Single Cell Bioinformatics: Methodologies and Insights
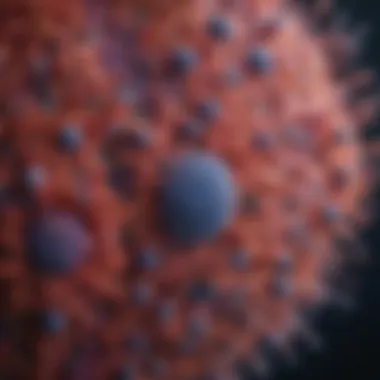
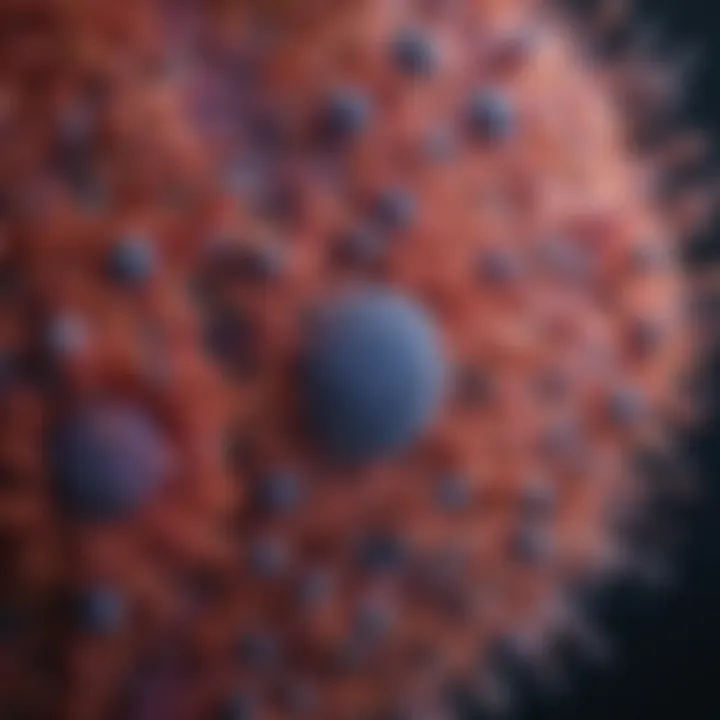
Intro
In recent years, single cell bioinformatics has catapulted into the spotlight of biological research, driven by the increasing recognition that cells are not merely uniform building blocks of life. Instead, they exhibit a remarkable variety, each playing a distinct role within tissues and organs. This understanding has made it imperative to develop techniques that can dissect the intricate behaviors and interactions at the individual cell level. As such, this article will provide a detailed overview of the developments, methodologies, and the significance of this rapidly evolving field.
Research Context
Background and Rationale
The journey into single cell bioinformatics begins with acknowledging the limitations of bulk tissue analysis. Traditional methods can obscure vital differences between individual cells, particularly in heterogeneous populations such as tumors or immune cell mixtures. For instance, consider a tumor made up of numerous cell types. Treating the tumor as a single entity masks the distinct responses each cell type has to various treatments. Thus, the need for single-cell analysis arises from both scientific and therapeutic imperatives.
The rationale is straightforward: By examining individual cells, researchers can uncover hidden phenomena that drive biological processes. Whether it be specific pathways activated in response to stimuli or genetic variances that contribute to drug resistance, the insights gained are profound.
Literature Review
The literature regarding single cell bioinformatics has expanded tremendously, offering various methodologies that cater to different types of experimental questions. A significant paper by Trapnell et al. (2014) in Nature Biotechnology laid the groundwork for single-cell RNA sequencing techniques that have since become pivotal. Meanwhile, studies exploring how single-cell techniques correlate with clinical outcomes in cancer therapy continue to emerge. Essential resources such as Wikipedia offer summaries of key advancements.
From the early days of single-cell genomics to the more recent incorporation of machine learning approaches, current literature reflects a multifaceted approach to tackling relevant questions. Researchers have increasingly emphasized the need to integrate experimental and computational strategies to harness the full potential of single cell analysis, making literature reviews a vital aspect of oneās understanding in this area.
Methodology
Research Design
The design of studies in single cell bioinformatics often requires a multidisciplinary approach, blending biology, computational sciences, and statistics. This integration allows researchers to tackle complex biological questions with precision. Modern research designs may incorporate longitudinal data to follow changes in cellular behavior over time or spatial data to understand cell behaviors within their native contexts.
For instance, a study on dendritic cells might utilize both single-cell RNA sequencing and mass cytometry to dissect how individual cells respond to infections differently. As this field is still maturing, researchers continuously refine their designs to better capture the often fleeting biological phenomena at the single-cell level.
Data Collection Methods
Data collection in single cell bioinformatics predominantly revolves around cutting-edge technologies. Some of the most prominent methods include:
- Single-cell RNA sequencing: This is perhaps the workhorse of the field, allowing researchers to analyze gene expression at the single-cell resolution.
- Mass cytometry: By employing heavy metal tags for cellular proteins, researchers can achieve deep profiling of individual cells.
- Spatial transcriptomics: This method provides a spatial context to gene expression data, allowing scientists to visualize how cells interact in their native tissue environments.
The choice of data collection techniques often hinges on the research question at hand, making it crucial for scientists to stay abreast of the latest technological advancements.
"The ability to analyze individual cells has transformed our understanding of biology, leading us into a new era of precision medicine."
In summary, the landscape of single-cell bioinformatics is as complex and diverse as the organisms it studies. With continuous advancements in methodologies and technologies, researchers are on the brink of uncovering fascinating insights that could redefine our understanding of biology and medicine.
Preamble to Single Cell Bioinformatics
Single cell bioinformatics has emerged as a pivotal domain in the life sciences, allowing researchers to delve into the complexities of cellular processes at an unprecedented resolution. The significance of this field cannot be understated; it opens doors to understanding the intricate behaviors and interactions of individual cells, rather than relying solely on bulk analyses that mask heterogeneity within populations. The advent of single cell technologies not only enhances our grasp of biological responses but also informs therapeutic developments, offering a comprehensive approach to personalized medicine.
Defining Single Cell Bioinformatics
At its core, single cell bioinformatics refers to the methodologies, computational tools, and analytical frameworks designed for studying biological data derived from single cells. Unlike traditional bioinformatics, which typically processes data from aggregated cell populations, single cell bioinformatics takes the spotlight off the average and shines it on the unique characteristics of individual cells.
This field encompasses various aspects, including single cell RNA sequencing, which reveals gene expression profiles specific to each cell type, and single cell genomics, which addresses genetic variations among cells. Moreover, the application of machine learning and advanced algorithms enables researchers to better interpret and visualize complex data sets, driving forward our understanding of cellular functions and interactions.
Historical Context and Evolution
The evolution of single cell bioinformatics is a remarkable journey, reflecting rapid advancements in technology and a growing appreciation for cellular diversity. Historically, the analysis of cellular behavior relied heavily on methods that aggregated data, often leading to oversimplification of biological phenomena. Citizens of the scientific community began to recognize that crucial insights were often lost in the noise of population-level analysis. This prompted a shift toward methodologies that could dissect the cellular landscape.
In the early 2000s, foundational techniques such as flow cytometry and microdissection laid the framework for single cell studies. The arena took a significant leap forward with the introduction of single cell sequencing technologies, which allowed for massive parallel sequencing of RNA and DNA from individual cells. This shift not only transformed how biologists view cellular functions but also set the stage for what we now consider a golden era of single cell bioinformatics.
"Single cell bioinformatics is the lens through which we can truly appreciate the vast complexity of life at the cellular level."
Today, this field weaves together various aspects of genomics, transcriptomics, and proteomics, contributing to fields such as developmental biology and oncology. As we look ahead, itās clear that single cell bioinformatics will continue to shape our understanding of life's building blocks and their roles in health and disease.
Methodologies in Single Cell Analysis
Understanding the methodologies in single cell analysis is crucial, as they serve as a foundation for further advancements in this dynamic field of bioinformatics. By employing state-of-the-art techniques, researchers can peel back the layers of complexity that often mask individual cellular behavior. These methodologies enable a detailed exploration of cellular heterogeneity, allowing for insights into processes that could go unnoticed in bulk analyses. As the demand for personalized and targeted treatments increases, the significance of robust methodologies cannot be understated.
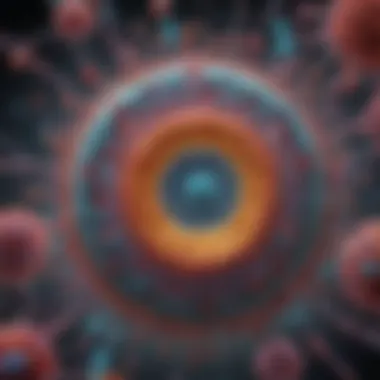
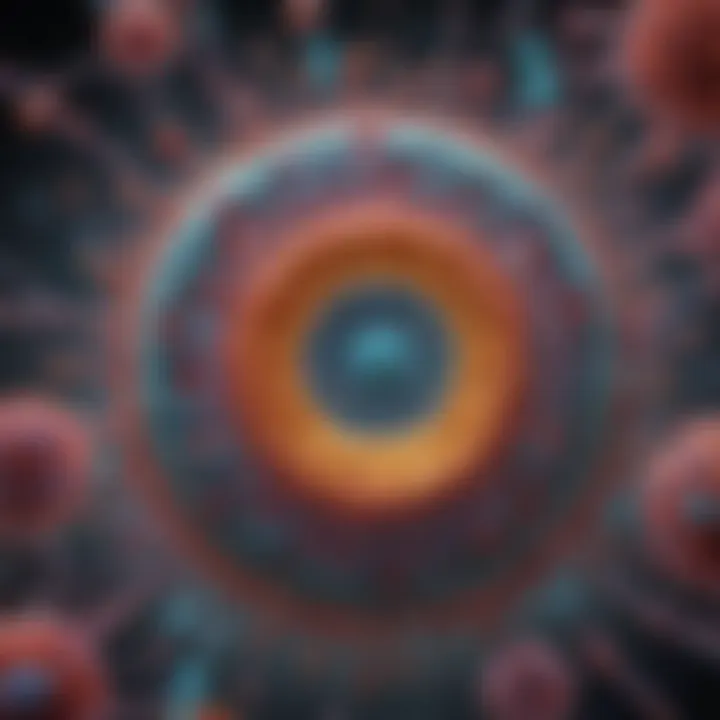
Technological Advances in Single Cell Sequencing
The rapid evolution of single cell sequencing technologies stands at the forefront of this domain. With the development of techniques such as droplet barcoding and miniaturized sequencing platforms, scientists now have the ability to analyze thousands of cells in a single experiment. The emergence of these technologies has served to democratize the access to single cell analysis, making it easier and more cost-effective to conduct comprehensive studies.
The key characteristic of these advancements is their ability to improve throughput while maintaining the fidelity of data. Take, for instance, the method known as 10X Genomics' Chromium platform, which allows researchers to capture and profile transcriptomes from individual cells with ease. This technology not only boosts efficiency but also opens the door to more nuanced observations about cell behavior, which can be pivotal when unraveling complex biological pathways.
However, it's essential to consider the limitations inherent to these technologies as well. For example, while these methods provide high-resolution data, the interpretation of such data requires advanced computational tools and considerable expertise. This balancing act between technology and data interpretation underscores the need for a multi-faceted approach to research.
Data Integration Techniques
Data integration techniques play an indispensable role in single cell bioinformatics, allowing researchers to weave together diverse datasets into a cohesive narrative. The ability to merge information from various omics layers or experimental conditions enhances the interpretative power of the data.
Multi-Omics Approaches
Multi-omics approaches are one of the most promising data integration techniques. This method involves the simultaneous examination of multiple biological layers, such as genomics, transcriptomics, and proteomics. By employing a multi-pronged strategy, researchers can build a more complete picture of the phenomena under study.
One of the standout features of multi-omics approaches is their capacity to reveal interactions between different biological systems. For instance, understanding how protein levels influence gene expression can provide vital clues about metabolic pathways. This interconnected view is a strong selling point as it leads to more accurate models of cellular behavior, which is a critical advantage in fields like disease research and therapeutic targeting.
Nonetheless, there are disadvantages to consider. The complexity of integrating such diverse data types can lead to challenges in standardization and interpretation. This adds a layer of difficulty that researchers must navigate while making sense of their findings.
Spatial Transcriptomics
Spatial transcriptomics, another innovative data integration technique, facilitates the mapping of gene expression within tissues. This method helps researchers capture the spatial context of cellular interactions, which is essential for understanding tissue architecture and function. By identifying where certain genes are being expressed in a tissue sample, scientists can glean insights into physiologically relevant processes.
A key characteristic of spatial transcriptomics is its ability to preserve the spatial information of the samples, allowing for more accurate interpretations of how cells communicate within their environment. This is particularly advantageous in studies related to developmental biology and cancer, where spatial relationships can greatly influence cellular behavior and function.
Despite its strengths, spatial transcriptomics can be cost-prohibitive, and the technical expertise required to implement these techniques is a barrier for many research teams. Thus, while promising, it requires thoughtful application and consideration of resource availability.
Data Analysis and Interpretation
In the realm of single cell bioinformatics, the importance of data analysis and interpretation cannot be overstated. This aspect not only fuels the understanding of biological systems at a granular level but also bridges the gap between raw data and meaningful scientific insights. Through meticulous analysis of data collected from single cell studies, researchers can unveil hidden patterns and draw conclusions that inform further investigations and clinical applications.
Focusing on specific elements, benefits, and considerations in data analysis is crucial as this journey encompasses various methodologies and frameworks that guide researchers in their quest for knowledge. The end goal remains clear: to distill complex single cell data into a format that is accessible, understandable, and applicable to real-world problems.
Moreover, effective data analysis improves reproducibility, making it easier for other scientists to verify findings. This collective effort leads to a more robust scientific community that is equipped to tackle the inherent challenges of modern biological research.
Common Data Analysis Pipelines
In single cell bioinformatics, data analysis pipelines serve as systematic approaches to handle and interpret vast amounts of data from sequencing technologies. The primary steps within a common pipeline often include data preprocessing, normalization, dimensionality reduction, clustering, and differential expression analysis. Letās take a closer look at each step:
- Data Preprocessing: This initial phase involves filtering out low-quality data and correcting any discrepancies within the dataset. It ensures that the following analysis is conducted on high-quality information.
- Normalization: This is where bias due to technical variations is addressed. Techniques such as size factor normalization or log transformation are commonly employed to create a less skewed representation of gene expression.
- Dimensionality Reduction: Given the high dimensionality of single cell data, methods like Principal Component Analysis (PCA) or t-Distributed Stochastic Neighbor Embedding (t-SNE) are often employed to simplify data visualization and analysis.
- Clustering: Clustering techniques help segment the cells into distinct groups based on shared characteristics or gene expression profiles, allowing researchers to compare cellular states.
- Differential Expression Analysis: This step identifies genes that are significantly expressed between different clusters or conditions, providing clues to underlying biological mechanisms.
Data pipelines, therefore, represent fundamental methodologies that guide researchers through the labyrinth of data from single cell experiments, ensuring a systematic and thorough approach to analysis.
Challenges in Data Interpretation
The road to effective data interpretation is laden with challenges that can significantly impact the outcomes of single cell studies.
Variance in Single Cell Data
Variance in single cell data represents the biological variability among individual cells, which can sometimes overshadow technical noise in the dataset. This inherent variability can be both a curse and a blessing. On one hand, understanding the nuances in gene expression and cellular behavior it provides helps researchers to paint a more accurate picture of cellular functions. On the other hand, it complicates the analysis process.
A key characteristic of this variance is its unpredictable nature, which can lead to difficulties in replicating results across different studies. This makes robustness in statistical analysis critical. Rather than seeing this variance as a hindrance, seasoned researchers often embrace it as a beneficial aspect that promotes deeper inquiry into the biological questions they are tackling.
One unique feature of variance in single cell data is its capacity to reveal subpopulations of cells that may respond differently to treatments or environmental changes. The advantages of exploring this variance can lead to significant breakthroughs in understanding disease mechanisms and therapeutic responses, making it a salient focus in single cell bioinformatics.
Batch Effects
Batch effects refer to systematic differences between groups of samples that are processed under different conditions or times. In the world of single cell analysis, this can create substantial interpretation challenges. These effects can arise from various factors, including variations in reagent quality, sample handling, and even equipment differences. Consequently, these non-biological variations can obscure true biological signals, leading to erroneous conclusions.
The key characteristic of batch effects lies in their potential to manifest as consistent shifts in data that do not reflect genuine biological diversity. This can mislead researchers into incorrect interpretations and affect reproducibility across studies. The unique feature of batch effects is that they often necessitate specialized computational approaches to correct for them, ensuring that biological signals can be accurately deciphered.
In sum, while batch effects pose significant challenges to data interpretation, recognizing and correcting for them provides a clearer lens through which to view cellular data, reinforcing the scientific integrity underlying single cell research.
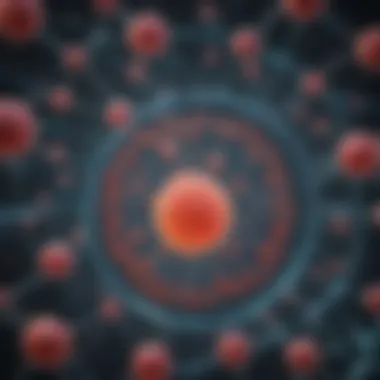
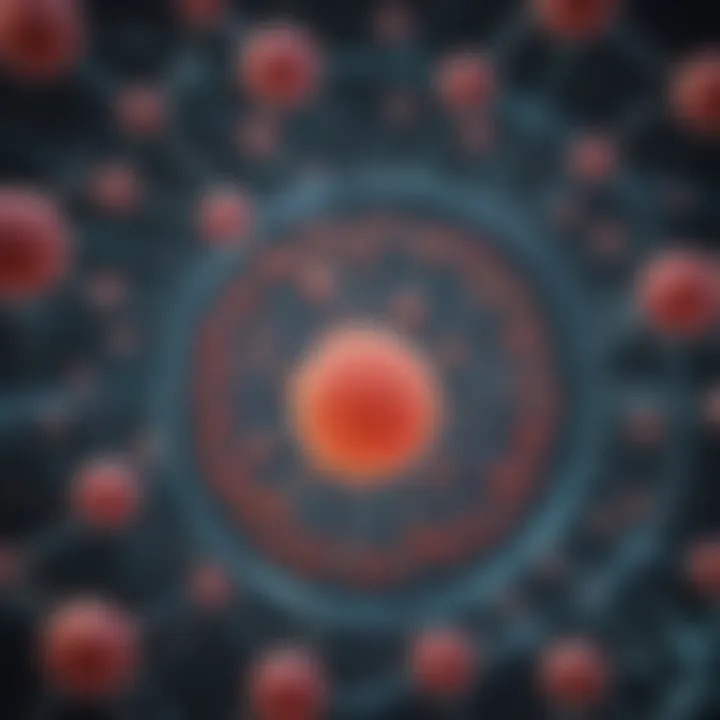
Correction techniques such as ComBat or limma are often employed to address batch effects, demonstrating that while challenges exist, the ingenuity of bioinformatics can help overcome them.
Applications of Single Cell Bioinformatics
Single cell bioinformatics plays a crucial role in our understanding of complex biological systems. It allows researchers to dissect cellular heterogeneity and capture the diverse responses of cells in various conditions. The applications of this field are vast, not only enhancing our grasp of fundamental biological processes but also paving the way for breakthroughs in medicine and therapeutics. In this section, we explore how single cell bioinformatics directly contributes to several key areas, emphasizing their importance and particular nuances.
Disease Mechanism Insights
Cancer Research
One of the standout applications of single cell bioinformatics is in cancer research. Here, it shines a spotlight on understanding tumor microenvironments and the intratumoral heterogeneity that influences treatment responses. The ability to analyze tumor cells individually opens a door to decipher how various cell populations contribute to tumor progression and drug resistance. This dissection highlights the complexity within tumors, revealing that not all cancer cells behave alike.
For instance, molecular subtyping of tumors can be fine-tuned through single cell analysis, providing insights into specific characteristics of cancer types that are otherwise obscured in bulk analyses. This precise characterization allows for more tailored therapeutic approaches. Notably, while the benefits are significant due to enhanced resolution and detail, there's a trade-off. Single cell approaches can lead to high dimensional data, making interpretation a bit tricky.
Neurobiology
Moving on to neurobiology, single cell bioinformatics has opened pathways to comprehend the nervous system's intricacies. Here, researchers can dive into the myriad of neuron types across various brain regions, unraveling how different cells contribute to overall brain function and pathology. By focusing on individual neurons or glial cells, it's possible to track their development, maturity, and responses to injury or disease.
The unique feature of single cell bioinformatics here lies in its ability to link cellular activity to behavioral outcomes. For instance, understanding how particular neuronal populations communicate can inform us on cognitive functions and disorders. That said, challenges also arise, such as the need for advanced computational tools for data analysis which can be resource-intensive.
Cellular Developmental Biology
The study of cellular developmental biology benefits immensely from single cell bioinformatics. This field focuses on lineage tracing and understanding how individual cells differentiate over time. Analyzing single cells provides insights into developmental trajectories, revealing when and how cells make fate decisions among various pathways. Understanding these processes has implications not only in stem cell research but also in regenerative medicine.
Additionally, utilizing single cell analysis helps identify key regulatory elements and external signals that guide cellular development, fostering further exploration into tissue development and organogenesis. However, the need for high-quality samples can pose challenges, as contamination or loss of cell viability can impair the data collected.
Personalized Medicine
In the realm of personalized medicine, single cell bioinformatics rediscovers patient treatments by considering cellular profiles instead of generalized biological markers. Each patientās unique cellular makeup can inform more specific treatment plans, particularly in areas like immunotherapy, where understanding the individual immune cell landscape can dictate therapy effectiveness.
The potential this application unlocks is vast, enabling precision treatments that go beyond traditional methodologies. Here, the concern often shifts to ensuring that the data is kept secure and private, as personalized approaches involve sensitive genetic information. As practices evolve, establishing robust frameworks for ethical considerations in genetic testing and treatment responses will be paramount.
"Single cell bioinformatics acts as the lens that brings our cellular world into sharp focus, making the invisible visible and actionable in research and clinical applications."
In summary, the applications of single cell bioinformatics reflect a shift toward a more detailed and nuanced understanding of life sciences. Each application embodies unique strengths and challenges, impacting therapeutic strategies, disease mechanisms, and our overarching goals in both research and clinical settings.
Challenges in Single Cell Bioinformatics
In the field of single cell bioinformatics, numerous challenges arise that can hinder the effective analysis of cellular data. Addressing these obstacles is crucial, as they not only impact data quality but also have far-reaching consequences on research outcomes. Understanding these challenges helps provide a more comprehensive view of the landscape of single cell research.
Technical Limitations
Sample Contamination
Sample contamination is a significant hurdle in single cell bioinformatics. It can stem from various sources, such as environmental factors, or during the process of handling biological samples. When contamination occurs, it can distort the data, leading to misinterpretations of cellular behavior.
The key characteristic of sample contamination is its subtlety; often, researchers may not realize that contamination is taking place until analysis reveals unexpected results. This aspect makes it a particularly tricky issue to tackle. In this article, we emphasize sample contamination because understanding its implications allows for more cautious sample collection and analysis strategies, ultimately improving data integrity.
A unique feature of this challenge is the introduction of noise in datasets. This noise can obscure genuine biological signals, complicating the journey toward reliable findings in cellular studies. The advantages of scrutinizing contamination lie in adopting better laboratory practices, such as stricter aseptic techniques. However, the disadvantage is that it demands additional time and resources during experiments, which not all institutions may afford.
Cell Identification Strategies
Cell identification strategies hold a significant role in unraveling the complexities inherent in single cell analysis. These strategies aim to accurately categorize and distinguish cells from a mixed population. The main characteristic here is the reliance on advanced computational methods and algorithms, which allow researchers to identify cell types based not only on their gene expression profiles but also other features such as spatial organization.
The reason this topic is emphasized in this article stems from the challenges linked with heterogeneous biological samples. Many diseases, especially cancers, consist of a variety of cell types, complicating identification and interpretation. The unique feature of cell identification strategies is their adaptability; they can incorporate new data as it becomes available, leading to a more dynamic understanding of cell classifications.
However, these strategies come with some disadvantages. For one, they can be computationally intensive, often requiring significant processing power and time to analyze large datasets. Additionally, misclassifications can happen if the algorithms are trained on biased datasets. Therefore, while these strategies are pivotal, they demand careful consideration in their application and development.
Computational Constraints
The computational constraints associated with single cell bioinformatics are deeply intertwined with the challenges discussed previously. As datasets scale dramatically in size and complexity, the need for powerful computational resources becomes evident. This involves substantial investments in both hardware and software, along with the skilled personnel necessary to leverage these technologies effectively.
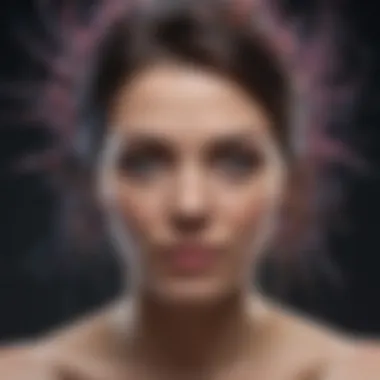
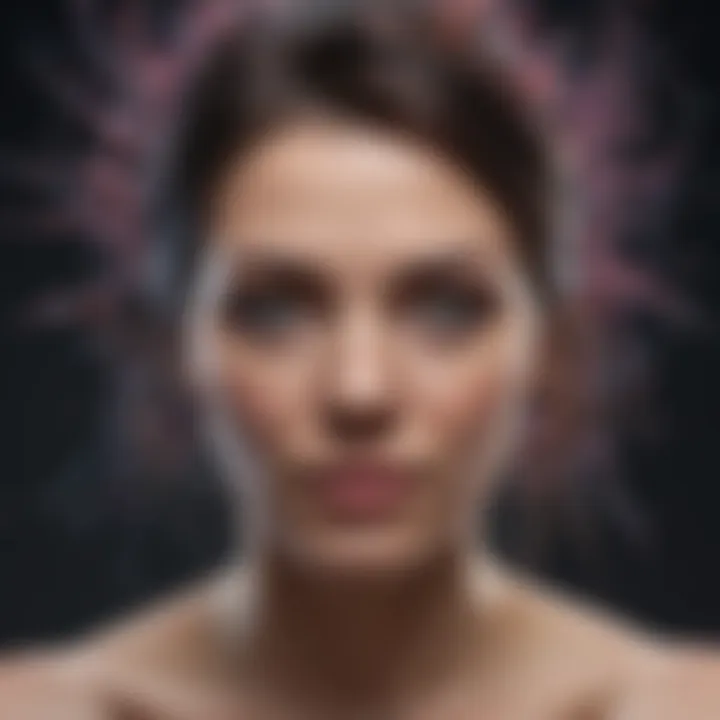
Moreover, these constraints can lead to delays in analysis, reducing the agility of research workflows. Regardless, the ongoing development of cloud computing and machine learning techniques provides encouraging avenues for overcoming these challenges. Itās essential to continually seek innovative solutions to enhance the efficiency of data processing and analysis in the ever-expanding realm of single cell bioinformatics.
Future Directions
As the realm of single cell bioinformatics continues to expand, the future directions of this field hold immense promise and relevance. It is not merely about predicting trends; it involves leveraging emerging technologies and interdisciplinary approaches to unravel the complexities inherent in biological systems. Focusing on the advancements that lie ahead is essential for researchers and practitioners eager to stay ahead in this fast-paced environment. By understanding where the trends are going, practitioners can better prepare for the potential challenges they might encounter down the road.
Emerging Technologies
Advancements in Machine Learning Techniques
Machine learning has become a game-changer in bioinformatics, especially in single cell analysis. The adaptability of these techniques allows researchers to sift through vast amounts of data, uncovering patterns that may not be visible to human analysts. This adaptability is a key characteristic, making machine learning a beneficial choice in interpreting single cell data. It allows for more accurate cell identification and classification, which is paramount for understanding complex biological processes.
One unique feature of machine learning is its ability to improve over time. As more data becomes available, algorithms can be trained to enhance their accuracy and efficiency. However, itās not without its challenges; for instance, ensuring that the datasets used are of high quality is crucial. Poor data can lead to misleading results, complicating the project instead of simplifying it.
Integration with Artificial Intelligence
The integration of artificial intelligence (AI) with single cell bioinformatics stands out as another vital area of growth. AI technologies allow for automated analysis, enabling researchers to spend more time on experimental design and interpretation rather than on data processing. This not only boosts productivity but also accelerates discovery.
A significant aspect of AI is its capability to manage unstructured data, which is increasingly vital in a world where experimental outputs can vary immensely. This unique feature of AI can enhance the understanding of cellular functions and interactions, which plays a pivotal role in various applications, from cancer therapies to regenerative medicine. However, the complexity of AI algorithms can also pose a challenge, as researchers must ensure they understand how these systems operate to interpret their findings accurately.
Interdisciplinary Approaches
Emphasizing interdisciplinary approaches involves drawing expertise from various fields, such as genomics, data science, and computational biology. Collaborations among scientists, statisticians, and computer engineers can drive innovation in methodologies and analytical frameworks.
"The intersection of multiple disciplines will likely give rise to breakthroughs that redefine our understanding of biology at the cellular level."
This approach not only fosters creativity but also enhances the precision of research outcomes. As we venture into the future, it is critical that researchers engage with technologically adept individuals outside their comfort zones. By combining different skill sets, the scientific community can tackle tough questions and drive forward the field of single cell bioinformatics that values complexity, accuracy, and innovation.
Ethical Considerations
In the rapidly evolving domain of single cell bioinformatics, ethical considerations are not just an afterthought; they are foundational to the integrity and advancement of research. As scientists probe deeper into the individual cellular makeup, understanding the ramifications that arise in terms of ethics becomes crucial. This section sheds light on two pivotal areas: data privacy and security, and the implications these technologies hold for genetic research.
Data Privacy and Security
The crux of single cell bioinformatics rests on handling vast troves of data, often tied to individual patients or specific cell lines. As data privacy issues come to the forefront, researchers must navigate this complex landscape with care. The information collected can include sensitive genetic details that, if mishandled, pose significant risks to individuals' privacy. With established regulations like the General Data Protection Regulation (GDPR) in Europe, researchers are obliged to ensure that data is anonymized, secure, and used responsibly.
Concerns about data breaches loom large; a single lapse in protection measures could expose identities and genetic information to unauthorized entities.
- Key Considerations for Data Privacy:
- Informed Consent: Researchers must secure informed consent from all participants. This ensures that individuals understand how their data will be used, stored, and potentially shared.
- Data Anonymization: Stripping identifiable details can help mitigate the risks associated with data breaches.
- Secure Storage Solutions: Advanced encryption methods and secure storage practices are essential to safeguarding data from potential breaches.
Implications for Genetic Research
As single cell bioinformatics intersects with genetic research, the ethical landscape grows even more intricate. The ability to analyze genetic information on a cell-by-cell basis opens doors to revolutionary insights but also raises ethical concerns regarding the potential misuse of data.
Researchers must grapple with several important implications, especially concerning genetic modifications and the prospect of gene editing technologies like CRISPR. The ethical questions swirl:
- Potential for Misuse: Thereās a slippery slope regarding how genetic data could be used, especially if it finds itself in the wrong hands. Would it be exploited for discrimination in employment or insurance?
- Ownership of Genetic Data: Who owns the data? The individual, the research institution, or the funding body? The nuances surrounding ownership remain a contentious debate in bioethics.
"The collection of genetic data is a double-edged swordāit can illuminate the structure of life but can also lead down a perilous path if not handled ethically."
Epilogue
The conclusion of this article serves as a pivotal synthesis of the intricate themes discussed throughout, emphasizing the profound importance of single cell bioinformatics in modern biological research. As we've traversed various methodologies, applications, and challenges, the narrative underlines how this field transcends traditional biological paradigms by aiming for a granulated understanding of cellular behavior. The significance of such depth cannot be overstated, as it leads to significant advances in health, drug development, and personalized medicine.
Summary of Key Insights
In analyzing single cell bioinformatics, several pivotal insights emerge:
- Nuanced Understanding: Individual cell analysis allows for capturing the idiosyncrasies that population-level data tends to mask. This granular approach elucidates variances in cellular responses, paving the way for targeted interventions.
- Technological Evolution: The rapid advancements in sequencing technology have created opportunities for unprecedented data generation. Platforms such as 10X Genomics have revolutionized how we approach cellular analysis.
- Integration of Disciplines: As this field continues to grow, the intersection with artificial intelligence and machine learning becomes ever more crucial. It allows researchers to handle complex datasets, fostering a more intuitive analysis of biological phenomena.
- Ethical Considerations: With this powerful data comes the need for responsible data management and the ethical implications tied to genetic information. Protecting privacy while leveraging data for research is a balancing act that must remain at the forefront of discussions.
The Road Ahead in Single Cell Bioinformatics
Looking forward, the future of single cell bioinformatics is rife with potential:
- Advancements in Data Interpretation: Continued development in algorithms and analytical frameworks will refine data interpretation, increasingly unveiling the intricacies of cellular functions.
- Emerging Technologies: As technologies such as CRISPR and advanced imaging techniques evolve, their integration with single cell bioinformatics will unlock new avenues for research. This could lead not only to greater depth of study but also to expansive applications across various fields including regenerative medicine and oncology.
- Collaborative Efforts: Interdisciplinary partnerships between biologists, data scientists, and ethicists will be vital. These collaborations promise to foster a robust framework for navigating future challenges while ensuring the responsible use of powerful new tools.
āThe question isnāt whether individual cells matter, but rather, how can we harness their secrets to cultivate breakthroughs in health?ā