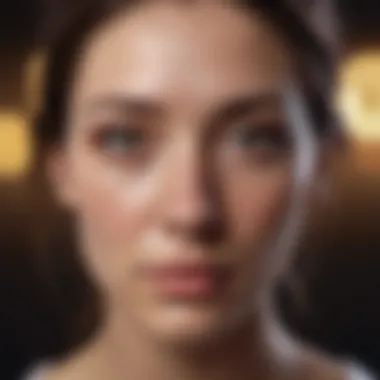
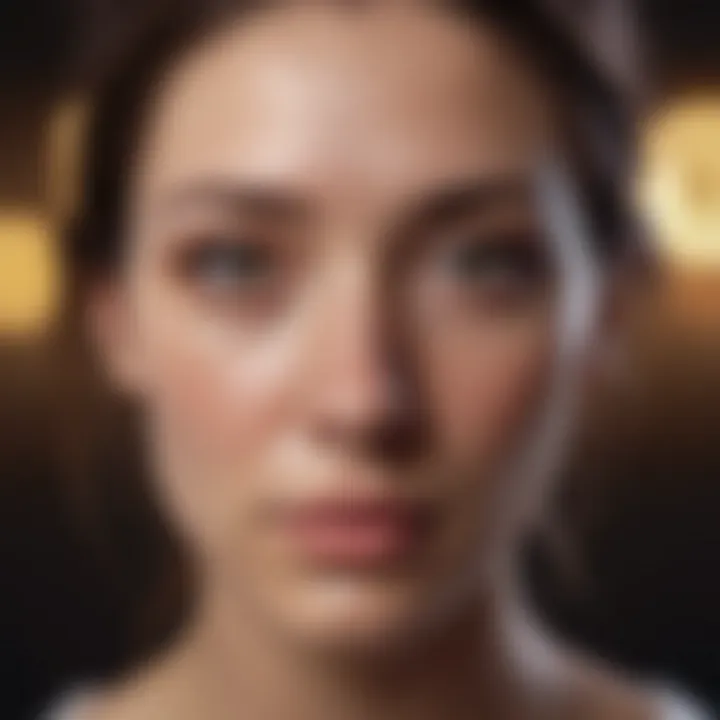
Intro
The field of face emotion recognition online is rapidly evolving. This technology uses algorithms to analyze facial expressions, aiming to determine an individual's emotional state. As methodologies improve, various sectors are looking to this technology for insights.
The relevance of this topic extends beyond simple emotional understanding. It touches on areas like psychology, marketing, and user experience. Researchers are curious about how culture and ethical concerns influence emotional interpretation. This article aims to clarify these points while also exploring future directions in this space.
Prologue to Face Emotion Recognition
Face emotion recognition has become a significant field in artificial intelligence and computer science. This area holds promise for various sectors, including psychology, marketing, and security. The ability to analyze and interpret human emotions through facial expressions offers numerous benefits. It enhances understanding of human behavior and streamlines data collection. Moreover, this technology can boost user experiences by allowing machines to respond appropriately.
With the rise of digital interaction, the relevance of face emotion recognition is even more pronounced. As online communication increases, understanding emotions becomes crucial. This article aims to provide insight into the importance of this technology, exploring its methodologies and applications.
Definition and Scope
Face emotion recognition refers to the task of identifying human emotions from facial expressions. This often involves both hardware and software components that analyze visual data to determine emotional states. Emotion recognition systems typically use camera-based technologies combined with machine learning algorithms to process and classify emotions.
The primary scope includes various types of emotions such as happiness, sadness, anger, and surprise. It encompasses both positive and negative emotional states, and understanding these emotions can lead to further advancements in various fields. Researchers focus on the accuracy and efficiency of these systems, improving the algorithms to better reflect real human emotions. Furthermore, the scope expands to consider the integration of emotional recognition with other technologies.
Historical Overview
The journey of face emotion recognition began several decades ago. Early studies in emotion research laid the groundwork for understanding how emotions manifest on human faces. Psychologists like Paul Ekman played a pivotal role by identifying basic emotions and creating the Facial Action Coding System. This foundation fueled the exploration of how machines might recognize human emotions.
As technology advanced, so did the methods of face emotion recognition. In the late 20th century, with the debut of basic video recognition systems, the foundations for automated recognition were established. The advent of machine learning and neural networks during the 21st century revolutionized the field, vastly improving accuracy and processing speeds.
Recent developments in deep learning have further enhanced capabilities. Researchers now explore complex models that can interpret subtle variations in expressions, taking us closer to mimicking human emotional intelligence in machines. The continuous evolution of hardware technology, such as high-resolution cameras, also contributes significantly to the accuracy of emotion recognition systems.
Understanding this history provides essential context for the ongoing evolution in face emotion recognition technologies. It highlights the path from basic human observation to sophisticated algorithmic processes that can potentially reshape how we interact with machines and one another.
Technological Foundations
The topic of technological foundations is crucial in understanding the dynamics of face emotion recognition. This segment delves into the underlying mechanisms that drive emotion recognition software and systems. By grasping these elements, one can appreciate how they contribute to accuracy and efficiency, as well as the ethical implications that may arise from their use. The synergy between machine learning algorithms and computer vision techniques will be explored. Both of these areas are instrumental in advancing the field, enabling nuanced interpretations of emotional states through facial cues.
Machine Learning Algorithms
Machine learning algorithms form the backbone of face emotion recognition technologies. These algorithms analyze data to identify patterns and make predictions based on input. Three prominent categories are typically discussed: supervised learning, unsupervised learning, and deep learning.
Supervised Learning
Supervised learning is characterized by its reliance on labeled datasets. During training, the algorithm learns from examples where the emotions associated with certain facial expressions are already known. This method is common in face emotion recognition due to its efficiency in achieving precise predictions. The primary benefit of supervised learning lies in its ability to produce reliable models once trained with sufficient data. It allows for a structured approach to model building.
However, a notable drawback is that it necessitates a comprehensive labeled dataset, which can be time-consuming and costly to assemble. Limited availability of diverse data can also pose challenges in model generalization, affecting accuracy across varied demographics.
Unsupervised Learning
Unsupervised learning differs as it does not require labeled output. Instead, it focuses on identifying inherent structures within the input data. This exploratory approach can uncover hidden patterns that might not be evident through supervised means. It is useful when labeled examples are scarce or absent.
The key characteristic of unsupervised learning is its adaptability. By clustering similar features, it can reveal insights into emotional nuances from raw data. Nevertheless, the challenge lies in the lack of guidance, making it difficult to validate the results, as there is no predefined output to compare against.
Deep Learning
Deep learning has gained prominence due to its ability to process large volumes of data. It employs layered neural networks to capture complex relationships in the data. Particularly effective for image analysis, deep learning can enhance the accuracy of emotion recognition tasks amidst varying environmental conditions.
The power of deep learning resides in its automatic feature extraction capability, requiring less manual intervention compared to traditional methods. However, it demands significant computational resources and a large volume of data for training, which may not be feasible for all organizations.
Computer Vision Techniques
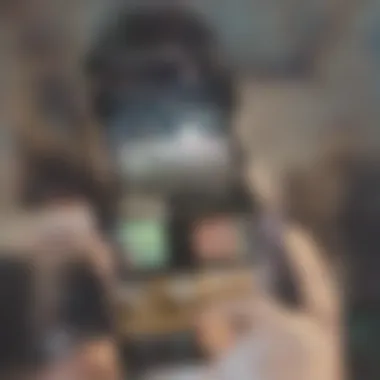
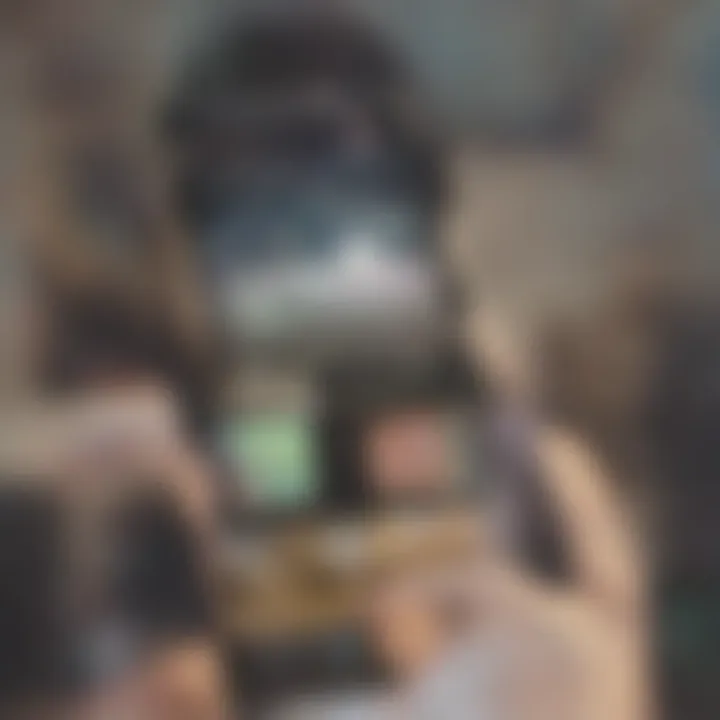
Computer vision techniques complement machine learning algorithms by focusing on how machines interpret and understand visual information. Among these techniques, feature extraction and facial landmark detection are paramount.
Feature Extraction
Feature extraction identifies relevant characteristics within facial images. This process converts raw pixel data into a more manageable format, highlighting essential features that indicate emotional states. It simplifies data while retaining critical information.
The advantage of feature extraction is its role in enhancing the performance of emotion recognition systems by reducing noise. While effective, an overreliance on specific features can lead to a loss of context, possibly hindering the overall accuracy of emotion recognition.
Facial Landmark Detection
Facial landmark detection involves identifying key facial points that define facial structures. This method provides a map of the face, helping systems assess expressions by focusing on changes at specific landmarks.
This technique is beneficial because it allows for increased precision in emotion recognition by concentrating on the most significant parts of the face, like the eyes and mouth. A potential drawback is that variations in head orientation or occlusions can complicate the detection process, potentially impacting recognition outcomes.
It's clear that the integration of both machine learning algorithms and computer vision techniques yields a strong foundation for advancing face emotion recognition technologies. Understanding their strengths and limitations will be essential as the field continues to evolve.
Current Applications of Face Emotion Recognition
The utility of face emotion recognition technology spans various fields, highlighting its potential to change how industries understand human behavior. It is not just a technological concept; it has real-world applications that can improve processes in marketing, mental health, and security. Each of these sectors benefits from the ability to analyze and interpret human emotions through facial expressions, allowing for more informed decisions and improved outcomes.
Marketing and Consumer Research
In marketing, understanding consumer emotions is vital for tailoring advertisements and enhancing customer experiences. Companies leverage face emotion recognition to gauge audience reactions during focus groups and advertisements. By analyzing expressions, brands can identify emotional engagement levels, leading to refined marketing strategies.
- Real-Time Feedback: Companies can get immediate feedback from consumers. This helps marketers adjust campaigns on the fly, optimizing effectiveness based on emotional responses.
- Targeted Advertising: The technology fosters personalized experiences. Advertisements can be directed to resonate with various emotional states, boosting engagement and conversion rates.
This application underscores the significance of aligning consumer products with genuine emotional experiences, fostering a stronger connection between brands and consumers.
Mental Health Assessment
Face emotion recognition also plays a crucial role in the field of mental health. The ability to detect subtle changes in facial expressions can assist therapists and psychologists in understanding patients' emotions better.
- Enhanced Diagnosis: Automated facial analysis tools can help in diagnosing mental health conditions more accurately, particularly for disorders like depression and anxiety, where emotional expression is critical.
- Remote Monitoring: For patients unable to attend in-person sessions, online platforms equipped with emotion recognition can provide remote assessments. This flexibility can lead to better outcomes by maintaining consistent monitoring and support.
This integration can change how mental health professionals approach patient care, making it more responsive and tailored to individual needs.
Security and Surveillance
The application of face emotion recognition technology in security and surveillance raises critical concerns and opportunities. This sector utilizes emotion detection for a broad range of purposes, from monitoring behavior in public spaces to analyzing threats.
- Threat Detection: By identifying signs of anxiety or aggression, security systems can alert personnel to potential threats before they escalate.
- Crowd Monitoring: In events or public gathering, understanding group emotions helps in predicting the likelihood of unrest or disturbances, allowing for preparedness and effective management.
However, it raises questions regarding privacy and ethical use, necessitating a balanced approach as security measures continue to evolve in this area.
In summary, the current applications of face emotion recognition reveal its multidimensional benefits across various sectors. Each application not only enhances understanding but also brings forth considerations regarding ethics and privacy. As we explore further, it is essential to evaluate how these technologies can expand their reach while addressing potential pitfalls.
Ethical Considerations
The implementation of face emotion recognition technology comes with a series of ethical considerations that demand careful attention. This aspect of the technology is essential, as it directly influences individuals' rights, societal norms, and the systems in which these technologies operate. Ethical considerations delve into the implications of data collection, algorithmic fairness, and the adherence to regulations. Addressing these matters not only builds trust among users but also ensures a responsible deployment of this technology in various sectors.
Privacy Concerns
Privacy remains at the forefront of ethical discussions surrounding face emotion recognition. The technology relies heavily on collecting and analyzing facial data, raising concerns about data ownership and user consent. Individuals may not be fully aware that their facial expressions are being captured and evaluated, often in real-time, which could breach personal privacy.
The growing interconnectedness of online platforms and databases further complicates the matter. Facial data could be vulnerable to unauthorized access, leading to potential misuse in various fields, such as marketing or even surveillance. To mitigate privacy risks, businesses and developers must prioritize securing data and obtaining clear consent from end-users. This can involve creating transparent policies that inform users about how their data will be used, ensuring accountability at every step.
"Ensuring privacy in technology that collects sensitive data is crucial to maintaining public trust."
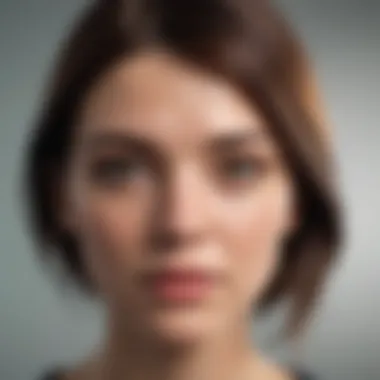
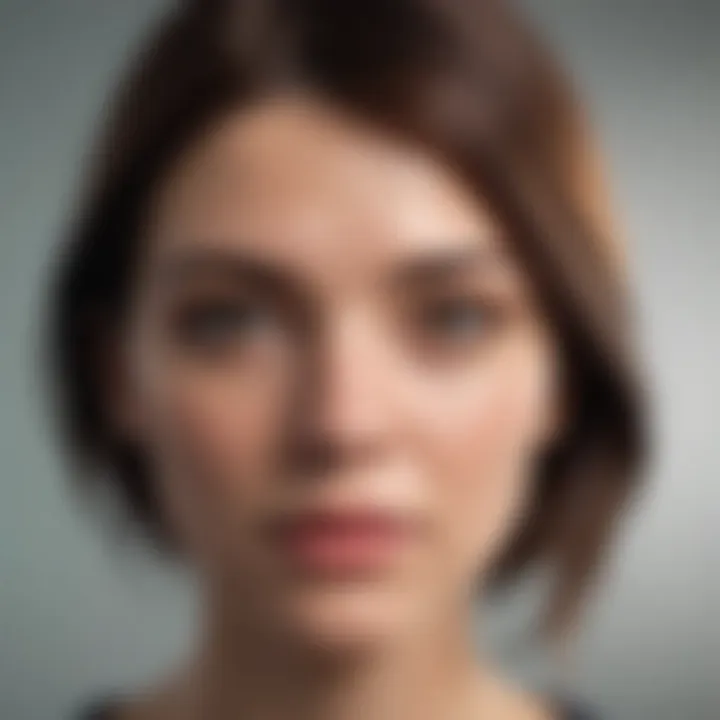
Bias and Fairness in Algorithms
Bias in face emotion recognition algorithms poses another significant ethical challenge. Algorithms learn from historical data, which can unintentionally reflect societal biases, such as gender or race. When these systems process facial information, they may produce outcomes that are unfair or inaccurate, disproportionately affecting specific demographic groups. This can lead to consequences ranging from misinterpretation of emotions to discriminatory practices in hiring, law enforcement, or education.
Developers are increasingly aware of the necessity to train algorithms on diverse datasets that represent multiple demographics. This approach helps ensure that the algorithms can respond fairly across different populations. Continuous monitoring and evaluation of algorithm performances are also essential to detect and rectify biases as they emerge. Overall, the focus on fairness within algorithmic design can promote more ethical outcomes, fostering inclusiveness.
Regulatory Frameworks
Implementing effective regulatory frameworks is crucial in addressing the ethical concerns associated with face emotion recognition. As the technology evolves, so too must the laws governing data collection, privacy, and algorithmic fairness. Clear guidelines must be established to protect individual rights while fostering innovation in the field.
Countries around the globe are starting to recognize the need for regulation concerning facial recognition technologies. In the European Union, the General Data Protection Regulation (GDPR) outlines strict data handling and user consent guidelines. Other regions might consider similar regulatory measures to ensure a balance between technological advancements and ethical considerations.
Cultural Factors in Emotion Perception
Understanding cultural factors in emotion perception is paramount in the realm of face emotion recognition. Different cultures interpret facial expressions in varied ways, which can significantly impact the algorithm’s effectiveness. These interpretations are influenced by societal norms, historical context, and emotional expression practices unique to each culture.
The implications of these differences are critical. For instance, a smile may be perceived as genuine happiness in one culture while being interpreted as a signal of discomfort in another. Therefore, designing face emotion recognition systems necessitates an awareness of these variables, enabling more accurate interpretations and reducing cultural biases.
Cross-Cultural Differences
Cross-cultural differences in emotion perception are at the forefront of discussions in artificial intelligence. Various studies illustrate significant disparities in how individuals from different cultures express and interpret emotions. For example, researchers often find that East Asian cultures tend to emphasize subtlety and context in emotional expression, whereas Western cultures may favor direct and expressive displays of feelings.
These differences are not merely academic; they have real-world consequences in applications of face emotion recognition technology. Developers must consider cultural contexts to avoid misinterpretations that could lead to erroneous outcomes. Consequently, when training machine learning models, it is essential to include diverse datasets representing distinct ethnic and cultural backgrounds. This inclusion can enhance the algorithm's robustness and generalizability across global contexts.
"Cross-cultural awareness is not merely a feature of good design; it is a necessity for ethical AI development."
Cultural Sensitivity in AI Systems
Cultural sensitivity in AI systems is a key consideration for developers and researchers working in face emotion recognition. As these systems are deployed in increasingly diverse environments, ensuring that they respect cultural nuances in emotion interpretation is vital.
When building AI systems, programmers must engage in practices that promote inclusivity and understanding of different emotional landscapes. This may involve collaborating with cultural experts or conducting ethnographic studies to gauge how emotions are perceived and expressed in various societies.
Implementing cultural sensitivity can yield multiple benefits:
- Improved Accuracy: By integrating cultural insights, AI can yield interpretations that align more closely with users' emotional nuances.
- Enhanced User Trust: Users are more likely to trust a system that acknowledges and respects their cultural context.
- Broadened Applicability: Culturally sensitive systems can be used in various fields, from international marketing to cross-cultural mental health assessments.
As the field of online face emotion recognition continues to evolve, recognizing and addressing cultural factors will be essential for maximizing its effectiveness and ethical deployment in varied global contexts.
Challenges in Face Emotion Recognition
Face emotion recognition (FER) online presents unique challenges that must be addressed to enhance its efficacy and reliability. As this technology becomes integral in various sectors, understanding these challenges is paramount. Many factors influence how emotions are recognized from facial expressions. Addressing these difficulties is essential for the development of more robust systems.
Variability in Facial Expressions
Facial expressions are not universal. Different people may express the same emotion in various ways. For example, a smile may be perceived differently depending on cultural context. This variability can lead to discrepancies in emotion recognition algorithms. Training datasets often rely on commonly accepted expressions like the six basic emotions proposed by Paul Ekman: happiness, sadness, anger, surprise, fear, and disgust. However, real-life expressions may deviate from these archetypes.
An additional layer of complexity arises when considering conditions like facial paralysis or specific medical conditions. These factors may alter expressions significantly while the underlying emotion remains the same. Machine learning models trained on typical datasets may struggle in these situations, resulting in misinterpretation. Thus, developing more representative datasets that encompass a wide range of expressions is crucial.
Environmental Influences
Environmental factors can greatly affect facial recognition technologies' accuracy. Lighting conditions, background distractions, and camera angle play vital roles when capturing facial expressions. For instance, poor lighting may obscure details in a face, making it difficult for algorithms to distinguish between different emotional states.
Moreover, the context in which a face is observed can change the interpretation of expressions. The same facial expression may come across as angry in one scenario but as determined in another. Social cues and environmental context must be integrated into recognition models for improved accuracy.
"Understanding context is vital when interpreting human emotions. Without it, the message can be distorted or misunderstood."
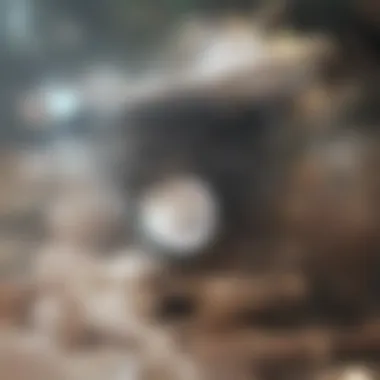
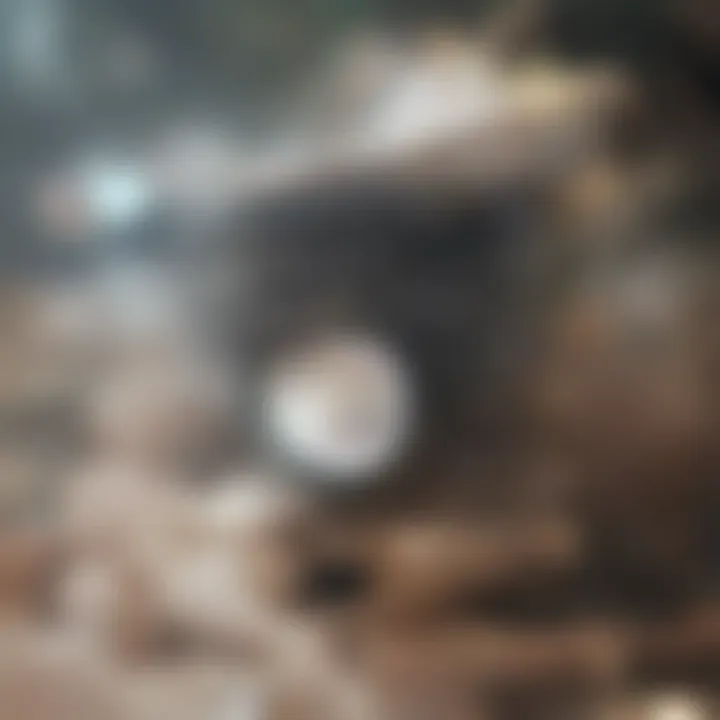
Future Directions
The field of face emotion recognition is rapidly evolving, driven by advancements in technologies and increasing awareness of its applications. This section will explore future directions in this area, focusing on the integration of face emotion recognition with other AI technologies and advancements in real-time processing. These trends signify not only potential improvements in accuracy and efficiency but also broader implications for various sectors.
Integration with Other AI Technologies
Integrating face emotion recognition with other artificial intelligence technologies presents significant opportunities for enhancing capabilities. Such combinations can lead to more nuanced understanding and applications. For instance, integrating natural language processing with face emotion detection allows systems to analyze not only visual cues but also verbal cues. This could lead to better emotional assessments in customer service settings or therapeutic environments.
Another integration worth considering is with machine learning models specifically focused on predictive analytics. By combining emotional recognition with predictive analytics, organizations can forecast trends in user behavior more accurately. This can be particularly beneficial in marketing, where understanding customer emotions is key to crafting effective campaigns.
Moreover, using cloud computing in tandem with face emotion recognition can facilitate data sharing and processing power. This is essential in scenarios requiring large-scale data analysis. With easier access to advanced computational resources, smaller companies and researchers can leverage sophisticated algorithms to enhance their findings.
"The amalgamation of technologies can redefine how we interpret human emotions through digital interfaces."
Advancements in Real-Time Processing
Real-time processing is a pivotal component for the effectiveness of face emotion recognition technologies. Current applications often face limitations regarding latency and speed, which can hinder user experience. Future advancements need to focus on improving these areas. Reducing latency through the development of optimized algorithms will enable more seamless interactions in applications like video conferencing or live marketing.
Advancements in hardware also play a crucial role. The growth of more powerful GPUs and AI-specific chips can enhance the processing capabilities of face emotion recognition systems. These developments can also drive down the cost of deployment, making these technologies more accessible to a wider range of users.
Finally, the refinement of edge computing solutions can play a transformative role. By processing data closer to its source, edge computing can enable quicker data interpretation, vastly improving response times for applications in security and surveillance.
Epilogue
The conclusion serves as a pivotal opportunity to encapsulate the elements discussed throughout the article, shedding light on the evolution and significance of face emotion recognition technology in online contexts. This topic resonates deeply amid the increasing merger of technology with daily life, underscoring its relevance to sectors like psychology, marketing, and security. By summarizing critical findings and considerations, the conclusion highlights the utility of face emotion recognition in understanding human emotions at a distance, enhanced by advancements in machine learning and computer vision.
In the current climate, where data privacy and ethical considerations are at the forefront, the implications of face emotion recognition cannot be understated. Readers must appreciate the intricate balance between leveraging technology for improved emotional comprehension while maintaining ethical boundaries. The integration of cultural sensitivity and fairness in these algorithms ensures that applications of the technology do not perpetuate bias, thus fostering greater acceptance and functionality across diverse populations.
"The growth of face emotion recognition heralds a new frontier in emotional intelligence, promising profound insights into human interaction."
Understanding this nuanced relationship is key to advancing research and development in the field. The balance of innovation and responsibility paves the way for future applications that could redefine conventional approaches to emotional analysis in various domains.
Summary of Key Points
The key points distilled from the comprehensive exploration of face emotion recognition include:
- The evolution of technology has enabled real-time emotion analysis through advanced algorithms.
- Ethical considerations, particularly regarding privacy, bias, and cultural sensitivity, are paramount.
- Applications span multiple domains, including marketing, mental health, and security, showcasing the adaptability of the technology.
- Ongoing research is critical to address current challenges and improve system accuracy in diverse environments.
Final Thoughts on the Future
Looking ahead, the future of face emotion recognition appears promising yet complex. As technological integration deepens within other AI frameworks, the potential for richer data analysis increases. Real-time processing advancements will likely enhance accuracy and efficiency, making these systems more ubiquitous in everyday applications.
Additionally, acknowledging and addressing ethical concerns will be critical for widespread acceptance. Future developments should prioritize transparency and accountability, especially in sensitive fields like healthcare and public safety. In doing so, the technology may evolve to not only recognize emotions but also to cultivate better human experiences and interactions.
As the field progresses, ongoing dialogue among researchers, developers, and ethicists will be essential. This collaborative approach may lead to the establishment of guidelines that ensure the technology serves humanity positively and ethically.
Key Studies and Papers
Several studies have paved the way for understanding face emotion recognition. Each study contributes to a distinct area of knowledge.
- Ekman and Friesen (1978): Their work on the universality of facial expressions laid foundational theories in the study of emotions and their visual representation.
- Zhao et al. (2019): This research highlights advancements in deep learning algorithms applied to emotion recognition in real-time scenarios.
- Wang et al. (2020): The paper explores bias within AI systems, emphasizing the need for more equitable solutions.
These studies serve as essential resources. They guide ongoing research while addressing ethical concerns and technology integration in face emotion recognition.
Online Resources and Tools
In the digital age, numerous online resources and tools enhance the study and application of face emotion recognition. These platforms foster collaboration, share data, and provide essential software tools.
- OpenFace: This open-source facial behavior analysis toolkit utilizes deep learning for emotion recognition and facial landmark localization.
- AffectNet: A comprehensive dataset that allows researchers to train models on various facial expressions and emotions.
- Facebook AI Research: Provides current developments and research initiatives in AI technologies related to emotion recognition.
Utilizing these tools and resources not only advances individual understanding but also contributes to the community's collective knowledge.
Overall, thorough referencing anchored on foundational studies and relevant tools is indispensable in exploring face emotion recognition's current trends and future potential.