Understanding Multi-Factor Risk Models in Finance
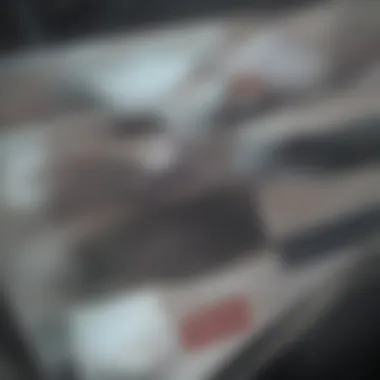
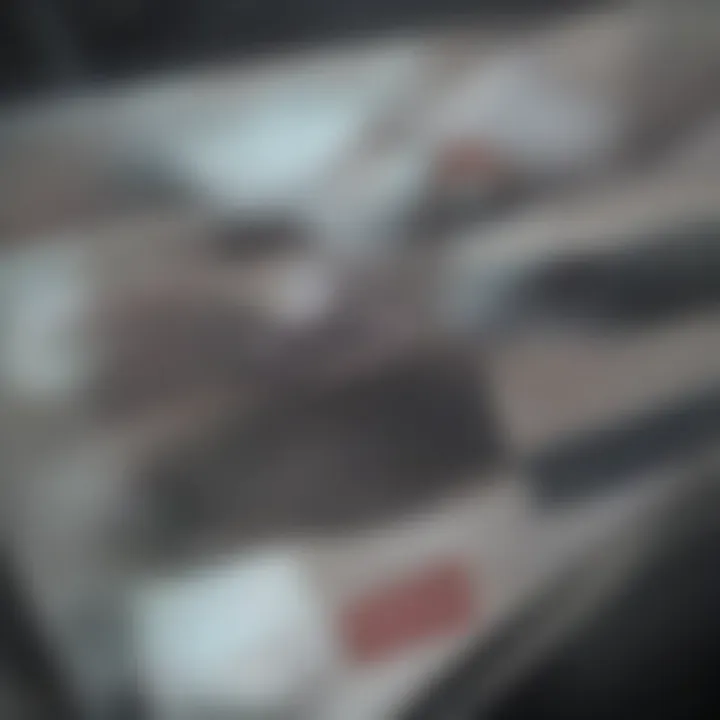
Intro
In the intricate landscape of finance, understanding risk is essential for sound decision-making. Multi-factor risk models play a pivotal role in this context, offering a structured approach to evaluate risks associated with various investment portfolios. This article aims to present a comprehensive guide on multi-factor risk models, outlining their principles, construction methods, applications, and challenges encountered during implementation.
By analyzing the components of these models, we can uncover insights that significantly influence portfolio management. As investors seek to optimize returns while managing potential losses, grasping the intricacies of multi-factor risk assessment becomes increasingly vital. A thorough comprehension of these models allows financial professionals to assess market behaviors, evaluate strategies, and ultimately devise informed investment plans.
Research Context
Background and Rationale
The evolution of financial markets has underscored the importance of advanced risk assessment tools. As portfolios grow in complexity, traditional single-factor models often fall short. Multi-factor risk models emerged from the need to incorporate various sources of risk that affect asset prices beyond just market movements. By utilizing multiple factors, such as size, value, and momentum, these models enhance the predictive power regarding asset returns, providing a more holistic view of risk exposure.
Literature Review
Previous research has highlighted the effectiveness of multi-factor models in portfolio construction and risk management. Notable works by Fama and French established the basis for many multi-factor approaches currently employed. The concept of augmenting traditional Capital Asset Pricing Model (CAPM) with additional factors laid the groundwork for further innovations. Consequently, the proliferation of academic papers has enriched the understanding of these models, leading to their widespread adoption in both academia and industry.
Methodology
Research Design
This article employs a qualitative approach, synthesizing existing literature and theoretical frameworks surrounding multi-factor risk models. By concentrating on prominent theories and methodologies, practitioners and scholars can develop a clear understanding of how these models operate within practical contexts.
Data Collection Methods
Data for this article draws from various academic journals, industry reports, and authoritative financial sources. Statistical analyses and empirical findings have been integrated to substantiate claims and provide a well-rounded perspective on multi-factor risk models. The review of diverse literature allows the identification of common themes and patterns that constitute the foundation of multi-factor risk assessment.
Multi-factor risk models offer a structured approach that integrates diverse risk factors, thereby enhancing risk assessment and portfolio optimization.
As we progress through this analysis, the subsequent sections will uncover specific approaches to building these models, the essential factors to consider, and potential challenges that professionals may face in their implementation and practical use.
Prolusion to Multi-Factor Risk Models
Multi-factor risk models represent a critical framework in the realm of financial analysis, shaping how investors understand and navigate the complexities of asset pricing and risk management. In these times of market volatility and unpredictability, the ability to dissect and comprehend the nuances of various risk factors is essential for anyone engaged in finance. This section serves as both an introduction to the topic and a basis for understanding the subsequent discussions regarding multi-factor models.
Defining Multi-Factor Models
Multi-factor models are analytical tools that aim to explain the relationship between multiple independent variables (factors) and a dependent variable (like asset returns). Unlike single-factor models, which focus on a lone variable, multi-factor models account for diverse influences that can affect the performance of a given asset. The concept finds its roots in the Capital Asset Pricing Model (CAPM), which traditionally considers only market risk. However, it quickly became apparent that other factors, such as size, value, and momentum, could also significantly contribute to returns.
By incorporating these variables, multi-factor models enhance the explanatory power and predictive capability regarding asset returns. Some well-known frameworks include the Fama-French three-factor model, which adds size and value to market risk, and the Carhart four-factor model that further factors in momentum. This expansion allows analysts and investors to capture a wider spectrum of risk that can lead to better performance forecasting.
Importance in Financial Analysis
The relevance of multi-factor models in financial analysis cannot be understated. They facilitate a more nuanced understanding of the risks associated with investments. By assessing various factors, investors can optimize their portfolios, minimize unwanted risks, and effectively strategize their asset allocations.
Here are several key reasons why they are important:
- Risk Diversification: Incorporating multiple factors opens avenues for diversification, reducing the dependency on any single risk element.
- Enhanced Predictability: Multi-factor models provide improved predictions of asset return behavior, contributing to more informed decision-making.
- Performance Attribution: These models enable analysts to decompose portfolio returns accurately, attributing performance to specific factors rather than general market movements.
- Adaptability: Multi-factor models can easily incorporate newly identified risk factors, allowing flexibility in an ever-evolving market landscape.
The utility of multi-factor risk models extends beyond theoretical applications; they are indispensable tools for analysts and practitioners aiming to navigate the intricacies of financial markets.
Historical Context and Development
The historical context of multi-factor risk models offers insight into how financial analysis has evolved over time. Understanding this evolution provides clarity on the necessity and effectiveness of multi-factor models in contemporary finance. Risk modelling itself has undergone significant transformations, influenced by advances in technology, data availability, and economic theories.
Evolution of Risk Modelling
The practice of risk modelling began long before the creation of modern financial instruments. Early theories focused on the principles of probability and statistics, but these were primarily employed in simplistic forms. As financial markets grew more complex, so did the methods used to assess risk.
The introduction of the Capital Asset Pricing Model (CAPM) in the 1960s marked a significant milestone in risk modelling. This single-factor model proposed a linear relationship between the expected return of an asset and its systematic risk, as measured by beta. While revolutionary, CAPM had limitations. It oversimplified the dynamics of financial markets by ignoring other potential sources of risk.
In the late 1980s and early 1990s, the multi-factor approach gained traction. Researchers like Eugene Fama and Kenneth French expanded upon CAPM by introducing additional factors that influence returns. The Three-Factor Model, for instance, incorporated size and value as factors besides market risk. This shift reflected a more nuanced understanding of risk and its components, updating conventional wisdom in finance.
Furthermore, with computer technology advancements, it became feasible to analyze large datasets. This allowed financial analysts to incorporate various economic factors into their models. The ability to identify numerous influences on asset prices intensified interest in more sophisticated multi-factor approaches, ultimately leading to improved risk assessments and investment strategies.
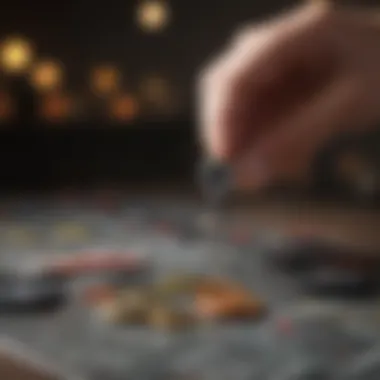
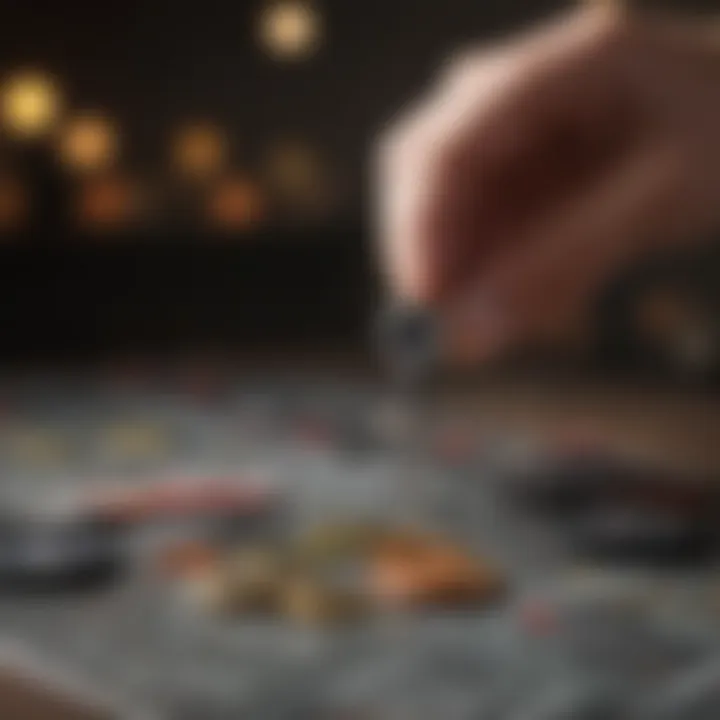
Key Theoretical Foundations
Several theoretical foundations underpin the development of multi-factor risk models. One is the Arbitrage Pricing Theory (APT), proposed by Stephen Ross in the 1970s. APT posits that the return on an asset can be predicted using a linear function of various macroeconomic factors, allowing for a more flexible approach than CAPM. This theory laid groundwork for understanding multiple influences on asset pricing.
Another cornerstone is the efficient market hypothesis, which asserts that asset prices reflect all available information. This theory supports the need for models that account for different risk factors, as most investors are unlikely to exploit all information effectively, creating opportunities in the market.
"The examination of different risk factors enhances predictive power in investment strategies, providing a buffer against market anomalies."
Furthermore, behavioral finance has gained prominence in recent years, illustrating how psychological factors affect investor decisions. This consideration enables models to encapsulate not only statistical relationships but also psychological barriers to efficient decision-making. Incorporating behavioral insights into multi-factor risk models has extended their applicability and relevance.
In summary, the historical development of risk modelling from simple statistical approaches to robust multi-factor risk frameworks reflects a broader understanding of financial markets. This progression illuminates the increasing complexity of investment environments and the critical need for comprehensive risk assessment methodologies.
Core Components of Multi-Factor Risk Models
The core components of multi-factor risk models are essential for understanding how these models function in financial analysis. These components provide structure to the models, allowing for more nuanced risk assessments and enhanced decision-making. Their importance cannot be understated; they serve as the foundational elements that facilitate the identification, measurement, and management of risk. A comprehensive grasp of these components ultimately guides practitioners in applying these models effectively within the investment landscape.
Factors Identified in Models
Multi-factor risk models hinge primarily on the factors selected to explain asset returns and associated risks. The factors can vary widely, often encompassing:
- Economic factors: These include variables like GDP growth, inflation rates, and interest rates. They reflect broader economic conditions that substantively influence market performance.
- Market factors: Commonly referenced are the market returns that may affect individual assets. This align with the overall movements in the market, usually represented by indices.
- Fundamental factors: These involve financial characteristics of the securities, such as earnings or book value. They offer insights into company performance and potential future returns.
- Industry-specific factors: Certain industries may respond differently to economic changes, making it essential to identify factors relevant to specific sectors.
Selecting the appropriate factors necessitates a thorough analysis of their historical performance and relevance to the portfolio being modeled. A careful selection can significantly enhance the model's ability to predict returns and manage risks.
Risk Exposure and Return
Understanding risk exposure and return is central to the application of multi-factor risk models. Risk exposure refers to the degree to which an investment is affected by changes in underlying factors. This exposure is critical for estimating potential losses if adverse changes occur.
Return, on the other hand, signifies the expected gain from an investment, taking into account the risk associated with those investments. Investors seek to optimize their portfolios by balancing risk and return, ensuring that the expected return compensates adequately for the associated risk.
Collectively, risk exposure and return enable investors to:
- Assess performance: By analyzing returns relative to exposures, investors can determine how effectively a portfolio manages risks while achieving desired outcomes.
- Diversify strategies: Different factors can behave differently under various market conditions. Understanding these relationships allows for diversification, mitigating risks while enhancing potential returns.
- Make informed decisions: A nuanced understanding of risk-return profiles enables better strategic choices in investment positions.
"The balance of risk and return highlights the fragile interplay that defines market dynamics and portfolio performance."
In summary, the core components of multi-factor risk models are vital for effective risk management and portfolio optimization. A deep understanding of identified factors and their impact on risk exposure and return empowers finance professionals to make informed decisions and strategically allocate resources.
Methodologies for Model Construction
The construction of multi-factor risk models is a crucial aspect of financial analysis. This section aims to unpack the methodologies involved. By understanding these methodologies, finance professionals can make informed decisions on factor selection, model design, and implementation.
Selecting Relevant Factors
Selecting factors is essential to ensure that a model captures the most significant risks affecting portfolio returns. Factors can stem from diverse sources, including economic indicators, market trends, or specific asset behaviors. The chosen factors ought to have a theoretical foundation, closely linked to financial outcomes.
- Theoretical Rationale: Each selected factor must have sound theoretical justification. For instance, economic growth often drives stock returns, thus making GDP growth a relevant factor.
- Empirical Relevance: Factors should also be backed by empirical evidence. Statistical tests can validate their effectiveness in predicting returns.
- Diversity of Factors: A diverse set of factors enhances model robustness. Including both systematic and idiosyncratic factors can capture various risk aspects.
While selecting factors, practitioners should avoid over-complication. It is crucial to strike a balance between comprehensiveness and simplicity. Selecting too many factors may lead to clutter, reducing clarity. This may hurt the model's explanatory power and increase the risk of overfitting.
Statistical Techniques
Once the factors have been selected, appropriate statistical techniques are vital for constructing an effective model. These techniques help in estimating the relationships between factors and asset returns.
- Regression Analysis: Ordinary Least Squares (OLS) regression remains a widely used technique for modeling relationships. It estimates the impact of each factor on returns, resulting in a clearer understanding of their significance.
- Principal Component Analysis (PCA): PCA can simplify the modeling process. It reduces the dimensions of data while retaining essential variance. This technique aids in identifying common factors that influence multiple assets, effectively reducing complexity.
- Time Series Analysis: Incorporating time series methods allows analysts to account for temporal effects. Understanding how factors change over time can lead to more accurate predictions.
- Machine Learning Approaches: In recent years, machine learning has started to intersect with finance. Techniques like random forests and support vector machines can capture non-linear relationships between factors and asset returns.
Choosing the right statistical technique often depends on the model's objectives and the nature of the data. It is important to rigorously test the model's validity through back-testing and cross-validation methods to ensure reliable predictions.
In summary, methodologies for model construction are foundational to the effective use of multi-factor risk models. Careful factor selection combined with robust statistical techniques facilitates better understanding and risk management in finance.
By mastering these methodologies, finance professionals can build models that not only assess risks effectively but also contribute to improved portfolio performance.
Data Considerations in Multi-Factor Models
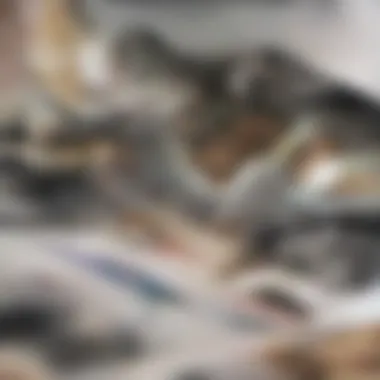
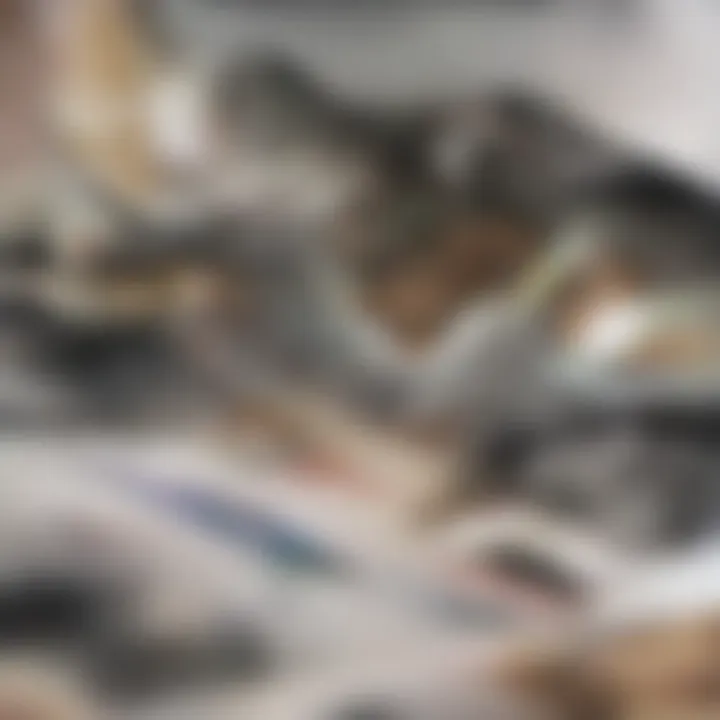
The validity and robustness of multi-factor risk models fundamentally depend on the quality of the data utilized. Effective data consideration comprises the collection, processing, and handling of data that correlates to the factors used in defining the models. Data quality impacts the reliability of the results generated. Therefore, understanding the sources of data and how to address issues such as missing data is crucial for practitioners in the finance sector.
Data Sources and Quality
Identifying diverse and credible data sources forms a pivotal point in constructing multi-factor risk models. Primary sources often include financial statements, market data, and statistical reports. Secondary sources like academic publications, research organizations, and financial news can also contribute valuable information.
The reliability of data can significantly influence the conclusions drawn from the models. Inconsistent data, outdated information, or errors can lead to misleading analyses. Hence, practitioners should focus on the following factors when evaluating data quality:
- Relevance: The data must directly relate to the factors being analyzed.
- Timeliness: Current data reflects the market's changing conditions.
- Accuracy: Errors in data can lead to flawed risk assessments.
- Completeness: It is essential to gather comprehensive data to fully understand the underlying factors.
When high-quality data is used, the model outcomes become more trustworthy, thus enhancing the decision-making process.
Handling Missing Data
Missing data is a common challenge that can significantly alter the performance of multi-factor models. Ignoring missing values is not an option, as this may introduce bias and lower the model effectiveness. Therefore, several strategies can be implemented to handle this issue:
- Imputation: This method involves filling in missing data with estimated values based on surrounding information. Popular techniques include mean, median, or mode substitution, as well as more advanced methods like regression or k-nearest neighbors.
- Data Deletion: In some cases, entire rows or columns may be removed from the analysis. While this makes the dataset cleaner, it can lead to loss of important information.
- Indicator Variables: Creating indicator variables to signify missing data points can help in assessing the impact of having incomplete data.
It is vital to examine the pattern of missing data before selecting a strategy. A thoughtful approach to missing data keeps the integrity of the model intact.
"Data is a precious thing and will last longer than the systems themselves." - Tim Berners-Lee
Applications of Multi-Factor Risk Models
The application of multi-factor risk models is crucial in modern finance. These models serve a range of purposes across various phases of investment and financial analysis. They help not only in assessing risk but also in informing strategies that can mitigate those risks effectively. Strong applications of these models can lead to better portfolio management and investors' decision-making capabilities. Understanding where and how these models can be applied equips finance professionals with valuable tools to navigate complex markets.
Portfolio Management Strategies
Utilizing multi-factor risk models in portfolio management offers several advantages. These models allow managers to understand the underlying factors that drive returns within an investment portfolio. By identifying the factors that significantly impact asset prices, managers can optimize asset allocation according to risk and return profiles. For example, a portfolio manager might use a multi-factor model to evaluate how sensitive their portfolio is to economic growth rates, interest rate changes, and various market sentiments.
- Diversification: Having a well-constructed multi-factor model encourages better diversification. Instead of relying on a single risk factor, managers can spread investments across several factors, which reduces the overall risk.
- Performance Attribution: These models facilitate performance attribution analysis. By deconstructing returns into contributions from various factors, managers can analyze which elements are adding value and which are detracting from performance.
- Risk/Return Optimization: Multi-factor models assist in optimizing risk and return trade-offs. Managers can simulate different portfolio construction scenarios based on scenarios derived from the model, providing insight into risk exposure aligned with investor objectives.
Risk Assessment Practices
Risk assessment is another vital application of multi-factor risk models. They provide frameworks for quantifying risks associated with various investment choices. These models can accommodate multiple dimensions of risk, which is essential given the evolving financial landscape.
- Comprehensive Risk Metrics: Multi-factor models generate a variety of risk metrics, allowing analysts to evaluate a wide spectrum of risk exposure. They help in understanding how different economic scenarios affect asset valuations.
- Scenario Analysis: Investment professionals can employ multi-factor risk models to conduct scenario analyses. Testing how portfolios respond to different economic conditions can offer insights and assist in preparing for adverse situations.
- Stress Testing: Many firms incorporate these models into stress testing exercises. By assessing how portfolios behave under extreme conditions, investors can make informed decisions on risk management strategies.
In essence, integrating multi-factor models into risk assessment practices enhances the predictability of risk outcomes. This leads to informed strategic decisions that blend well with overall financial planning.
"Analyzing risk through multi-factor frameworks significantly sharpens the lens through which investors assess market uncertainties."
The applications of multi-factor risk models are expansive and multifaceted. They integrate a valuable approach that bolsters both portfolio management strategies and risk assessment practices. Engaging deeply with these applications can transform the investment landscape and refine how risks are understood and managed.
Challenges in Implementing Multi-Factor Models
Implementing multi-factor models in finance is not just a matter of constructing algorithms and analyzing data. It involves navigating complex challenges that can significantly affect the robustness and reliability of the model's outcomes. These challenges stem from the intricacies of financial data, the theoretical assumptions, and more importantly, the dynamic nature of financial markets.
To fully understand the relevance of these challenges, one must first recognize the environment in which multi-factor models operate. They aim to capture the relationship between various macroeconomic variables and asset returns. However, discrepancies in model construction and market behaviors can lead to substantial pitfalls. Successful implementation not only increases the predictive power of financial analyses but also aids in better portfolio management strategies and enhances risk assessment practices. Neglecting these challenges can lead to model failure, financial loss, or misguided investment decisions, thereby mandating that practitioners remain vigilant and informed about the issues at hand.
Model Overfitting
Model overfitting occurs when a multi-factor model becomes excessively complex, fitting not only the historical data but also the noise inherent in that data. An overfitted model typically performs well on past datasets but poorly predicts future outcomes. This is a critical challenge that often arises from a poor balance between model complexity and data adequacy.
When practitioners include too many factors or employ overly sophisticated statistical techniques without sufficient justification, they risk creating models that do not generalize well. One approach to mitigate overfitting is to use simpler models or reduce the number of factors considered. Alternatives such as cross-validation can also provide insights into a model's potential performance on unseen data.
Adaptive Market Hypothesis
The adaptive market hypothesis presents another layer of complexity in the implementation of multi-factor models. Traditional financial theories often presume that markets are efficient and that all available information is already reflected in asset prices. However, the adaptive market hypothesis introduces a more dynamic view of market behavior, suggesting that participants adapt their strategies based on the evolving nature of market conditions.
As investors modify their behaviors in response to changing environments, the factors that drive asset prices can shift. This necessitates flexibility in model construction and an understanding that factors deemed significant today may become irrelevant in the future. Thus, practitioners must remain agile, regularly revisiting their models to ensure they adequately reflect current market dynamics.
Ultimately, while multi-factor models offer significant advantages in risk assessments and investment strategies, addressing the challenges of model overfitting and the adaptive market hypothesis is crucial for their practical application. Without such considerations, one may risk operating under illusions of predictability in fundamentally unpredictable markets.

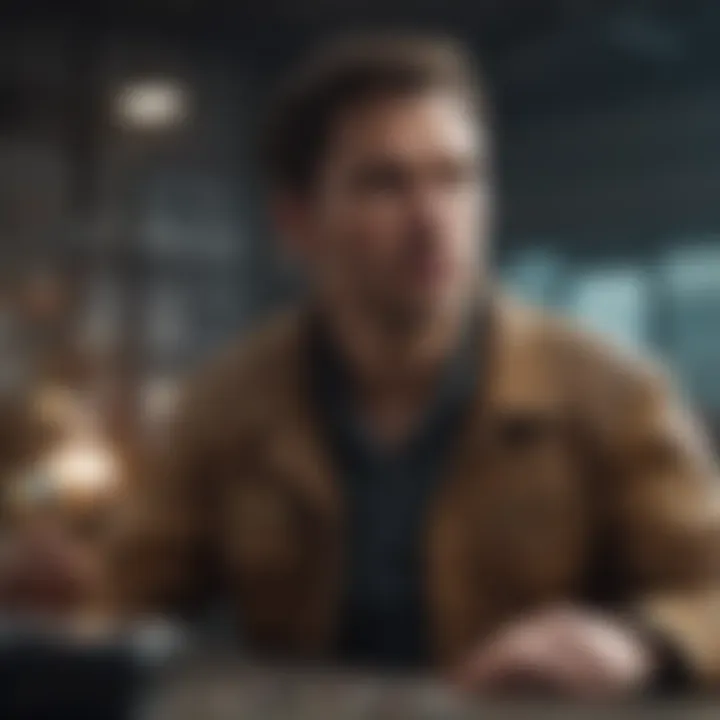
Comparative Analysis of Multi-Factor Models
The comparative analysis of multi-factor models is a crucial aspect of understanding the efficacy and application of these models in finance. It allows practitioners to assess strengths and limitations by examining multi-factor approaches against other modeling techniques, particularly single-factor and hybrid models. This analysis identifies conditions under which each model may be most effective, providing insights that enhance investment strategies and risk assessment practices.
Versus Single-Factor Models
Single-factor models, typically represented by the Capital Asset Pricing Model (CAPM), focus on a single source of risk, which generally simplifies analysis but limits its applicability in a complex financial landscape. By contrast, multi-factor models incorporate various factors to explain asset returns and better account for the nuances of market behavior.
The comparative efficacy emerges notably in risk measurement. Multi-factor models are adept at capturing the variability of returns linked with multiple influences, such as macroeconomic factors and specific asset characteristics. In scenarios depicting market volatility or structural changes, multi-factor models often outperform single-factor designs due to their comprehensive nature.
Some key benefits of multi-factor models over single-factor models include:
- Risk diversification: They provide a more granular understanding of factors driving returns.
- Improved accuracy: More data points lead to enhanced predictive capabilities.
- Flexibility: They allow for adjustments based on changes in market conditions.
It is crucial to note that the simplicity of single-factor models can be alluring, especially for less complex portfolios. However, the richness of information in multi-factor systems is often justified by better performance in diverse scenarios.
Hybrid Models
Hybrid models present an interesting middle ground between single-factor and multi-factor approaches. They bring together various elements, aiming to capture the benefits of both methodologies. This flexibility allows for a tailored application based on specific investment goals or market environments.
Hybrid models often incorporate multi-factor analysis within a single-factor framework. For instance, a researcher may start with a single-factor model to establish a baseline risk, and then refine their analysis through the addition of factors pertinent to the asset class or portfolio.
Key considerations for understanding hybrid models include:
- Integration of diverse factors: Use of both micro and macroeconomic indicators can enrich analysis.
- Dynamic adjustments: They allow for modifications as market conditions evolve, maintaining relevance.
- Complexity management: While multifactor models can be complex, hybrid models can simplify by focusing on key factors relevant to specific contexts.
Future Trends and Innovations
The realm of multi-factor risk models is experiencing rapid evolution, influenced by advancements in technology and changes in market dynamics. This section highlights critical future trends and innovations relevant to the application of multi-factor risk models in finance. Understanding these trends can provide practitioners and researchers with strategic insights, enhancing their analytical capabilities and decision-making processes.
Technological Advancements
Technological advancements are reshaping the landscape of multi-factor risk models. The integration of high-frequency trading systems and real-time data feeds allows for more dynamic risk assessment. The capacity to process vast amounts of data quickly and accurately has become essential in identifying and analyzing the factors driving market behavior.
The use of cloud computing enables finance professionals to scale their modeling efforts without the constraints of on-premises computing power. Increased computational resources facilitate complex simulations and back-testing of models, enhancing the reliability of risk assessments.
Moreover, improved data visualization tools allow analysts to present findings and insights in more accessible formats. These tools can help summarize complex relationships between different factors in an intuitive way, aiding communication and understanding among stakeholders.
Integrating Machine Learning Techniques
Integrating machine learning techniques into multi-factor risk models offers numerous benefits. Machine learning algorithms are capable of detecting patterns within large data sets that traditional methods may overlook. This capability enhances factor selection and can significantly improve the accuracy of risk predictions.
Furthermore, machine learning can adapt to the changing nature of financial markets. As new data becomes available, these models can update themselves, ensuring that they reflect current trends and behaviors. This adaptability is crucial in an ever-evolving market landscape, where investors need to be responseive to new information.
Additionally, the application of unsupervised learning methods can assist in identifying new factors that were previously considered non-essential. These factors may hold significant value in risk models, providing a more comprehensive view of potential risks.
Closure and Implications
The conclusion of an article on multi-factor risk models serves a critical role in weaving together the various threads presented throughout the discussion. This section emphasizes the necessity of recognizing the significance of these models in financial risk assessment and portfolio management. Multi-factor risk models are not just tools for analysis; they provide a framework for understanding the complexities of market behavior and the multitude of factors influencing asset returns.
In summarizing the key insights garnered from previous sections, it becomes evident that multi-factor models extend beyond the traditional frameworks, integrating a broader range of influences on risk and return. Practitioners and scholars alike benefit from a comprehensive grasp of these insights, which offer a clearer picture of investment dynamics. The adaptation of these models allows for more informed decision-making, ultimately leading to enhanced investment strategies.
Summarizing Key Insights
Through the analysis provided, several pivotal insights emerge regarding multi-factor risk models:
- Diversity of Factors: The models highlight that a variety of factors—ranging from macroeconomic indicators to industry-specific influences—play critical roles in predicting asset performance.
- Enhanced Risk Evaluation: By employing multi-factor models, investors can better understand their exposure to various risk factors, allowing for more strategic allocation of assets.
- Model Construction: The construction of these models requires careful selection of relevant factors, backed by statistical methodologies that ensure robustness in predictions.
- Forward-Looking Assessment: With ongoing developments in technology and data analytics, multi-factor models are becoming increasingly sophisticated, incorporating machine learning techniques for predictive accuracy.
In framing these insights, the article reaffirms the assertion that multi-factor risk models significantly improve the analytical capabilities of investors and risk managers.
Implications for Practitioners
The implications for practitioners are profound and multifaceted. Understanding and implementing multi-factor risk models can yield several benefits:
- Informed Investment Strategies: Professionals equipped with the insights from these models can devise more resilient investment strategies, fostering better portfolio performance.
- Risk Mitigation: By recognizing the specific risk exposures inherent in their portfolios, investors can take proactive steps to mitigate potential losses.
- Regulatory Compliance: As regulations surrounding transparency and risk management evolve, proficiency in multi-factor models will aid in addressing compliance mandates effectively.
- Long-Term Performance Assessment: The integration of multi-factor risk assessment into regular evaluations enables a more consistent analysis of portfolio performance over time.
"Multi-factor risk models shift the focus from conventional, simplistic approaches to a more nuanced understanding of risk and return dynamics."
These implications remind practitioners that as financial landscapes change, the adoption of comprehensive analytical frameworks like multi-factor risk models is not optional but essential for success in today's competitive markets.