Understanding RMS: A Comprehensive Exploration
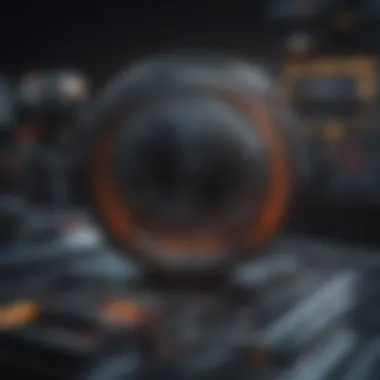
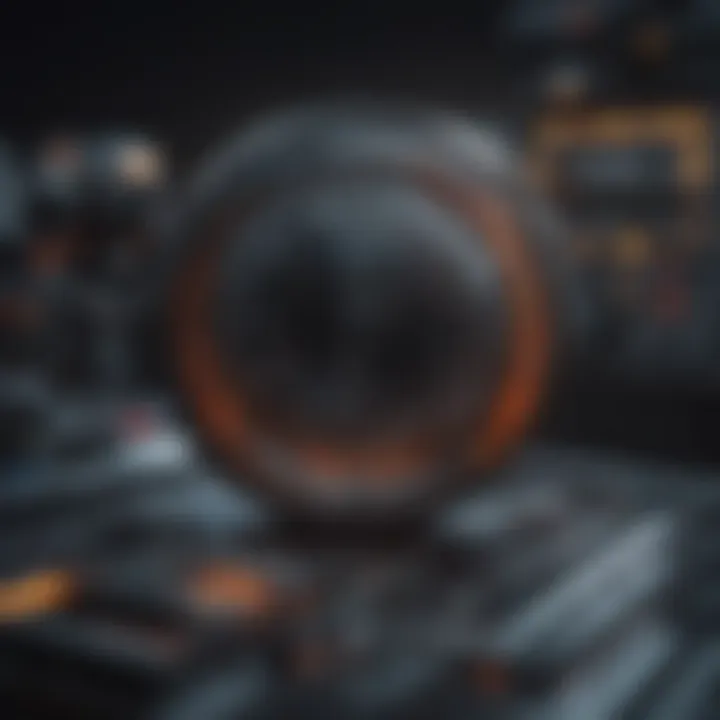
Intro
In various fields, the term RMS often emerges as a cornerstone concept. Understanding its implications provides essential insights into more complex subjects across mathematics, engineering, and statistics. RMS, or root mean square, serves as a crucial tool in data analysis and signal processing. As we delve into this exploration, it is vital to grasp the foundational aspects as well as the nuances that make RMS significant.
The breadth of RMS applications is vast, extending from electrical engineering, where it assists in analyzing alternating currents, to data analysis techniques that refine our understanding of datasets. Thus, this examination aims to clarify the diverse meanings, applications, and impact of RMS in both theoretical and practical lenses, catering to students, professionals, and researchers alike.
Prelude to RMS
In the study of electrical systems, statistics, and various engineering disciplines, the term RMS, short for Root Mean Square, emerges as a pivotal concept. Its significance is not just theoretical; it has practical implications that affect designs, calculations, and methodologies in diverse fields. Understanding RMS is crucial for effectively analyzing performance, maximising efficiency and ensuring reliable systems in complex applications.
Definition of RMS
Root Mean Square is a statistical measure of the magnitude of a varying quantity. It is especially valuable for assessing the average power of an alternating current (AC) signal. The formula calculates the square root of the mean of the squares of the values. This method provides a way to equate fluctuating signals to a steady direct current (DC) value for practical purposes. In mathematical terms, if we denote a function by f(t), the RMS value over a period T is defined as:
RMS is often employed in scenarios where the fluctuations of a signal need to be accurately portrayed. This would include applications in electrical engineering for AC waveforms, statistical analysis for data sets, and other fields requiring precise calculations of average values.
Historical Context
The concept of RMS has origins that can be traced back to the needs of early engineers and mathematicians. In the 18th century, the burgeoning field of electrical engineering started documenting the behavior of AC systems. The RMS method notably gained traction in the late 19th century, when scientists and engineers began to develop a formal understanding of alternating currents and the fundamental principles of electricity. This historical backdrop is essential, as it reveals how RMS evolved from an abstract mathematical construct to a critical tool in technology today.
The pioneering works conducted by figures such as James Clerk Maxwell further advanced the understanding of RMS by connecting it with the statistical properties of waves. The method has expanded over time, now finding usage beyond engineering, in domains like finance, signal processing, and risk assessment. Its robust framework enables professionals to navigate and interpret oscillations and irregularities in data effectively.
Understanding RMS allows for a unified approach to solving diverse problems across different realms, reinforcing its importance in both the historical and modern contexts.
Mathematical Foundation of RMS
The mathematical foundation of RMS (Root Mean Square) is critical in understanding how this concept applies across various fields. RMS serves as a powerful tool because it provides a form of average that is especially useful for evaluating fluctuating signals, measurements, and even data sets. Understanding the mathematical underpinnings ensures accurate application in engineering, finance, and data analysis.
In essence, RMS is valuable because it allows for consistent comparison across different data types. It effectively considers both the magnitude and frequency of values. This approach is beneficial when assessing energy or power related to alternating current (AC), emphasizing the necessity of accurate calculations for optimal performance.
Formula Derivation
To derive the RMS formula involves several steps. The formula for RMS is expressed as:
Where N is the total number of values, and xᵢ represents each individual value in the set. This expression effectively captures the average of the squares of the values, followed by taking the square root of that average. This method of calculation enhances the significance of larger values, which is essential in many practical applications.
Calculation Steps
Example Problems
Example problems involve simple to complex scenarios that illustrate RMS calculations in real contexts. For instance, consider a set of values from a current measurement in an electrical circuit. If the values are 3, 4, and 5, the RMS can be calculated as follows:
- Square each value: 3² = 9, 4² = 16, 5² = 25.
- Compute the average of these squared values: (9 + 16 + 25) / 3 = 50 / 3 = 16.67.
- Finally, take the square root: √16.67 ≈ 4.08.
This systematic approach shows how RMS assists in achieving an effective generalization of fluctuating data. It allows practitioners to grasp cumulative effects without being influenced solely by extremes.
Visual Representations
Visual representations, including graphs and charts, provide clarity in understanding RMS calculations. By illustrating the relationship between time and value through graphical means, it becomes easier to recognize trends and patterns. For example, plotting RMS values against time in an alternating current circuit can highlight changes in power distribution.
Using such visuals increases engagement with the data, making it easier to grasp complex interactions. However, this approach can also lead to misrepresentation if scales are not consistent or accurately maintained. Therefore, ensuring proper scales and labeled axes is crucial for maintaining the integrity of visual data presentations.
"Incorporating visual representation into RMS analysis can drastically enhance comprehension and decision-making processes."
Combining both example problems and visual representations creates a comprehensive understanding of RMS. Utilizing multiple approaches leads to better retention and application of knowledge.
Applications of RMS
RMS, or Root Mean Square, is a concept deeply embedded in varied domains, from electrical engineering to signal processing and data analysis. Understanding its applications enhances appreciation for its capabilities and relevance across sectors. It provides insights into the measurement and analysis of diverse phenomena. This section delves into the practical applications of RMS, highlighting its importance in multiple fields, detailing specific aspects of its utility, and examining the benefits of using this approach.
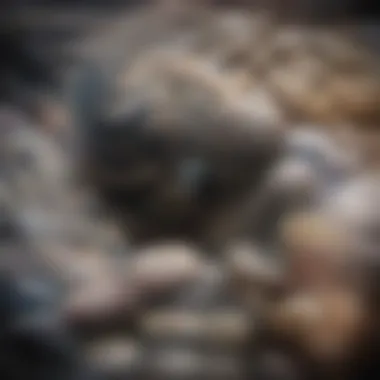
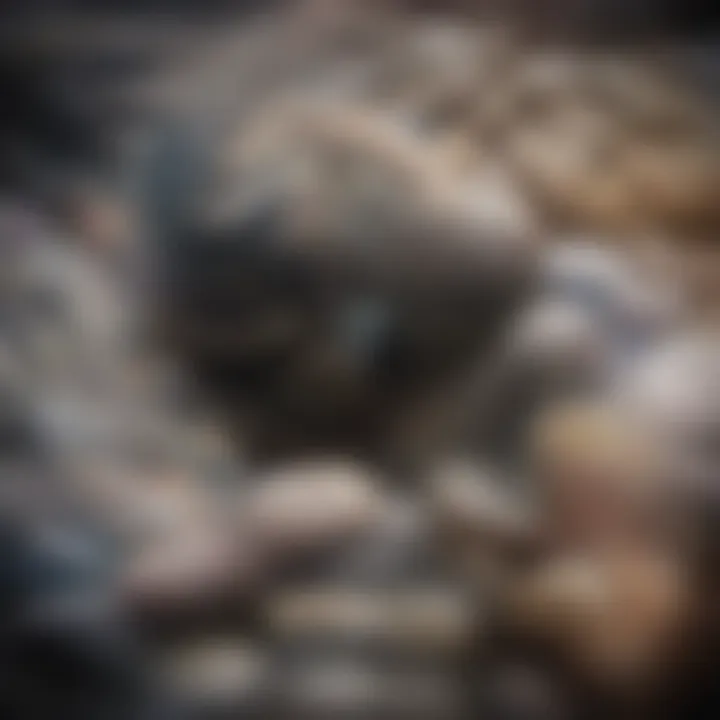
RMS in Electrical Engineering
AC Circuits
RMS plays a crucial role in AC circuits, which are fundamental in electrical engineering. The key characteristic of AC circuits is that they alternate direction and magnitude, which can complicate their analysis. RMS simplifies this by providing a measure that reflects the effective value of an AC current. This value is vital for designing and analyzing circuits since it allows engineers to compare AC and DC systems easily.
A unique feature of using RMS in AC circuits is its ability to convey average power delivered. By applying RMS, one can compute power with better accuracy and relevance. This characteristic makes it preferred for power calculations in electrical engineering. However, for those new to the concept, there can be a learning curve associated with understanding how RMS values relate to different aspects of circuit behavior.
Power Calculations
Power calculations are another area where RMS proves its worth. The primary aspect of power calculations is determining the energy consumed or delivered in a circuit efficiently. The RMS voltage and current values are key to accurate power measurement in AC systems. The standard formula involves using the RMS values in conjunction with the circuit's resistance or impedance.
The highlighted characteristic of RMS in power calculations is its accuracy. By using RMS values, engineers avoid the pitfalls of using average values, which can misrepresent power delivery in alternating current situations. However, it is essential to recognize that over-reliance on RMS without considering other circuit conditions may lead to misinterpretations in specific contexts.
RMS in Signal Processing
Noise Reduction
In signal processing, RMS is often employed in noise reduction techniques. Noise can significantly degrade signal quality, making this aspect vital in many applications, such as telecommunications. The specific aspect of noise reduction involves filtering out unwanted components from a signal while retaining essential information.
A prominent feature of RMS in noise reduction is its ability to quantify the average power of the accompanying signal. By focusing on RMS values, technicians can set thresholds for filtering out noise. This method is beneficial as it allows a clearer signal transmission. Nevertheless, it can also lead to scenarios where certain critical signal components may be unintentionally filtered out, so careful calibration and analysis are necessary.
Data Interpretation
Data interpretation in signal processing greatly benefits from using RMS values. The specific focus here is on comprehending the information within noisy data streams effectively. By utilizing RMS, analysts gain valuable insights into the underlying trends and behaviors that may not be evident from raw data alone.
The distinctive aspect of using RMS for data interpretation is its smoothing capability. It helps in revealing patterns that might be obscured by noise or volatility in the measurements. Nevertheless, it is crucial to indicate that relying solely on RMS can lead to oversimplifications. Understanding the data's context remains critical for accurate conclusions.
RMS in Data Analysis
Statistical Relevance
In data analysis, RMS has notable applications related to statistical relevance. It contributes fundamentally to how data sets are interpreted, especially in understanding variability and dispersion. The emphasis here lies on quantifying how much data points deviate from their mean value.
The critical feature of RMS in this context is its ability to provide a consistent measure of spread. This characteristic makes it a favorable choice for statisticians who require precision in their analysis. Despite these advantages, the interpretation of RMS values without considering other statistical measures may yield partial insights into data behavior.
Standard Deviation Comparison
Standard deviation comparison is a pivotal aspect of RMS's applications in data analysis. This discussion focuses on how RMS relates to the concept of standard deviation. In practice, the two metrics are closely linked, as both offer insight into data variability.
A uniqueness of RMS in this scenario is its superior capability to represent the magnitude of deviations, especially with non-linear distributions. This allows analysts to compare variances across different datasets effectively. However, it must be considered that RMS can sometimes lead to misinterpretations. Relying solely on it without understanding the underlying data structure may lead incorrect conclusions about data stability and reliability.
RMS is not just a calculation method; it is a versatile tool that enhances precision and understanding across various fields, particularly in electrical engineering and data analysis.
Advantages of Using RMS
The ReqIired Mean Square (RMS) is a valuable tool often used in various fields. Its significance lies in how it provides a reliable way to measure the average of a set of values, especially when dealing with varying elements. This section details the advantages of using RMS, focusing on its precision and ability to withstand anomalies in data. Understanding these benefits can enhance practical applications for students, researchers, and professionals alike.
Accuracy in Measurement
RMS offers a high degree of accuracy in quantifying values, particularly in the context of oscillating signals. Unlike simple averages, RMS takes into account the distribution of values in a dataset. This characteristic allows it to provide a more realistic representation of the overall magnitude. For instance, when analyzing alternating current in electrical engineering, using RMS values helps to ensure calculations reflect the true power delivered in a circuit.
The formula for RMS is:
[ RMS = \sqrt\frac1N \sum_i=1^N x_i^2 ]
This formula shows that each value is squared, which eliminates negative values and emphasizes larger deviations. This approach leads to more accurate readings, especially when assessing fluctuating values.
To illustrate, consider a dataset of sound wave frequencies. An average might suggest a moderate overall loudness, but RMS would identify the louder parts dominated the sound profile. Hence, in domains like signal processing, RMS remains a precise measurement metric critical for analysis.
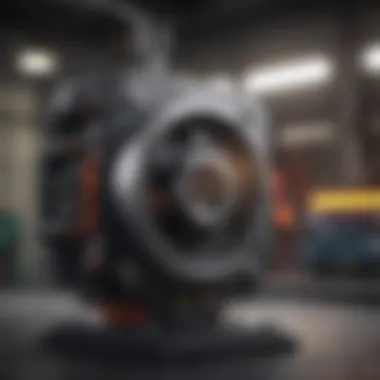
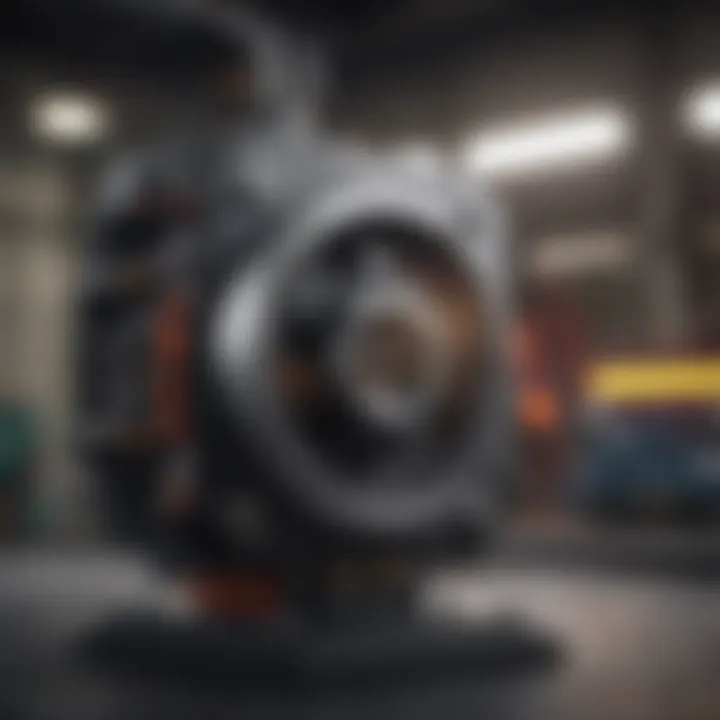
Robustness Against Outliers
Another advantage of RMS is its robustness against outliers. In many datasets, extreme values can skew averages, leading to misleading conclusions. However, RMS diminishes the impact of such anomalies through its squaring function. When calculating RMS, larger deviations, whether positive or negative, disproportionately influence the result due to their squared nature.
Consequently, this feature makes RMS particularly suited for scenarios where data may include unrepresentative extremes. For instance, in financial data analysis, outlier transactions can occur which do not reflect the general trend. By employing RMS, analysts can achieve a more reliable metric that accounts for everyday variations without the distortions introduced by outliers.
Challenges Associated with RMS
Understanding the challenges associated with RMS (Root Mean Square) is crucial as it informs users about the potential pitfalls of this important measurement metric. RMS, while highly useful in many fields, does have its limitations and risks of misinterpretation that need close examination. Recognizing these challenges can lead to better application and interpretation of RMS values across various disciplines.
Limitations in Application
One of the primary challenges tied to RMS is its limitations in application. RMS is typically used in contexts where there is a regular or predictable pattern, such as in alternating current (AC) circuits or signal processing. However, in situations with non-linear dynamics or systems that are highly unpredictable, RMS may not provide an accurate representation. Here are some specific limitations:
- Non-linear Systems: In non-linear applications, the relationship between variables may not be adequately captured by RMS. The method’s reliance on squaring values can overshadow important behaviors of the system.
- Transient Signals: In the case of transient signals, which are brief and can change rapidly, RMS may not give a true representation of the signal’s characteristics. Traditional RMS calculations assume a stable period, which transient phenomena lack.
- Assumption of Continuity: RMS assumes that data is continuous over its period of observation. When data points are missing or irregularly spaced, the RMS calculation can become unreliable.
These limitations demand awareness from practitioners who use RMS in their work. When using RMS, careful consideration of its assumptions and potential shortcomings is necessary for accurate measurement.
Misinterpretation Risks
Misinterpretation of RMS values is another significant challenge. RMS values can be misconstrued if the user does not fully understand the context in which they are applied. Here are several common areas where misunderstanding can arise:
- Confusion with Mean Values: Users often conflate RMS with simple averages or means, leading to inaccurate conclusions about data variability and trend. While RMS includes squaring the values, a mean does not account for this, potentially distorting perceptions of data.
- Ignoring Outliers: RMS can be unduly influenced by outliers, which may lead one to believe that the general trends in the data are more severe or pronounced than they really are. For instance, in data sets that contain extreme values, the RMS can tell a different story if one is not aware of these outliers.
- Contextual Coherence: In some disciplines, the context can greatly affect the interpretation of RMS values. For example, an RMS value in a signal processing context may not be directly comparable to the same RMS value evaluated in a different field, such as electrical engineering.
Misinterpretation of RMS can lead to erroneous conclusions and practices, affecting analysis adversely.
Awareness of these risks is essential for anyone involved in measurements that utilize RMS. Proper understanding and education on the correct usage of RMS values can greatly mitigate these issues and contribute to more informed decision-making.
Advanced Concepts Related to RMS
In understanding RMS, it is crucial to explore advanced concepts. This section delves into the relevance of RMS in non-linear systems and how it intersects with other measurement metrics. Recognizing these advanced concepts can show the versatility of RMS in various contexts.
RMS in Non-linear Systems
RMS applies effectively to non-linear systems. Non-linear systems do not follow the principle of superposition, making analysis complex. Hence, using RMS provides a way to measure fluctuations in outputs resulting from variable inputs. RMS helps in assessing the overall energy in signals that might not oscillate symmetrically about a mean value. With RMS, one can encapsulate the behavior of non-linear responses in a single value, enabling easier analysis and communication.
It is important to note that while RMS is effective in such systems, misapplication can lead to misleading results. For instance, using RMS over non-linear data without considering the context may obscure critical data variations. Therefore, caution is necessary when interpreting RMS values in non-linear scenarios.
RMS vs. Other Measurement Metrics
Measuring signals and data requires a careful choice of metrics. In this regard, RMS competes with several other measurement standards, two significant ones being Average and Mean Squared Error. Each has unique characteristics that affect their utility based on the application.
Average
The average, or arithmetic mean, originates from adding values and dividing by the number of values. This metric holds importance due to its simplicity and ability to represent a central value succinctly.
The key characteristic of the average is its straightforward calculation. This makes it a commonly used choice in basic statistics and everyday applications. Its benefits include ease of interpretation and the ability to handle a wide range of data types. However, one disadvantage is its susceptibility to outliers, which can skew results significantly.
The average presents a valuable context in understanding general trends but does not fully capture the variance present within the data. This limitation aligns with RMS's strength as it offers a different perspective that takes into account the magnitude of variations.
Mean Squared Error
Mean Squared Error (MSE) serves as another critical metric, especially in predictive modeling and signals processing. The MSE represents the average of the squares of the errors, quantifying how far predictions deviate from actual values.
A defining characteristic of MSE is its emphasis on larger errors, as squaring increases their influence in the overall calculation. This feature is beneficial when large errors are particularly undesirable in performance measures. However, MSE shares one weakness with the average; it is also sensitive to outliers, which may lead to misleading interpretations.
In summary, both average and mean squared error serve distinct purposes. While average provides a basic understanding, MSE reinforces RMS by addressing error magnitude in a rigorous way. Each metric contributes essential insights but knowing when to apply each can significantly impact outcomes.
Case Studies of RMS Applications
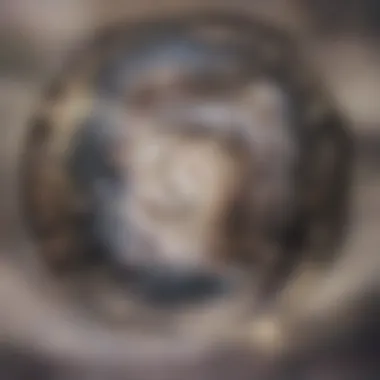
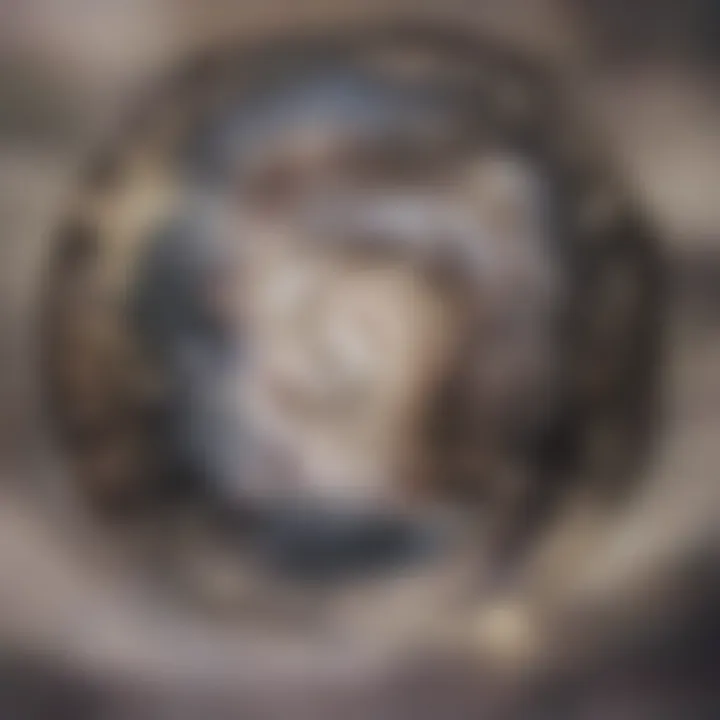
The application of Root Mean Square (RMS) can be better understood through specific case studies that showcase its relevance in real-world scenarios. These case studies not only illustrate the practical implications of RMS but also highlight its significance in various disciplines. By examining these instances, readers can gain insight into how RMS helps in measuring quality, ensuring accuracy, and ultimately influencing decisions in professional settings.
Case Study in Electrical Engineering
Electrical engineering often deals with alternating currents (AC), where the concept of RMS finds great utility. For instance, in the analysis of power distribution systems, measuring the voltage and current accurately is crucial. Suppose an electrical engineer is tasked with designing a circuit that resonates at a specific frequency. To achieve optimal performance, it’s essential to calculate the RMS values of the AC signals involved.
RMS provides a way to express the effective value of an AC signal, enabling engineers to determine how much actual power is transmitted through a circuit. In a practical example, consider a household appliance like a washing machine, which operates using an AC motor. To ensure efficiency and safety, engineers need to assess the RMS voltage and current specifications of the motor to guarantee that it operates within safe limits.
Some key points in this case study include:
- RMS Calculation in Power Systems: Determining the effective power consumption.
- Safety Measures: Establishing thresholds for voltage and current to prevent equipment damage.
- Performance Testing: Ensuring the appliance delivers required functions under varying load conditions.
This case study vividly shows RMS's role in electrical engineering. The accuracy in measurement contributes not only to the device's functionality but also to the safety of users, hence showcasing the critical nature of this computation.
Case Study in Signal Processing
In the realm of signal processing, RMS plays a fundamental role in analyzing and interpreting signals, particularly in communications and audio technology. For example, audio engineers often utilize RMS to determine the loudness of sound recordings. When working with various audio tracks, achieving a balanced sound level across all recordings is paramount.
By calculating the RMS value of each audio waveform, audio engineers can maintain consistent volume levels, ensuring that playback devices do not distort the sound. In an instance where an engineer is working with a series of spoken word recordings, the RMS values can assist in normalizing audio levels. This results in automatic adjustments where louder portions of sound do not overpower quieter segments, facilitating a more engaging listening experience.
Relevant elements in this case study include:
- Normalization of Audio: Achieving uniform loudness across multiple tracks.
- Denoising Techniques: Using RMS levels to filter out background noise effectively.
- Quality Analysis: Constructing an accurate representation of an audio signal’s loudness.
Through this case study, the utility of RMS in signal processing becomes clear. It serves not simply as a mathematical tool, but as an essential component that aids in enhancing audio quality and maintaining consumer satisfaction.
RMS calculations are vital across various fields, confirming its approach is multifaceted, blending mathematics with practical applications.
Future Directions in RMS Research
The exploration of future directions in RMS research is critical for both academic and professional communities. The rapid advancements in technology and the expansion of its applications underscore the need to continuously assess and evolve the use of RMS. Understanding emerging applications and technological innovations can provide insights into the potential growth areas and methodologies that may influence various sectors including engineering, data analysis, and signal processing.
Emerging Applications
As industries evolve, the adoption of RMS is extending into new and innovative fields. These emerging applications highlight the versatility of the RMS concept and its capacity to adapt to complex requirements. For example:
- Healthcare Monitoring: RMS can be used in analyzing physiological signals, improving the accuracy of patient monitoring systems. Integration with smart devices can enhance data interpretation, leading to timely medical interventions.
- Renewable Energy: In the domain of renewable energy, RMS measurements are crucial for evaluating waveform distortions in grid-connected systems. Enhanced RMS metrics can support better energy management, leading to improved efficiency.
- Artificial Intelligence: RMS is increasingly finding relevance in AI algorithms for processing sensory data. Using RMS metrics can help refine deep-learning models, making them more robust against environmental noise.
These applications illustrate the necessity of ongoing research into RMS methodologies to optimize performance across various fields. By developing new techniques tailored to specific challenges, researchers can unlock further potentials of RMS.
Technological Innovations
Technological innovations present unique opportunities for enhancing RMS methodologies. For example:
- Machine Learning Integration: The use of machine learning to process RMS data can lead to improved predictive analytics. Advanced algorithms can automate complex calculations, making real-time analysis feasible.
- Cloud Computing: The advent of cloud computing facilitates the handling of large datasets prevalent in RMS applications. This capability enhances data storage, sharing, and processing efficiency, allowing for collaborative research and cross-institutional studies.
- Wearable Technology: New devices that monitor various physical parameters are utilizing RMS to analyze data. This can advance personal health tracking and enhance athletic performance assessments.
These innovations contribute not only to the theoretical understanding of RMS but also promote practical applications that have real-world impact. Different sectors can greatly benefit from the intersection of RMS research and technological advancement, leading to shared knowledge and collaborative solutions.
"Innovations in technology can redefine how we understand and apply RMS across various fields, turning theoretical insights into actionable solutions."
These future directions in RMS research offer hope for exciting advancements. The potential for enhanced applications and technological progress underscores the importance of continued inquiry into RMS developments. Engaging with these elements ensures that scholars and practitioners remain at the forefront of an evolving landscape.
Closure
In this article, we have delved into the multifaceted concept of RMS (Root Mean Square) and its relevance across various fields, including engineering and data analysis. The conclusion serves not only as a recap of what we have discussed but also underscores the importance of understanding RMS in today’s context. Key elements include the mathematical theories backing it, its practical applications in real-world scenarios, and the advantages it brings to measurements and analysis.
Summary of Key Points
- Definition and Historical Context: RMS is defined as a statistical measure that reflects the magnitude of a varying quantity, especially applicable in electrical engineering and signal processing. Understanding its historical evolution provides insight into its significance.
- Mathematical Foundation: The derivation of the RMS formula showcases its analytical power, while calculation steps reinforce the methodology behind its applications.
- Diverse Applications: RMS plays a crucial role in electrical engineering through AC circuits and power calculations. Moreover, its utility in signal processing and data analysis highlights its versatility.
- Advantages and Challenges: While RMS is noted for its accuracy and robustness, one must also be mindful of its limitations in specific contexts and the potential for misinterpretation.
- Future Directions: Emerging applications and technological innovations suggest that RMS will continue to evolve, impacting research and methodology in various fields.
Final Thoughts on RMS
RMS stands as a critical tool in both theoretical and applied contexts. Its precision in measuring signals makes it invaluable in engineering disciplines, while its statistical relevance cannot be overstated in data analysis. As research advances, further exploration of RMS will likely unveil new applications and insights. Understanding RMS today not only enhances academic pursuits but also equips professionals with a vital skillset in their respective fields.
"Understanding RMS is crucial for anyone working with data and signals, offering clarity in measurement and analysis."
By committing to a thorough knowledge of RMS, individuals and organizations can improve decision-making and drive innovation in their work.