Understanding Pricing Algorithms: Dynamics and Implications
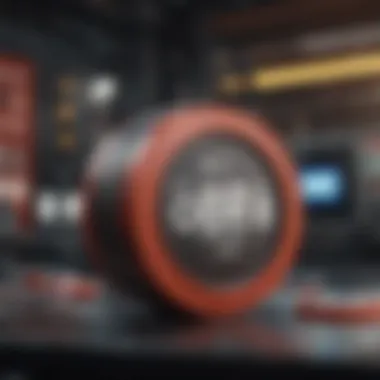
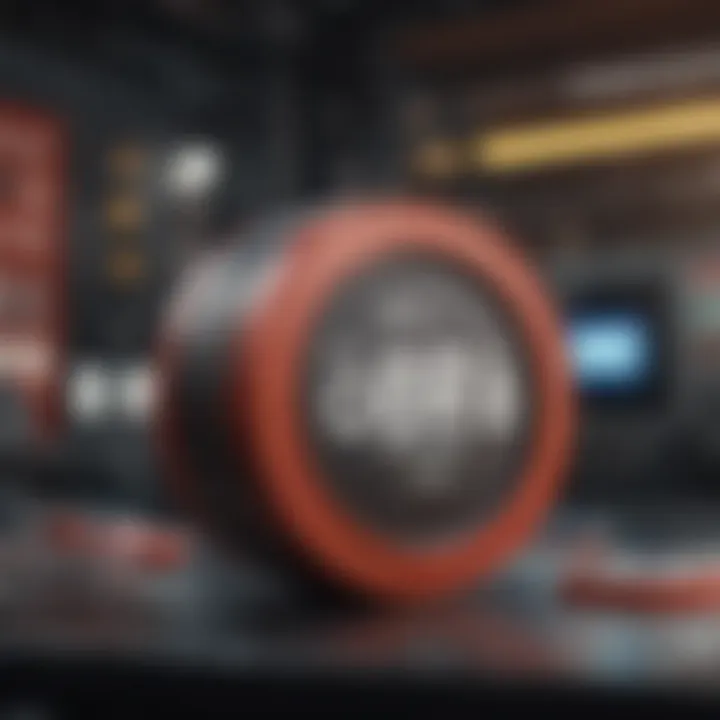
Intro
In today’s tech-driven world, pricing algorithms are more than just a mathematical tool; they are a complex web of strategies that can sway market dynamics significantly. As industries race to optimize their pricing strategies, understanding the mechanisms behind these algorithms becomes essential for anyone involved in commerce or technology. The market is inundated with choices, but the impact of smart pricing cannot be understated. Having a comprehensive grasp of how these algorithms function, their applications across diverse sectors, and the ethical challenges they pose is crucial for navigating this ever-evolving landscape.
This guide will delve into the intricate world of pricing algorithms. It aims to clarify how organizations employ technology to refine their pricing models while grappling with fundamental questions related to fairness and transparency. Through a methodical approach, we will dissect the factors that shape pricing decisions, explore the societal implications of algorithmic pricing, and offer insights into the vital balance between innovation and ethical responsibility.
Research Context
Background and Rationale
The backdrop of our exploration lies in an increasingly competitive marketplace where businesses are relentlessly refining their pricing tactics. Leveraging big data and machine learning, organizations aspire to not just meet but anticipate consumer demands. This shift prompts a closer examination of the algorithms that power these decisions. By dissecting this topic, we aim to unveil the layers that govern pricing in various sectors, and how these algorithms affect consumer behavior at large.
Many practitioners and theorists suggest that understanding these algorithms provides two vital advantages:
- Efficiency: They optimize pricing strategies, ensuring they align with real-time market demand.
- Agility: Quick adaptations to price changes mean businesses can stay ahead of fluctuations in supply and consumer trends.
Literature Review
An examination of existing literature reveals a tapestry of insights from diverse fields, such as economics, data science, and ethics. Research indicates that algorithms can lead to price discrimination, where different consumers pay different prices for the same product based on their perceived willingness to pay. Scholars like
- R. Varian in Price Discrimination and Optimal Pricing, and
- I. Condliffe in various studies on data-driven pricing, provide a foundation for identifying risks and benefits tied to algorithmic pricing. The literature often emphasizes the importance of algorithmic transparency and the need for businesses to ensure consumer trust.
Moreover, ethical considerations about these algorithms often surface in discussions. As algorithms can inadvertently discriminate against specific demographics or become overly complex, the challenge is not only maximizing profit but also ensuring fairness in pricing practices. The existing body of work frequently revisits the need for companies to maintain a balance between competitive advantage and ethical responsibilities to consumers.
Preamble to Pricing Algorithms
Pricing algorithms have become a cornerstone in modern commerce, wielding the power to influence both market dynamics and consumer behavior. At their core, these algorithms represent an intersection of technology, economics, and psychology, enabling businesses to set prices that can maximize profitability while remaining competitive. Understanding these algorithms is essential for businesses aiming to navigate today's rapidly evolving marketplace, where traditional pricing strategies may no longer be sufficient.
Companies utilize pricing algorithms to analyze vast amounts of data ranging from consumer purchasing habits to market trends, crafting strategies that can vary by the minute. This sophistication not only enables businesses to react promptly to changes in consumer demand but also allows them to customize pricing based on individual customer profiles. The implications are profound; a customer shopping at an online retailer might see different prices based on their browsing history and purchasing patterns.
Before diving deeper, it’s essential to grasp the variety of components that define pricing algorithms. They involve various methodologies including data input, analytical techniques, and algorithmic modeling that flows into a cohesive pricing strategy. This diverse tapestry reflects not just mathematical calculations but also an understanding of human behavior and market signals.
In this section, we will look into how we define pricing algorithms and situate them within a broader historical context, tracing their evolution and increasing significance in various sectors.
Definition and Scope
To tackle the definition, pricing algorithms can be articulated as systematic processes that utilize data analysis, mathematical models, and predefined rules to determine pricing structures. They help businesses analyze various factors, such as market demand, customer preferences, and competitive pricing. The scope of these algorithms extends beyond mere number crunching; they also account for psychological pricing and seasonal trends, ensuring that businesses remain agile in a fast-paced environment.
Using algorithms effectively can lead to better market segmentation, personalized pricing, and responsiveness to external economic changes. However, as we shall see, this increased optimization raises various considerations regarding ethics and fairness in pricing.
Historical Context
Looking back, the historical context of pricing algorithms reveals an evolution shaped by technological advancements and economic theories. In the early days of commerce, prices were often set based on intuition and rudimentary market assessments. However, as markets grew and technology advanced, businesses began to recognize the limitations of such approaches.
The advent of computers in the late 20th century marked a turning point. Businesses could now analyze data at an unprecedented scale, leading to the development of more sophisticated pricing models. Early examples included cost-plus pricing strategies and basic demand forecasting. As computers became more capable, so did the algorithms, evolving into models that could incorporate complex variables and real-time data.
By the early 21st century, machine learning techniques started to take precedence, allowing pricing algorithms to learn and adapt independently. Organizations like Amazon leveraged these developments, implementing dynamic pricing strategies that adjusted prices based on algorithms' analyses of competitors, stock levels, and customer behavior in real-time.
In summary, the history of pricing algorithms is a testament to the relentless pursuit of business efficiency and competitiveness, continuously shaped by technological innovations and an ever-changing economic landscape.
Mechanics of Pricing Algorithms
Understanding the mechanics of pricing algorithms is vital to grasping their influence in the contemporary market. These systems are not just mathematical constructs; they are the pulse of many industries, dictating how products are priced and impacting consumer decision-making profoundly. We explore the intricate components that come into play, the benefits of leveraging these algorithms, and the considerations that need to be kept in mind when applying them in real-world scenarios.
Data Input and Analysis
The backbone of any pricing algorithm is the data it processes. Data input varies widely, encompassing everything from historical sales figures to customer demographics and current market trends. This breadth of information allows businesses to create a detailed picture of the market landscape. The ability to analyze and interpret this data meaningfully is what sharpens the effectiveness of pricing strategies. With tools to handle complex datasets, companies can identify patterns in consumer behavior, optimize inventory levels, and adjust prices accordingly.
"Without adequate data analysis, any algorithm is like a ship lost at sea, lacking direction and purpose."
However, the significance of data input goes beyond quantity; quality is paramount. Data must be accurate and relevant to ensure effective algorithmic functioning. Poor data quality can lead to misguided pricing, driving customers away or leaving money on the table instead of capturing potential profits. Thus, organizations need robust systems for data collection and analysis to leverage their pricing algorithms fully.
Algorithmic Models Employed
Pricing strategies are powered by various algorithmic models, each offering unique strengths and weaknesses. Here we will look into three prominent types: Linear Regression Models, Machine Learning Techniques, and Dynamic Pricing Strategies.
Linear Regression Models
Linear regression models provide a straightforward approach to understanding pricing dynamics. They identify relationships between price and other variables like demand or consumer preference. This relationship helps businesses predict outcomes based on historical data trends.
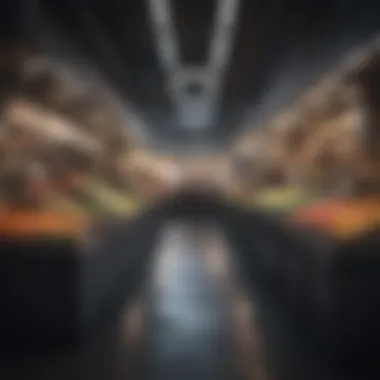
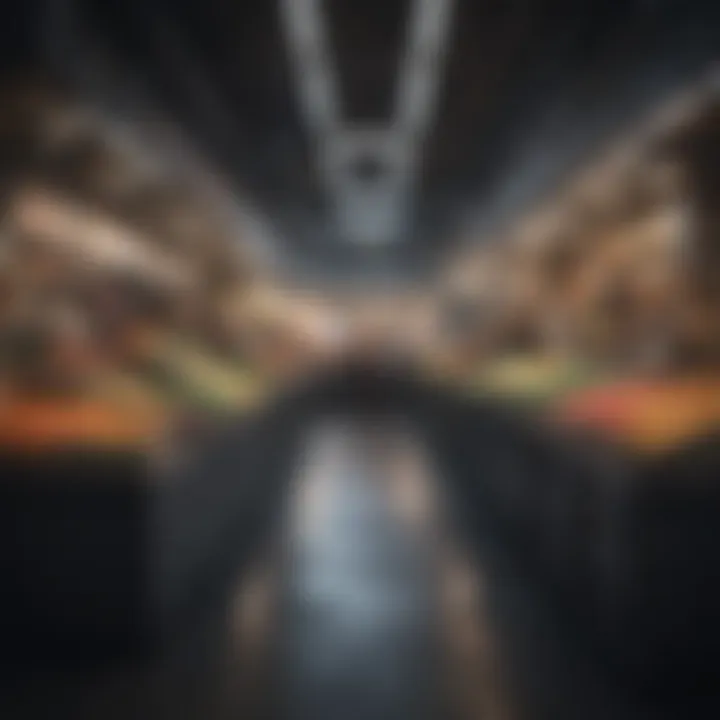
One key characteristic of linear regression is its simplicity. This model is beneficial because it is easy to explain and implement, making it popular among analysts and decision-makers alike. The unique feature of linear regression lies in its ability to demonstrate clear causal relationships, allowing companies to see just how price adjustments might influence sales.
However, its limitation is also evident. Linear regression assumes a constant relationship; it may misrepresent complex market behaviors where interactions between variables can change over time. This challenge necessitates the use of more sophisticated models when the market conditions shift significantly.
Machine Learning Techniques
Turning to machine learning techniques, they represent a leap forward in pricing algorithm design. These models learn from data iteratively, improving their predictions as they process more information. They're especially valuable in environments where patterns are not easily identifiable or where data changes rapidly.
Key characteristics of machine learning include scalability and adaptability. As new data comes in, these models can recalibrate and refine their output, which boosts responsiveness to market nuances.
Machine learning’s unique feature is its handling of vast datasets. Unlike traditional models, it can analyze non-linear relationships and interactions that a human might overlook. Nonetheless, its complexity also poses challenges: the opaque nature of some machine learning algorithms can make it difficult for stakeholders to understand how prices are determined, raising ethical concerns about transparency.
Dynamic Pricing Strategies
Dynamic pricing strategies are a game-changer in the current marketplace. This approach allows businesses to adjust prices in real-time based on varying factors such as competitor pricing, demand spikes, and consumer purchasing habits. With the rise of e-commerce, companies can leverage these strategies to optimize revenue effortlessly.
A standout characteristic of dynamic pricing is its flexibility. Businesses can seize opportunities quickly, aligning prices with current market conditions. This unique feature enhances very competitive markets where price can be a deciding factor for customers.
However, while dynamic pricing can drive revenue, it can lead to customer dissatisfaction if not managed carefully. Frequent fluctuations may erode trust and lead to perceptions of unfairness. Thus, businesses must strike a balance between algorithmic efficiency and customer trust, ensuring they communicate pricing changes clearly to consumers.
Applications Across Industries
Pricing algorithms have ingrained themselves into the fabric of various industries, acting as unseen gears that keep the market machine well-oiled. From e-commerce to hospitality and retail, these algorithms allow businesses to optimize pricing strategies, ultimately enhancing profitability and competitiveness. Understanding their application is not just a matter of convenience—it's vital in comprehending how they shape consumer behavior, market dynamics, and the broader economic landscape.
E-commerce Platforms
In the bustling world of e-commerce, pricing algorithms are the unsung heroes that determine how goods are priced and how sales strategies are devised. Online retailers like Amazon are renowned for adjusting their prices based on real-time data regarding competition, demand, and inventory levels. A notable example is when a product is found to be in high demand; the algorithm may increase the price to maximize profit. On the flip side, items that aren't moving off the virtual shelves might see their prices drop, encouraging quicker sales.
- Dynamic Pricing: This is the name of the game. Algorithms track competitor prices, checking around the clock for optimal pricing that aligns with real-time market fluctuations.
- Personalized Offers: By analyzing user data, e-commerce platforms can send tailored promotional offers to potential buyers, compelling them to act quickly before the deal evaporates.
Consequently, these platforms are not just marketplaces; they are sophisticated ecosystems where pricing plays a pivotal role in the consumer's purchase journey.
Travel and Hospitality
Travel and hospitality sectors have also embraced pricing algorithms with open arms. These industries have complex pricing models because they must balance a multitude of factors—seasonal demand, available inventory, and competitor pricing are just a few.
An airline, for instance, often uses these algorithms to manage seat availability and ticket prices. A last-minute traveler will likely pay a premium compared to someone booking well in advance. This practice not only maximizes revenue but also helps to manage customer expectations about what constitutes a reasonable price.
- Yield Management: A technique used extensively, yield management involves pricing according to predicted demand. Higher prices during peak travel seasons entice potential buyers while maintaining lower prices during off-peak times keeps flights filled.
- Booking Platforms: Websites like Expedia or Booking.com use algorithms to sift through vast amounts of data to provide competitive pricing options for consumers, ensuring they receive the best deals available.
Ultimately, these algorithms shape the customer's experience, often dictating not just when they buy but how much they are willing to pay.
Retail Sector Innovations
The retail sector is witnessing a revolution fueled by the implementation of pricing algorithms. Modern retail relies heavily on data analytics to derive actionable insights on customer behavior and buying patterns.
Consider how brick-and-mortar stores utilize algorithms. They might adjust in-store pricing based on online trends or even the behavior of foot traffic in-store.
- Price Matching Policies: Retailers have begun using algorithms to implement real-time price matching. If a local competitor drops their price, the algorithm can instantly adjust the price within the store to retain customers.
- Promotional Events: Algorithms also play a significant role in determining when to launch sales or promotions. By analyzing historical data, they can suggest the best timing for a sale to maximize interest and profits.
Through these innovations, not only do retailers enhance their revenue streams, but they also build a more responsive relationship with their consumers.
The integration of pricing algorithms across various industries is reshaping the dynamics of market competition, making it essential for organizations to adapt swiftly to gain and maintain a competitive edge.
By grasping how these applications function in different sectors, businesses and consumers alike can navigate the complexities of the market with greater awareness and agility.
Impact of Pricing Algorithms
The impact of pricing algorithms extends far beyond simple number crunching. These algorithms, crafted from layers of data intelligence and economic principles, serve as the backbone for modern pricing strategies across various sectors. Their importance cannot be overstated as they not only determine how much a customer pays but also shape the overall competitive landscape. Ultimately, this leads to market efficiencies, optimizes resource allocation, and influences consumer behavior in ways that are both quantifiable and profound.
Market Efficiency and Competition
At the heart of pricing algorithms lies the promise of enhanced market efficiency. When these sophisticated tools are deployed effectively, they can adjust prices in real-time based on supply-demand dynamics, enabling businesses to stay competitive. For instance, online marketplaces such as eBay or Amazon utilize algorithms that can alter prices every moment based on various factors like competitor pricing, inventory levels, and customer behavior. This sort of agility allows businesses to maximize their margins without alienating customers.
However, there is a darker side to this equation. While smaller players can harness these algorithms to compete against giants, sometimes it can lead to unintended outcomes like price wars that erode profits for everyone involved. Thus, understanding the balance between competitive pricing and profitability is crucial for any organization.
Consumer Behavior Dynamics
Consumer behavior is a complex dance influenced by a myriad of factors, and pricing algorithms play a pivotal role in shaping the moves that consumers make. By leveraging data analytics, businesses can foresee trends and anticipate changes in consumer purchasing patterns, allowing them to adjust their pricing strategies accordingly.
Price Sensitivity
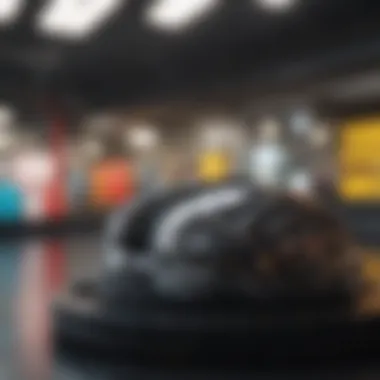
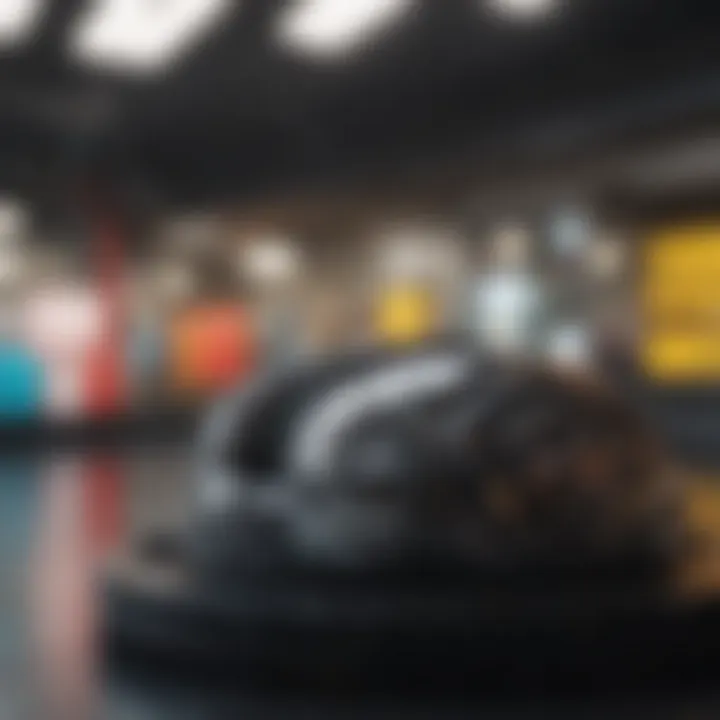
Price sensitivity reflects how responsive consumers are to price changes. In essence, it indicates how much a price increase or decrease can affect consumer buying behavior. For instance, lower-priced products often attract a larger customer base, suggesting a higher price sensitivity in certain market segments. Companies may find it beneficial to implement tiered pricing strategies—offering different price points for different segments to capture maximum value.
One unique aspect of price sensitivity is its interplay with consumer demographics. For example, younger consumers might be more price-sensitive compared to older generations who may prioritize quality over cost. Understanding this nuance not only helps firms refine their pricing strategies but also increases customer loyalty by aligning with consumer expectations.
Perceived Value
Perceived value centers on what a consumer believes a product or service is worth, not just its price tag. It captures that intangible quality that makes buyers feel they are getting their money’s worth. This concept is vital for brands that seek to charge premium prices. For instance, luxury goods thrive on consumers’ perceived value rather than strict price considerations.
The unique feature of perceived value is that it can be influenced by branding, marketing, and social proof. Brands like Rolex capitalize on creating an image of exclusivity and prestige, which often allows them to charge significantly more than their competitors. The advantage here is clear; firms that successfully cultivate a strong perceived value can maintain higher profit margins. However, if consumers begin to question that value—due to a decline in quality or public relations missteps—the results can be disastrous.
"Pricing algorithms affect not just what we pay but also how we think about value in the marketplace."
Ethical and Social Considerations
In the realm of pricing algorithms, ethical and social considerations play a pivotal role that cannot be overlooked. As these algorithms become integral to diverse sectors from e-commerce to aviation, their impact on society expands significantly. Understanding the moral implications of algorithmic decisions is paramount in ensuring they operate within ethical boundaries. This section delves into two critical aspects of ethical considerations: fairness and bias in algorithms, and the need for transparency and accountability.
Fairness and Bias in Algorithms
A significant concern surrounding pricing algorithms is the potential for inherent bias, which may lead to unfair pricing strategies. Algorithms, while designed to be objective, are often built on historical data that may reflect societal inequalities. For instance, if past consumer behavior data indicates a preference for certain demographics, an algorithm could unintentionally disadvantage others. This can pave the way for predatory pricing practices, where certain groups are charged more based merely on their data profiles.
"Algorithms are only as good as the data they are trained on; bad data can lead to unethical outcomes."
To combat this, organizations must take steps to regularly audit their algorithms to spot bias and recalibrate them to promote fairness. Creating diverse datasets is crucial to reduce skewed outcomes. Moreover, there have been ongoing discussions in various industries regarding the implementation of fairness metrics that would gauge the equity of algorithmic decisions.
- Engage with community feedback to refine algorithms.
- Develop guidelines for equitable pricing decisions.
- Implement regular audits to assess algorithm performance.
Transparency and Accountability
In an age where technology governs many facets of life, transparency and accountability emerge as fundamental pillars that support ethical pricing algorithms. Consumers today demand clarity on how prices are determined. Lack of transparency can breed distrust, leading to reluctance in using services, as people may fear being taken advantage of without knowing the pricing rationale.
In response, companies should strive to provide explicit explanations of their pricing strategies, perhaps by describing how data influences price changes. It can be beneficial to disclose the factors that contribute to price fluctuations, whether they are based on demand, supply, or even competitor pricing.
Furthermore, accountability is essential in addressing errors that arise from algorithmic pricing strategies. If a pricing error occurs—whether it's an overcharge or sudden price drop—there should be mechanisms in place to correct this swiftly and effectively. Customer service transparency, with clear channels for addressing pricing disputes, fosters a sense of trust.
- Publish accessibility reports on algorithm performance.
- Offer customer service training for staff handling pricing concerns.
- Encourage community engagement through surveys for feedback on pricing satisfaction.
Technological Advances in Pricing Algorithms
The realm of pricing algorithms is constantly evolving, shaped by rapid advancements in technology. These innovations are not merely enhancements; they represent a transformation in how businesses perceive and implement pricing strategies. As organizations increasingly look to leverage technology to stay competitive, understanding these advances becomes crucial. They allow for more refined decision-making processes, adapt to real-time market conditions, and deliver smarter pricing solutions.
Artificial Intelligence Integration
One of the most significant strides in pricing algorithms is the incorporation of artificial intelligence. AI capabilities allow for deeper data analysis and predictive modeling, leading to more responsive and tailored pricing strategies. For instance, consider how retail giants like Walmart harness AI to assess vast amounts of data, from customer shopping habits to seasonal buying trends. This integration of AI enables algorithms to learn from past behaviors and forecast future demand with greater accuracy.
The benefits of using AI in pricing include:
- Enhanced Predictive Accuracy: AI can identify patterns that humans might miss, offering insights based on complex data correlations.
- Real-Time Adjustments: With market conditions changing by the second, AI-driven algorithms can adjust prices instantaneously to reflect supply and demand fluctuations.
- Personalization: By analyzing customers' purchasing behaviors, pricing algorithms can deliver personalized pricing strategies, which can significantly influence consumer spending.
However, as organizations embrace AI in pricing strategies, they also encounter challenges. For instance, balance must be struck between automation and ethical considerations regarding consumer treatment. It’s important to ensure that while AI optimizes pricing, it does not lead to unfair practices or price surging during critical times, such as natural disasters or global pandemic situations.
The Role of Big Data
The integration of big data is another pillar supporting the advancement of pricing algorithms. Organizations are now equipped to analyze a myriad of data sources—from user behavior across e-commerce platforms to economic indicators. This wealth of information provides invaluable insights that help in making informed pricing decisions.
Utilizing big data involves:
- Data Aggregation: Collecting data from various sources, including transaction history, social media interactions, and competitor pricing.
- Data Analysis Tools: Employing sophisticated analytical tools to interpret this data can reveal significant trends and consumer preferences that would otherwise go unnoticed.
The implications of harnessing big data in pricing strategies include:
- Informed Decision-Making: An analytical approach can aid organizations in setting prices that align with market expectations while also maximizing revenue.
- Risk Mitigation: Understanding market trends and customer behavior can help in anticipating shifts and preparing accordingly, thus reducing financial risk.
- Granular Insights: Organizations can segment their customer base more effectively, leading to targeted pricing strategies that resonate with different consumer segments.
To sum up, the intersection of AI and big data in pricing algorithms is reshaping the landscape of pricing strategies. As the pace of technological change accelerates, those who spare no effort in adapting to these trends will likely outperform their competitors, reinforcing the need for ongoing engagement with these advancements.
Challenges Faced by Pricing Algorithms
The realm of pricing algorithms, while brimming with potential, is riddled with complexities that present significant challenges. Companies leveraging these algorithms often find themselves navigating a landscape marked by volatility and uncertainty, as well as grappling with issues regarding data integrity. It is crucial to understand these challenges as they can significantly influence the efficacy of pricing strategies, consumer trust, and overall market dynamics.
Market Volatility and Uncertainty
Market volatility can feel like a tempestuous sea, with unpredictable waves that can capsize even the most robust pricing strategies. Pricing algorithms, which aim to respond to market conditions in real-time, often struggle against sudden and drastic changes. For instance, during economic downturns, consumer spending habits can shift dramatically, making historical data less reliable for forecasting future trends.
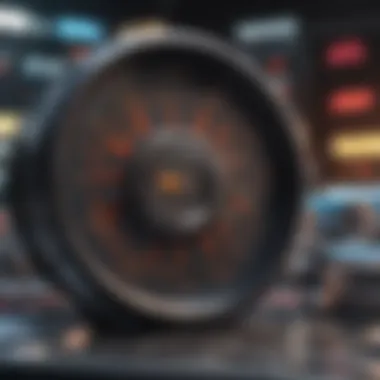
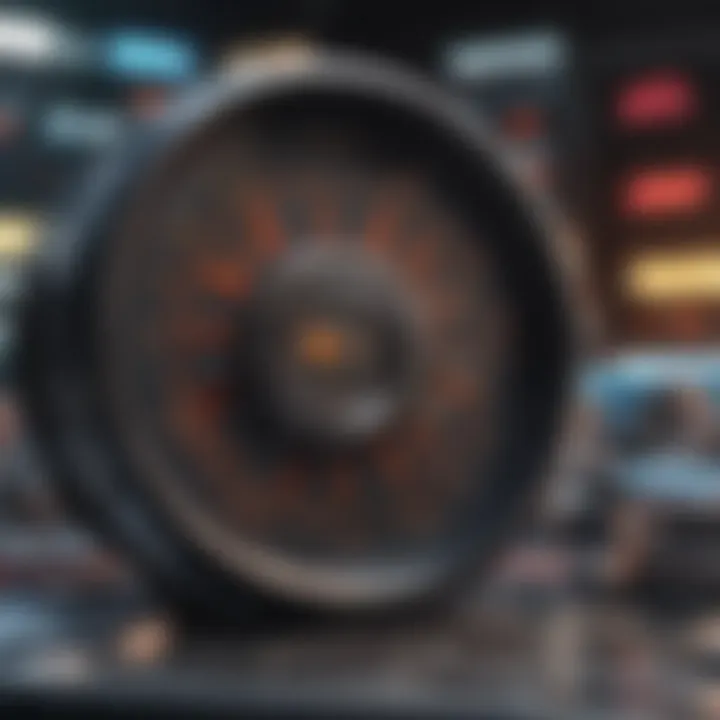
Furthermore, external factors such as political instability, natural disasters, or sudden changes in consumer preferences can create a ripple effect through the market that pricing algorithms might not be designed to handle. This unpredictability necessitates an adaptive approach, where algorithms must be frequently recalibrated to account for new information. Companies are then left in a perilous position, forced to choose between adapting quickly to maintain competitiveness or relying on established models that could lead to mispricing.
"Volatility in markets is like surfing; it takes skill to ride the waves without crashing."
Handling Data Quality Issues
The foundation of any effective pricing algorithm is high-quality data. Yet, this is often where the trouble begins. Data quality issues can plague organizations, from inconsistencies in data collection methodologies to incomplete datasets. Imagine trying to bake a cake with half the ingredients missing; the outcome is rarely pleasing.
In practice, this might mean dealing with outdated information or errors that can skew analysis and result in misguided pricing strategies. For example, if an e-commerce store relies on sales data that only reflects a small portion of its customer base, its pricing decisions could alienate a larger audience. Moreover, integration of varied data sources can lead to incompatibilities, where data from one platform doesn’t align with another, further muddying the waters of insight.
To navigate these issues, companies must establish robust data governance frameworks. This includes consistent veriification processes, periodic audits, and investment in technology that ensures data accuracy. In doing so, organizations can create a reliable foundation upon which to build their pricing strategies, thereby reducing the risk of poor decision-making.
Future Trends in Pricing Algorithms
The landscape of pricing algorithms is continuously shifting, influenced by advancements in technology, consumer behavior, and regulatory frameworks. Understanding these future trends is crucial, as they not only shape how businesses operate but also affect market dynamics and consumer experiences. As we peep into the crystal ball, several specific elements stand out that could redefine the mechanisms behind pricing strategies.
Evolving Regulatory Landscape
The regulatory environment surrounding pricing algorithms is becoming increasingly complex. Governments and regulatory bodies are beginning to scrutinize how these algorithms impact competition and fairness in the marketplace. For example, many nations are evaluating legislation aimed at preventing price discrimination and ensuring transparency in algorithmic pricing.
- Policy Frameworks: Different jurisdictions are establishing regulations that encourage developers of pricing algorithms to disclose their data sources and pricing strategies, creating a more accountable environment. The rise of such frameworks means that businesses must be proactive in ensuring their algorithms align with local laws.
- Consumer Protection: Regulations may also focus on protecting consumers from deceptive pricing practices. As pricing algorithms become more sophisticated, the risk of unintentional bias becomes apparent, which regulators will likely aim to mitigate.
"The opacity surrounding pricing algorithms could be a double-edged sword; while they enhance efficiencies, they may also lead to unintended consequences if left unchecked."
Consumer Expectations and Responsiveness
With the rise of technology and easy access to information, consumer expectations have evolved dramatically. Today’s consumers are not only price-sensitive but also value effectiveness, speed, and personalization. This heightened awareness and expectation shift the dynamics for businesses that use pricing algorithms.
- Demand for Personalization: Customers increasingly expect personalized pricing based on their buying habits, preferences, and even their location. Companies might leverage past purchase data to predict future needs and adjust prices accordingly, leading to a tailored shopping experience that can increase customer loyalty.
- Real-time Adjustments: The ability to respond and adapt to real-time market changes will become a hallmark of successful pricing strategies. Algorithms that can analyze shifts in demand more swiftly will provide a significant competitive edge.
- Enhanced Transparency: As consumers become more educated about how algorithms work, there will be a push for transparency in pricing strategies. Consumers will want to understand why they are offered certain prices or discounts, making it vital for businesses to communicate their pricing structures clearly.
Understanding these future trends provides valuable insights into how pricing algorithms will evolve. As we move forward, keeping an eye on regulatory changes and consumer sentiments will be central to navigating the complex world of algorithm-driven pricing.
Case Studies of Successful Implementation
Understanding success stories of pricing algorithms is more than just a look at numbers or graphs; it is about seeing how real companies have adapted mechanisms in this arena to their strengths and needs. Case studies serve as a roadmap. They provide insights into what works, allowing businesses to learn from the triumphs and mistakes of others. In this section, we'll delve into two notable implementations: one by Amazon and the other focusing on the airline industry.
Case Study: Amazon's Dynamic Pricing
Amazon, the giant of e-commerce, is often considered a pioneer in utilizing dynamic pricing strategies. Their approach hinges on adjusting prices in real-time based on various factors, such as demand fluctuations, competitor pricing, and even inventory levels.
The significance of this strategy cannot be understated. By continuously analyzing vast amounts of data, Amazon can offer customers competitive prices while maximizing profits. This strategy is akin to a chess game where each move is calculated based on the opponent's position. The resources they dedicate to data analysis and algorithmic adjustments are enormous.
Benefits observed from Amazon's strategy include:
- Increased sales: The ability to set a price point that captures more consumer interest boosts overall sales figures.
- Inventory management: Products that are slow in sales can see a reduction in pricing, helping to clear out stock efficiently.
- Consumer engagement: Frequent price changes can entice customers to keep checking back, creating a habit that can lead to higher overall spending.
However, important considerations are also present here. Amazon's aggressive pricing can sometimes lead to consumer skepticism and accusations of price manipulation, which creates a need for transparent practices that built trust with customers.
Case Study: Airline Revenue Management Systems
Moving to the travel industry, the implementation of pricing algorithms in airline revenue management demonstrates the sophisticated dynamics at play. Airlines use complex algorithms that factor in demand, booking patterns, competitor prices, and even day of the week to set ticket prices. What stands out is the highly variable nature of airline pricing—a single seat on a flight can have multiple prices based on when a ticket is purchased.
This practice is essential in maximizing revenue for airlines, especially given the slim profit margins they often work with. The models they use are not just numbers game; they involve extensive forecasting and behavioral analysis.
Key Elements of Airline Revenue Management include:
- Price Elasticity: Airlines understand how sensitive consumers are to price changes, allowing them to capture yield from different market segments effectively.
- Booking Models: Algorithms predict when consumers are likely to purchase tickets, enabling airlines to set dynamic prices that change as the flight date approaches.
- Competitive Pricing: Continuous monitoring of competitor prices means that airlines can adjust theirs almost instantaneously to remain competitive without sacrificing margins.
Closure and Reflections
In the landscape of today’s technologically driven economy, the relevance of pricing algorithms cannot be overstated. In this article, we have traversed the intricate mechanisms powering these algorithms, delved into their real-world applications, and dissected their profound impact on market dynamics. The conclusions drawn here aren't just academic; they carry tangible implications for businesses and consumers alike.
Synthesis of Findings
First and foremost, we must appreciate how pricing algorithms operate at the intersection of data science and business strategy. By leveraging vast amounts of data, these algorithms fine-tune pricing strategies, ensuring they remain competitive in an ever-changing market. Factors such as demand fluctuations, competitor pricing, and consumer behavior patterns are constantly analyzed. This enables organizations to respond swiftly to market changes, optimizing revenue without alienating potential customers.
As observed, various industries have witnessed transformative shifts due to the integration of these pricing models. For instance, in e-commerce, Amazon has set a new standard, utilizing dynamic pricing to capture market segments effectively. Similarly, the airline industry exemplifies sophisticated revenue management tactics that operate on algorithms designed to maximize bookings and enhance customer satisfaction.
Moreover, ethical considerations cannot be brushed aside. The biases inherent in algorithmic designs pose significant concerns about fairness and transparency. Organizations must prioritize accountability and strive for a deeper understanding of how these algorithms function, ensuring that consumer trust is not merely an afterthought.
The Path Ahead for Pricing Algorithms
Looking ahead, we must anticipate the evolving landscape that pricing algorithms inhabit. Regulators are progressively honing in on the ethical implications of algorithmic decision-making. Companies will likely face increased scrutiny aimed at ensuring fair pricing practices. As algorithmic models advance, it is essential for businesses to remain not only compliant but also proactive in addressing these ethical standards.
Furthermore, consumer expectations are shifting. Individuals are increasingly aware of how pricing decisions affect their purchasing power and are demanding more transparency and fairness. As businesses respond to these pressures, integrating public sentiment into pricing strategies may very well become the status quo.
To wrap it up, the journey of understanding pricing algorithms transcends the realm of numerical equations. It involves a blend of technology, psychology, and ethical responsibility. The implications of their use venturing into CRM, product positioning, and brand loyalty are profound. Those who grasp the nuanced dynamics at play will not only navigate the complexities of modern pricing but also lead the charge toward a more equitable marketplace.
"The price is what you pay. The value is what you get." — Warren Buffett
In summary, the intricate tapestry of pricing algorithms illustrates a snapshot of how contemporary businesses operate. Their evolving nature, intertwined with technological advancements, challenges us to rethink market strategies while upholding the values of fairness and transparency. As we move forward, it is vital that we constantly reflect on these aspects to align our practices with the expectations of a data-centric society.